I. Introduction
Increasing ethanol production from cereal grains generates huge quantities of dried distillers’ grain with solubles (DDGS). As corn DDGS is rich in protein and energy it was commonly used for ruminants. In recent years, the high cost and supply shortage of common feed ingredients have prompted poultry producers to adopt DDGS in formulations. DDGS is known for its variation. During the past 10 years, we’ve seen evolutions in the ethanol industry and new technologies used in order to improve ethanol yield and profitability. These changes may not necessarily improve the quality and consistency of DDGS. Thus, it is important to determine the value and monitor the variation of DDGS of each batch, in a rapid yet reliable manner, for procurement and optimum usage in formulation. Classical evaluation on DDGS includes analyses of proximate nutrients by wet chemistry and colour density by visual check. This paper describes our latest Near Infrared Spectroscopy (NIRs) models developed for DDGS and the quality variation of 2433 DDGS samples uploaded by feed producers across the Asia Pacific region, for proximate composition and digestible nutrients.
II. Nir models for DDGS
We have collected 764 corn DDGS samples globally and analyzed for their proximate composition and total amino acids (TAA). We determined standardised ileal amino acid digestibility (SID AA) using in vivo model based on adult caecectomized cockerels, following the method described by Green at al. (1987). We also measured AME values of more than 130 corn DDGS samples through in vivo measurements using 3-week-old male broilers, following European reference method (Bourdillon et al., 1990) with ad libitum feeding and total excreta collection. All these corn DDGS samples were analyzed for their absorbances in the NIR region from 1100 to 2500 nm. Correlations between spectra characterizations and reference data were developed using partial least squares (PLS) regression technique. Performance of prediction models was reported as the standard error of calibration (SEC), standard error of cross validation (SECV) and calibration R2. The accuracy of the calibrations was validated with external samples and expressed by the standard error of prediction (SEP).
Table 1 shows the wet chemistry and in vivo results of these corn DDGS samples used for the calibrations and their performance statistics. The results indicate a wide range of sample set used for the calibration development. Crude protein of these 764 corn DDGS samples ranged from 152.0 to 434.0 g/kg, total lysine 7.52 g/kg (range 3.6 – 11.8 g/kg) with digestibility ranging from 45.2% to 80.6% (average = 55.67%). The values of R2 for CP, TAA and SID AA were higher than 0.77 (range 0.77 – 0.97), with ratio of standard deviation (SD) to standard error of calibration (SEC) close or above 3.
Table 1- Database description of NIRs calibration and statistics of corn DDGS
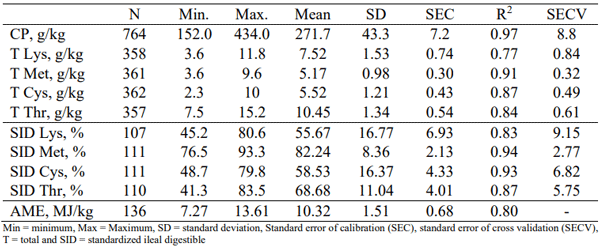
We have noticed other authors also reported AME prediction equations (Rochell et al., 2011, Jie et al., 2013, Meloche et al. 2013, Losada et al., 2015), based on limited batches of corn DDGS (usually from 6 - 30), and their residual standard deviation (RSD) ranged from 0.38 to 0.82 MJ/kg depending on the variables they reported. The best RSD 0.23 MJ/kg was from an equation developed by Losada et al. (2015), with 6 corn DDGS just derived from crude protein. In contrast, our in vivo based AME NIR calibrations included a calibration set ranging from 7.27 to 13.61 MJ/kg with average value 10.32 MJ/kg. High correlations were also noted for AME with R2 of 0.80. Considering the high variability and in particular the wide range of quality of our calibration set, our in vivo based AME NIRs model with 0.68 MJ/kg is appears to be more representative and reliable than previous models, as confirmed by external validation of 0.54 MJ/kg (SEP) using in vivo determination.
Thereafter, we integrated individual NIR database or equations into an online platform called Precise Nutrition Evaluation (PNE), which offers fast and robust tool for feed producers to obtain nutritional value, evaluate variability, track suppliers, and control formulation cost at their own convenience.
III. Corn DDGS used in southeast Asia
Following the above model development, from June 2020 to June 2021, our PNE platform received in total 2433 corn DDGS samples. All these samples were scanned on Adisseo standardized and validated NIR instruments at feed mill level. These samples were predicted for their proximate (PROX), namely dry matter (DM), crude protein (CP), ash (Ash), crude fiber (CF) and fats (Fat), TAA and SID AA, AME and AMEn.
Substantial variations were observed for all nutrition parameters, except for dry matter (Table 2). From the usual proximate parameters, the average corn DDGS contains: CP 295.4 g/kg, Ash 43.6 g/kg, Fat 83.2 g/kg, CF 74.2 g/kg. Coefficient of variation (CV) was 13.9% for Fat, 9.2% for Ash, 7.5% for CF and 5.8% for CP. Corn DDGS was rich in Leu but deficient in Trp. Essential TAA had CV ranging from 4.7 to 7.1%.
Table 2 – Contents of proximate compositions, total essential amino acids of corn DDGS
Standardized ileal digestibility amino acid (SID AA) CV ranged from 3.4% for SID Met and SID Leu to 12.6% for SID Cys. Mean value of AME for these corn DDGS samples was 9.81 MJ/kg with a minimum value 6.86 and maximum value 12.2 MJ/Kg. Nitrogen corrected AME (AMEn) was from 6.50 to 11.38 MJ/Kg with a standard deviation 0.79 MJ/kg. These results suggest considerable diversity among the corn DDGS sources which warrant a real time monitoring on the variability by screening every batch for its specific digestible nutrients. NIRs with in vivo based digestible nutrients database is a great tool used by feed mill for such monitoring.
Table 3. SID essential amino acids, AME and AMEn of corn DDGS
IV. Conclusion
Corn DDGS is a valuable ingredient for poultry diets but its variation has been a real concern. Our advanced NIR calibration models are based on wide sample representativeness, accurate wet chemistry data plus in vivo determinations, with prediction reliability confirmed by their performance statistics and external validation. This report summarized results of 2433 samples collected from Southeast Asia, showing the average crude protein 295.4 g/kg, crude ash 43.6 g/kg, crude fats 83.2 g/kg, and crude fibre 74.2 g/kg. Crude fats carried the highest variation (CV 13.9%), followed by crude ash (CV 9.2%). Corn DDGS was rich in Leu (34.5 g/kg) but deficient in Trp (2.4 g/kg). Essential TAA had CV from 4.7 to 7.1%. Mean AME value was 9.81 MJ/kg, with a considerable range from 6.86 to 12.2 MJ/Kg. Nitrogen corrected AME (AMEn) was from 6.50 to 11.38 MJ/Kg with a standard deviation 0.79 MJ/kg. These results revealed a considerable diversity among the corn DDGS sources used in Southeast Asia, and advanced NIR technology allows rapid and precise assessment, which enables informed procurement and the formulation by using “true” value to achieve expected performance results.
Presented at the 33th Annual Australian Poultry Science Symposium 2022. For information on the next edition, click here.