Introduction
Agricultural dust is generated in animal production facilities (Cambra-Lopez et al., 2010). Inhalation exposure to agricultural dust induces pulmonary inflammation and can lead to the development of chronic respiratory diseases (Palmberg et al., 1998; Redente and Massengale, 2006; Poole and Romberger, 2012). Several studies have demonstrated that agricultural workers, particularly poultry workers, have a reduction in lung function and higher prevalence of chronic respiratory diseases (e.g., chronic bronchitis) (Zuskin et al., 1995; Simpson et al., 1998; Radon et al., 2001; Rylander and Carvalheiro, 2006; Viegas et al., 2013). Furthermore, poultry dust induces pulmonary lesions and cardiac morphological changes in broiler chickens (Oyetunde et al., 1978; Riddell et al., 1998; Lai et al., 2009). Due to these human and animal health implications, it is critical to characterize the composition of poultry dust to determine the source and potential targets for engineering interventions.
Dust generated in poultry production consists of a complex mixture of chicken and human derived dander, bedding, chicken feed, and viable and nonviable microbial populations (Lenhart and Olenchock, 1984; Radon et al., 2002). Previous studies have used more specific molecular biology tools such as enzyme-linked immunosorbent assay and polymerase chain reaction to characterize poultry dust (Kwon et al., 2000; Oppliger et al., 2008; Prester and Macan, 2010; Just et al., 2011; Gerald et al., 2014). These studies have shown that poultry dust contains inflammatory agents such as lipopolysaccharide (LPS) and peptidoglycan (PGN), constituents of bacterial cell walls in Gram-negative and Gram-positive bacteria (Thedell et al., 1980; Sonesson et al., 1988; Gerald et al., 2014). Also, zoonotic viruses (e.g., avian influenza) have been detected in dust (Chen et al., 2010; Spekreijse et al., 2013). Settled agricultural dust extracts are predominantly employed to determine the mechanism underlying pulmonary toxicity (Palmberg et al., 1998; Redente and Massengale, 2006). Furthermore, it is unknown if there is a microbial difference between settled dust and aerosolized dust in large animal facilities.
The advancement of genomic sequencing technology has made the comprehensive analysis of microbes in poultry dust readily available. Previously, pyrosequencing was used to characterize bacteria and fungi targeting ribosomal RNAs (i.e., 16S and 18S), which are ubiquitously expressed in the bacteria and eukaryota domains (Nonnenmann et al., 2010; Boissy et al., 2014). In one sample of poultry dust, Staphylococcus cohnii and Sagenomella sclerotialis, respectively, were the most abundant bacterial and fungal species (Nonnenmann et al., 2010). Whole-genome shotgun pyrosequencing demonstrated that there are differing taxonomic profiles and genus abundance among swine, grain, and house dust (Boissy et al., 2014). Pyrosequencing technology generally has a high error rate, high cost per megabase, and lack of sufficient sequence coverage needed to assess complex whole genomic samples containing hundreds of low-abundance bacterial, viral and fungal species. Newer sequencing technology such as the Illumina HiSeq 2500 platform has several advantages over other sequencing technologies. Specifically, the Illumina HiSeq 2500 platform has lower error rates, better breadth and coverage depth, and lower cost per megabase than older technologies. Illumina HiSeq 2500 uses the sequencing by synthesis approach. Briefly, genomic DNA is fragmented into 300–600 base pair segments, and fragments are indexed using adapter sequences. The prepared library DNA is added to a flowcell and undergoes bridge amplification to form clonal clusters, where each cluster represents a single DNA fragment. Four different fluorescent dye-tagged nucleotides (dNTPs) are added to the reaction. Once the complementary dNTPs are incorporated into the DNA strand, the instrument detects the emission of light at each cluster simultaneously, which allows parallel sequencing of the library. Using paired-end sequencing, two reads are generated from the same DNA strand by sequencing from both the 50 and 30 end.
The aim of this study was to characterize the composition of microbial communities in airborne and settled poultry dust using a whole-genome shotgun sequencing approach and state-of-the-art analysis tools.
Results
Comprehensive analysis of microbial composition of airborne and settled poultry dust in broiler chicken houses Microbes account for the vast majority of the extracted DNA from both inhalable and settled poultry dust, yielding 95% of total reads (Fig. 1). DNA belonging to Homo sapiens (human) and Gallus gallus (broiler chicken) contributed less than 2% of reads in all poultry dust samples. Zea mays (maize) and Glycine max (soybean) DNA, components of chicken feed, were less than 1% of reads from settled and inhalable dust. Therefore, the vast majority of the DNA reads from each sample represent genuine microbial diversity present in the dust.
Metagenomic Phylogenetic Analysis 2 (MetaPhlan2) was used as the relative abundance analysis because it reports relative abundance on a community basis (i.e., the proportion of the total number of microbes in the sample belonging to a given species, genus, etc.) rather than on a read basis (Segata et al., 2012). This is advantageous because it yields percentages that have greater biological meaning and interpretability than simply the proportion of reads sequenced. MetaPhlan2 analysis showed that inhalable litter sampling (LS) dust, settled (S) dust and inhalable mortality collection (MC) dust had similar relative abundance at the domain level with the majority classified as bacteria (67%, 64% and 64% respectively). Viruses were the second most abundant domain in LS dust (25%), settled dust (29%) and MC dust (29%) (Fig. 2A). Although most viruses in the samples are attributed as ‘no-name’ or unclassified, there were significant contributions from the plant viral family Potyviridae and well characterized viral orders Caudovirales, Picornavirales, Tymovirales, Mononegavirales and Herpesvirales (data not shown).
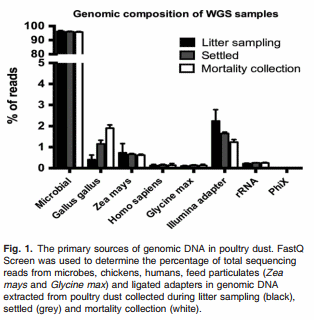
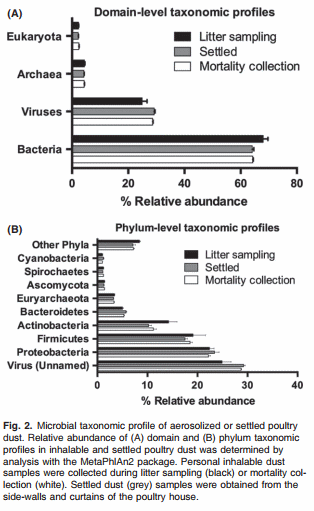
At the bacterial phylum level, Proteobacteria (33%, 37%, 35%), Firmicutes (28%, 27%, 28%) and Actinobacteria (19%, 15%, 16%) were the most abundant in LS dust, settled dust and MC dust respectively (Fig. 2B). The fungal phylum Ascomycota (1.4%, 1.3%, 1.4%) was a smaller relative abundance in poultry dust than bacterial phyla (Fig. 2B).
The heat map in Fig. 3 shows the 25 most abundant species in common among the poultry dust samples. The samples are clustered hierarchically using the BrayCurtis dissimilarity method. With the exception of three locations (LS13-15), the LS dust samples were one distinct cluster. The MC and settled dust samples formed a secondary cluster. Staphlyococcus sp AL1, Salinicoccus carnicancri and Lactobacillus crispatus had a high relative abundance in personal inhalable LS dust (LS1-15). In comparison, MC dust (MC1-3) had a reduction in L. crispatus but similar relative abundance of Staphylococcus sp AL1 and S. carnicancri. Bacterial genera detected in settled dust (S1-3) had a moderate relative abundance of Staphylococcus sp AL1, L. crispatus, S. carnicancri, Staphylococcus lentus and Lactobacillus salivarius. Settled dust had an elevated abundance of Enterococcus cecorum compared with LS and MC samples (Fig. 3). In addition, the viral species, Lactococcus phage 936 sensu lato, Carrot red leaf luteovirus, Mycobacterium phage Hamulus, Pseudomonas phage JG024, were present at low relative abundance among the poultry dust samples (Fig. 3).
Bacterial species composition is significantly different between aerosolized and settled dust
A statistical analysis of sample relative abundance was performed using the Linear discriminant analysis Effect Size (LEfSe) demonstrated ‘features’ (i.e., taxonomic relative abundances) of samples that may be used as a predictor of either LS, MC or settled dust (Fig. 4). The features shown in Fig. 4 are statistically different between dust types using a Kruskal–Wallis test (P < 0.05) and are subsequently found to have the largest effect score in a linear discriminant analysis model between the dust types (log LDA score > 3). Specifically, settled dust samples are characterized by an enrichment in the Enterococcus, Bacteroides, Vibrio and Clostridium genera. In contrast, LS dust is characterized by enrichment in the phylum Actinobacteria, the genus Brachybacterium and family Dermabacteraceae. Furthermore, MC dust is enriched in the Lachnospiricae family and the Porphyromonadaceae family. These taxonomic features could potentially provide reliable ‘biomarkers’ of LS, MC or settled dust in poultry houses. However, further studies are warranted.
Clustering samples on Bray-Curtis dissimilarity
The Bray-Curtis dissimilarity matrix, a statistical test used to quantify the compositional dissimilarity between different ecological sites, was calculated for all samples. Then, a principal component analysis (PCA) was performed to reduce the higher dimensional matrix to allow visualization of the amount of variation between sample profiles (Fig. 5). The LS and MC dust samples showed little variance among the groups, demonstrated by the formation of two distinct clusters. However, LS13-15 samples are outliers (which could be caused by either batch effects or genuine biological diversity). In contrast, settled dust samples show a greater variance among the three samples.
Discussion
In this study, next generation sequencing was used to make a comprehensive DNA analysis of poultry dust. Metagenomics analysis of inhalable poultry dust demonstrated that the Gram-positive bacteria species, Staphylococcus sp AL1, L. crispatus and S. carnicancri, are highly abundant in bioaerosols during LS and MC tasks (Fig. 3). Staphylococcus sp AL1, L. crispatus and Salinicoccus carncancri belong to the Firmicutes phylum, the second most abundant bacterial phylum in poultry dust (Fig. 2B). Staphylococcus sp AL1 was first isolated from Chinese soy sauce brine fermentation and encodes an N-acyl homoserine lactone (AHL) lactonase gene (Chong et al., 2012). AHL lactonases are quorumquenching enzymes that inhibits cell-to-cell communication of bacteria through the quorum sensing signalling of AHL (Dong and Zhang, 2005). L. crispatus has been found to colonize the epithelium of the chicken crop, mature enterocytes at the villus tip of the small intestine, and in the follicle-associated epithelium (FAE) and Peyer’s patches of the ileum (Edelman et al., 2002). In addition, L. crispatus strain ST1 and 134mi inhibited E. coli 789 adherence to the epithelium of the crop and ileal FAE (Edelman et al., 2003). S. carnicancri is a halophilic bacteria isolated from Korean ‘ganjanggejang’, raw crabs preserved in soy sauce, that prefers a salt rich environment of 5–20% (wt/vol) NaCl (Jung et al., 2010; Hyun et al., 2013). Lu et al. (2003) demonstrated that Salinococcus spp was present in broiler chicken litter collected from farms in Northeast Georgia using 16S rDNA sequencing. Furthermore, S. lentus, Salinicoccus spp., Lactobacillus spp., Corynebacterium ammoniagenes, Corynebacterium urealyticum, Brevibacterium sp. and Brachybacterium spp. were detected in broiler chicken dust and broiler chicken litter (Fig. 3) (Lu et al., 2003; Nonnenmann et al., 2010). Staphylococcus and E. coli were the only bacteria detected across studies using culture based and molecular techniques that corresponded to our findings (Brooks et al., 2010; Gerald et al., 2014). Clearly, the phenomenon of culture bias is limiting the reported compositional diversity of poultry dust in past studies. The results from our study suggest that poultry production facilities have a diverse and highly complex microbial ecosystem which varies substantially between airborne and settled dust.
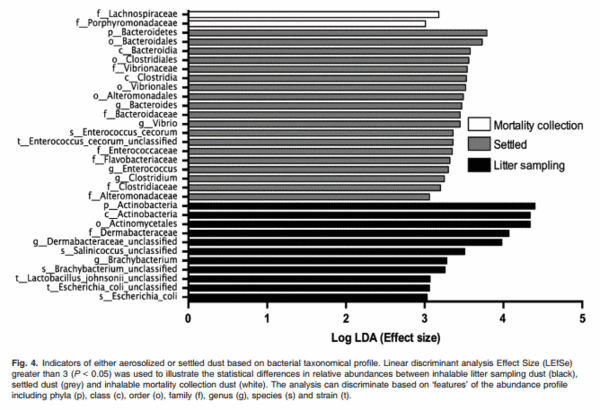
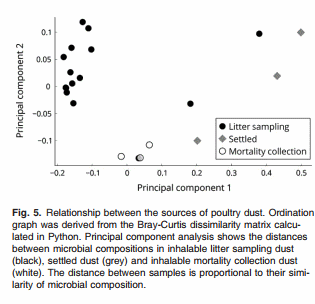
Both workers and broiler chickens suffer from exposure to dust generated during broiler chicken production. Poultry workers have a higher prevalence of acute and chronic respiratory diseases (e.g., occupational asthma and chronic bronchitis) than any other agricultural occupation, which has been attributed to the induction of the inflammatory response by endotoxin, a constituent of Gram-negative bacterial cell wall, in organic dust (Clark et al., 1983; Simpson et al., 1998; Donham et al., 2000; Malireddy et al., 2013; Viegas et al., 2013). For instance, inhalation of LPS, a marker of endotoxin, induced polymorphonuclear neutrophil and myeloperoxidase in the blood and sputum of healthy subjects 24 h post-exposure (Thorn and Rylander, 1998). In addition, endotoxin was shown to be a predictor of chronic phlegm in poultry workers (Kirychuk et al., 2006). For these reasons, an occupational exposure limit of 614 endotoxin units (EU) m3 has been recommended for poultry workers by (Donham et al. 2000). However, the inhibition of endotoxin activity in agricultural dust did not attenuate the inflammatory response in lung epithelial cells nor human peripheral blood monocytes (Romberger et al., 2002; Poole et al., 2010; Gottipati et al., 2015). Muramic acid, a marker of the bacterial cell wall component PGN and Gram-positive bacteria, has a higher concentration in large animal agricultural dust (i.e., swine and dairy) than human house dust (Poole et al., 2010). Our results further suggest that Gram-positive bacteria should be used for future mechanistic studies. Furthermore, the vast majority of studies elucidating the agricultural dust induced inflammatory mechanism in the respiratory tract have focused on aqueous or organic settled dust extracts (Poole et al., 2010; Gottipati et al., 2015). Our results demonstrate a significant difference of microbial diversity and abundance between airborne and settled poultry dust (Figs 3–5). These results suggest that future in vitro and in vivo toxicological studies should be performed to differentiate the immunomodulatory effects between bacteria in either airborne dust or settled dust. In addition, this study provides direction for selecting bacteria species as potential targets in future exposure characterization and respiratory toxicological studies.
There are potential limitations that may affect a study of this magnitude and scope. First, batch effects can occur when undertaking a study involving multiple sites spread throughout the Southeast region of the United States. Different environmental and housing conditions may impact the microbial populations present in broiler production in ways that are presently not well understood. For example, variation between samples may be partially due to differences in litter treatment and/or bedding, age of birds, and environmental conditions (e.g., temperature, relative humidity and poultry facility). Although whole-genome shotgun sequencing is an extremely powerful technique to characterize the ecological diversity of any environment, it cannot distinguish between viable and non-viable organisms in the sample. Therefore, the relative abundance of viable microorganisms in poultry dust needs to be further evaluated by specific cell culture assays. Despite these potential sources of variability, we note that there is a robust consistency in the taxonomic pro- files reported by the analysis that provides strong support for the general taxonomic profile of poultry production environments as reported. More studies will be needed to assess the role of environmental sources of variability on the taxonomic composition in poultry production environments
In conclusion, our findings demonstrate that poultry dust is predominately composed of bacteriophage viruses and Gram-positive bacteria with Staphylococcus sp. AL1, L. crispatus and S. carnicancri having the highest relative abundance among the airborne samples. In addition, these results show that the microbial composition of poultry dust varies between airborne and settled dust as well as working conditions. Our results provide an in-depth analysis of different types of poultry dust which can be used to elucidate mediators of the inflammatory response in poultry workers’ respiratory tract and provide guidance for the development of engineering controls designed for microorganisms present in broiler chicken production facilities.
Experimental procedures
Sample population
In this study, we collected either inhalable dust or settled dust samples on commercial broiler chicken farms in the southern region of the United States. Specifically, personal exposure of inhalable dust samples was collected among research staff performing LS (LS1-15) or MC (MC1-3) at 16 commercial broiler chicken farms (15 farms:LS and 1:MC). Litter sampling inhalable dust samples were collected among poultry farms during April and May. Seventy-three percent (11/15) of LS inhalable dust samples and 100% of MC inhalable dust samples had litter bedding treated with sodium bisulphate (PLT) (Table S1). Settled dust (S1-3) was collected into a 50 ml conical tube (Fischer Scientific, Pittsburgh, PA, USA) from curtains and side-walls of the chicken house on a university commercial broiler chicken farm during the month of March. This farm had litter bedding treated with liquid aluminium sulphate (Al+ Clear A7) (Table S1).
Sample collection
Two inhalable dust samplers were used for this study, Institute of Occupational Medicine (IOM; SKC, Eighty Four, PA, USA) or Button sampler (SKC). Each sampler contained a polyvinyl chloride filter with diameter of 25 mm and pore size 5.0 lm (SKC). The samplers were located in the personal breathing zone of a research personnel for times raging between 30 and 90 min depending upon the time needed to complete either LS or MC. The air samplers were connected to an AirChek XR5000 air pump (SKC) using an air flow of 2 (IOM) or 4 (Button) litres per minute depending upon the inhalable sampler used. Airflow was pre- and post-calibrated using a rotameter (VFB-65; Dwyer, Michigan City, IN, USA) calibrated to a primary airflow standard (Bios DryCal Defender 510; Mesa Labs, Butler, NJ, USA). After sampling, filters were stored in 50 ml polystyrene conical tube (Fisher Scientific) and stored at 20°C until further processing. Inhalable dust concentrations were determined gravimetrically.
Genomic DNA extraction
The inhalable dust and settled dust were allowed to equilibrate to room temperature. Genomic DNA was extracted from samples having a minimum of 0.500 mg of dust on the filters (Table S2). The samples were added to the tubes of a commercial DNA extraction kit, PowerSoil DNA Isolation Kit (MoBio Laboratories, Carlsbad, CA, USA). Genomic DNA was extracted per manufacturer’s instructions. Contaminants were removed using Agencourt Ampure XT Beads (Beckman Coulter, Pasadena, CA, USA). Genomic DNA was quantified using Quant-iT PicoGreen dsDNA assay kit (Life Technologies, Carlsbad, CA, USA). All dust samples had genomic DNA quantity above the required 1 ng for Illumina 2500 library preparation (Table S2).
Whole genome sequencing
Twenty-one dual-indexed libraries were prepared using Nextera XT DNA library prep kit (Illumina, San Diego, CA, USA) per manufacturers’ instructions. Libraries were combined into a single pool sequenced using a 125 bp paired-end run on a HiSeq 2500 instrument (Illumina) using version 4 chemistry located at the University of Minnesota Genomics Center (Minneapolis, MN, USA). Lane yields were greater than 220 M reads/lane. All expected barcodes were detected in the demultiplexing report and were well balanced. Library inserts averaged 500 bp. Sequence data used in this analysis have been deposited to the NCBI Sequence Read Archive (Study Accession SRP075218) (Table S3).
Sample genomic composition analysis
FastQ Screen (Babraham Bioinformatics; http:// www.bioinformatics.babraham.ac.uk) was used to identify the sequence composition of the raw data using the bowtie2 aligner (Langmead and Salzberg, 2012) with a 500 000 sequence subset and default parameters. Reference genomes for human (GRCh37), chicken (galGal4), corn (AGPv3), soybean (GM01) and phage PhiX (1993- 04-28) were downloaded from iGenomes (https://sup port.illumina.com/sequencing/sequencing_software/igeno me.html). The rRNA reference database was downloaded from green genes (http://greengenes.lbl.gov), and adapter sequences were collated from publicly available Illumina protocols for MiSeq and HiSeq experiments (Illumina). Although FastQ Screen identified non-microbial, non-adapter sequences present at low levels in the samples, these were not specifically filtered as the probability of aligning to the microbial clade-specific reference databases used for downstream analysis was negligible (Segata et al., 2012).
WGS read quality control
The FastQC tool (Babraham Bioinformatics; http:// www.bioinformatics.babraham.ac.uk) was used to assess sequence quality for Illumina paired-end dataset prior to downstream analysis. Data for each sample consisted of forward and reverse reads of length 126 nt and average GC content of 46.2%. Read quality was generally very high at all positions within the read. The introduction of sequencing errors towards the end of Illumina short reads can be a concern for accurate taxonomic classification (Camarinha-Silva et al., 2014). The marker gene approach applied in this work relies on many dozens of markers to identify each species, making the introduction of random sequencing errors near the end of reads less of a concern as compared with methods that rely on one region to assign taxonomic classification. Thus, we decided not to truncate reads unless quality scores were below a threshold value (described below). FastQC identified adapter contamination from the Nextera XT transposase kit (Illumina). Forward and reverse reads were left unpaired, but were concatenated together into a single FASTQ file for quality control processing and downstream analysis. Trimmomatic (Bolger et al., 2014) was used to remove adapter contamination from the reads as well as further improve the mean quality scores across all reads prior to taxonomic profiling. The software settings were ‘ILLUMINACLIP:./adapters.fa:2:30:10’ to remove the Nextera adapter sequences and ‘LEADING:3 TRAILING:3 SLIDINGWINDOW:4:20’ to improve mean sequence quality by trimming leading and lagging bases with Q < 3 and any sequences with a four-base sliding window mean below Q20.
Taxonomic abundance profiling of WGS reads
Microbial relative abundance profiles were generated with MetaPhlAn2 (Segata et al., 2012) using default parameters and bowtie2 alignment on a single 10-core node of an Intel(R) Xeon(R) E7-4850 (2.0 GHz) server with 120GB of physical (RAM) memory. A single lane of forward and reverse reads (~13 million read pairs) was provided as input for each sample to MetaPhlan2. The MetaPhlAn2 reference database consists of clade-specific marker genes (i.e., genes that unambiguously characterize a taxonomic clade as they are always present in the sequenced isolates of that clade and never present in any other sequenced organism) from ~17 000 reference genomes (79% bacteria/archea, 20.4% viral and 0.6% eukaryotic). Profiles for all samples were merged with the script ‘merge_metaphlan_tables.py’ included with the MetaPhlAn2 distribution and heatmaps were generated with ‘metaphlan_hclust_heatmap.py’ script using default options and the ‘-d braycurtis’, ‘-minv 0.01’, ‘-c bbcyr’ flags. Pie plots were generated with the script ‘metaphlan2krona.py’ and the KronaTools tool suite (Ondov et al., 2011).
Principal component analysis
Principal component analysis of the sample abundance profiles was carried out by first merging MetaPhlan2 results into a single BIOM format table. A Qiime (Caporaso et al., 2010) utility script for calculating the Bray-Curtis (Bray and Curtis, 1957) dissimilarity matrix on BIOMformatted taxonomic profiles was then used. The resulting dissimilarity matrix was imported into Python; calculation of PCA was performed with the scikit-learn ‘decomposition’ library (Pedregosa et al., 2011). The first principal component contained 65% of the variation and the second principal component contained 9% of the variation in the data. Graphical analysis was performed in Python.
Statistical biomarker analysis
A statistical analysis of differentially abundant features between classes of samples was accomplished with the LEfSe statistical analysis package. A table of species abundances for all samples was first prepared for analysis using the ‘format_input.py’ script with the ‘-o 1000000’ option to normalize all values to one million as recommended by the package authors. Three classes were defined as LS (15 samples), MC (3 samples) and S (3 samples). Running the analysis was accomplished with the ‘run_lefse.py’ script using a default P-value of 0.05 and the ‘-y l’ option to specify the ‘all-versus-all’ comparison for the initial class-level Kruskal–Wallis test prior to model building with LDA (Segata et al., 2011). The internal Wilcoxon test on subclasses was not performed because no subclasses were defined in the data. The cut-off value of three or greater for the log LDA effect size is arbitrary, selected to limit the features identified by the analysis to a reasonable number of the most significant features for plotting.
References
Boissy, R.J., Romberger, D.J., Roughead, W.A., Weissenburger-Moser, L., Poole, J.A., and LeVan, T.D. (2014) Shotgun pyrosequencing metagenomic analyses of dusts from swine confinement and grain facilities. PLoS ONE 9: e95578.
Bolger, A.M., Lohse, M., and Usadel, B. (2014) Trimmomatic: a flexible trimmer for Illumina sequence data. Bioinformatics 30: 2114–2120.
Bray, J.R., and Curtis, J.T. (1957) An ordination of the upland forest communities of southern wisconsin. Ecol Monogr 27: 325–349.
Brooks, J.P., McLaughlin, M.R., Scheffler, B., and Miles, D.M. (2010) Microbial and antibiotic resistant constituents associated with biological aerosols and poultry litter within a commercial poultry house. Sci Total Environ 408: 4770–4777.
Camarinha-Silva, A., Jauregui, R., Chaves-Moreno, D., Oxley, A.P., Schaumburg, F., Becker, K., et al. (2014) Comparing the anterior nare bacterial community of two discrete human populations using Illumina amplicon sequencing. Environ Microbiol 16: 2939–2952.
Cambra-Lopez, M., Aarnink, A.J.A., Zhao, Y., Calvet, S., and Torres, A.G. (2010) Airborne particulate matter from livestock production systems: a review of an air pollution problem. Environ Pollut 158: 1–17.
Caporaso, J.G., Kuczynski, J., Stombaugh, J., Bittinger, K., Bushman, F.D., Costello, E.K., et al. (2010) QIIME allows analysis of high-throughput community sequencing data. Nat Methods 7: 335–336.
Chen, P.S., Tsai, F.T., Lin, C.K., Yang, C.Y., Chan, C.C., Young, C.Y., and Lee, C.H. (2010) Ambient influenza and avian influenza virus during dust storm days and background days. Environ Health Perspect 118: 1211–1216.
Chong, T.M., Tung, H.J., Yin, W.F., and Chan, K.G. (2012) Insights from the genome sequence of quorum-quenching Staphylococcus sp. strain AL1, isolated from traditional Chinese soy sauce brine fermentation. J Bacteriol 194: 6611–6612.
Clark, S., Rylander, R., and Larsson, L. (1983) Airborne bacteria, endotoxin and fungi in dust in poultry and swine confinement buildings. Am Ind Hyg Assoc J 44: 537–541.
Dong, Y.H., and Zhang, L.H. (2005) Quorum sensing and quorum-quenching enzymes. J Microbiol 43: 101–109.
Donham, K.J., Cumro, D., Reynolds, S.J., and Merchant, J.A. (2000) Dose-response relationships between occupational aerosol exposures and cross-shift declines of lung function in poultry workers: recommendations for exposure limits. J Occup Environ Med 42: 260–269.
Edelman, S., Westerlund-Wikstrom, B., Leskela, S., Kettunen, H., Rautonen, N., Apajalahti, J., and Korhonen, T.K. (2002) In vitro adhesion specificity of indigenous Lactobacilli within the avian intestinal tract. Appl Environ Microbiol 68: 5155–5159.
Edelman, S., Leskela, S., Ron, E., Apajalahti, J., and Korho- € nen, T.K. (2003) In vitro adhesion of an avian pathogenic Escherichia coli O78 strain to surfaces of the chicken intestinal tract and to ileal mucus. Vet Microbiol 91: 41–56.
Gerald, C., McPherson, C., Xu, Z., Holmes, B., Williams, L., Whitley, N., and Waterman, J.T. (2014) A biophysiochemical analysis of settled livestock and poultry housing dusts. American Journal of Agricultural and Biological Sciences 9: 153.
Gottipati, K.R., Bandari, S.K., Nonnenmann, M.W., Levin, J.L., Dooley, G.P., Reynolds, S.J., and Boggaram, V. (2015) Transcriptional mechanisms and protein kinase signaling mediate organic dust induction of IL-8 expression in lung epithelial and THP-1 cells. Am J Physiol Lung C 308: L11–L21.
Hyun, D.W., Whon, T.W., Cho, Y.J., Chun, J., Kim, M.S., Jung, M.J., et al. (2013) Genome sequence of the moderately halophilic bacterium Salinicoccus carnicancri type strain Crm(T) (= DSM 23852(T)). Stand Genomic Sci 8: 255–263.
Jung, M.J., Kim, M.S., Roh, S.W., Shin, K.S., and Bae, J.W. (2010) Salinicoccus carnicancri sp. nov., a halophilic bacterium isolated from a Korean fermented seafood. Int J Syst Evol Microbiol 60: 653–658.
Just, N., Kirychuk, S., Gilbert, Y., Letourneau, V., Veillette, M., Singh, B., and Duchaine, C. (2011) Bacterial diversity characterization of bioaerosols from cage-housed and floorhoused poultry operations. Environ Res 111: 492–498.
Kirychuk, S.P., Dosman, J.A., Reynolds, S.J., Willson, P., Senthilselvan, A., Feddes, J.J., et al. (2006) Total dust and endotoxin in poultry operations: comparison between cage and floor housing and respiratory effects in workers. J Occup Environ Med 48: 741–748.
Kwon, Y.M., Woodward, C.L., Pillai, S.D., Pena, J., Corrier, ~ D.E., Byrd, J.A., and Ricke, S.C. (2000) Litter and aerosol sampling of chicken houses for rapid detection of Salmonella typhimurium contamination using gene amplification. J Ind Microbiol Biot 24: 379–382.
Lai, H.T., Nieuwland, M.G., Kemp, B., Aarnink, A.J., and Parmentier, H.K. (2009) Effects of dust and airborne dust components on antibody responses, body weight gain, and heart morphology of broilers. Poult Sci 88: 1838–1849.
Langmead, B., and Salzberg, S.L. (2012) Fast gapped-read alignment with Bowtie 2. Nat Meth 9: 357–359.
Lenhart, S.W., and Olenchock, S.A. (1984) Sources of respiratory insult in the poultry processing industry. Am J Ind Med 6: 89–96.
Lu, J., Sanchez, S., Hofacre, C., Maurer, J.J., Harmon, B.G., and Lee, M.D. (2003) Evaluation of broiler litter with reference to the microbial composition as assessed by using 16S rRNA and functional gene markers. Appl Environ Microbiol 69: 901–908.
Malireddy, S., Lawson, C., Steinhour, E., Hart, J., Kotha, S.R., Patel, R.B., et al. (2013) Airborne agricultural particulate matter induces inflammatory cytokine secretion by respiratory epithelial cells: mechanisms of regulation by eicosanoid lipid signal mediators. Indian J Biochem Biophys 50: 387–401.
Nonnenmann, M.W., Bextine, B., Dowd, S.E., Gilmore, K., and Levin, J.L. (2010) Culture-independent characterization of bacteria and fungi in a poultry bioaerosol using pyrosequencing: a new approach. J Occup Environ Hyg 7: 693–699.
Ondov, B.D., Bergman, N.H., and Phillippy, A.M. (2011) Interactive metagenomic visualization in a Web browser. BMC Bioinformatics 12: 385.
Oppliger, A., Charriere, N., Droz, P.O., and Rinsoz, T. (2008) Exposure to bioaerosols in poultry houses at different stages of fattening; use of real-time PCR for airborne bacterial quantification. Ann Occup Hyg 52: 405–412.
Oyetunde, O.O., Thomson, R.G., and Carlson, H.C. (1978) Aerosol exposure of ammonia, dust and Escherichia coli in broiler chickens. Can Vet J 19: 187–193.
Palmberg, L., Larsson, B.M., Malmberg, P., and Larsson, K. (1998) Induction of IL-8 production in human alveolar macrophages and human bronchial epithelial cells in vitro by swine dust. Thorax 53: 260–264.
Pedregosa, F., Varoquaux, G., Gramfort, A., Michel, V., Thirion, B., Grisel, O., et al. (2011) Scikit-learn: machine learning in Python. J Mach Learn Res 12: 2825–2830.
Poole, J.A., and Romberger, D.J. (2012) Immunological and inflammatory responses to organic dust in agriculture. Curr Opin Allergy Clin Immunol 12: 126–132.
Poole, J.A., Dooley, G.P., Saito, R., Burrell, A.M., Bailey, K.L., Romberger, D.J., et al. (2010) Muramic acid, endotoxin, 3-hydroxy fatty acids, and ergosterol content explain monocyte and epithelial cell inflammatory responses to agricultural dusts. J Toxicol Environ Health A 73: 684–700.
Prester, L., and Macan, J. (2010) Determination of Alt a 1 (Alternaria alternata) in poultry farms and a sawmill using ELISA. Med Mycol 48: 298–302.
Radon, K., Weber, C., Iversen, M., Danuser, B., Pedersen, S., and Nowak, D. (2001) Exposure assessment and lung function in pig and poultry farmers. Occup Environ Med 58: 405–410.
Radon, K., Danuser, B., Iversen, M., Monso, E., Weber, C., Hartung, J., et al. (2002) Air contaminants in different European farming environments. Ann Agric Environ Med 9: 41–48.
Redente, E.F., and Massengale, R.D. (2006) A systematic analysis of the effect of corn, wheat, and poultry dusts on interleukin-8 production by human respiratory epithelial cells. J Immunotoxicol 3: 31–37.
Riddell, C., Schwean, K., and Classen, H.L. (1998) Inflammation of the bronchi in broiler chickens, associated with barn dust and the influence of barn temperature. Avian Dis 42: 225–229.
Romberger, D.J., Bodlak, V., Von Essen, S.G., Mathisen, T., and Wyatt, T.A. (2002) Hog barn dust extract stimulates IL- 8 and IL-6 release in human bronchial epithelial cells via PKC activation. J Appl Physiol (1985) 93: 289–296.
Rylander, R., and Carvalheiro, M.F. (2006) Airways inflammation among workers in poultry houses. Int Arch Occup Environ Health 79: 487–490.
Segata, N., Izard, J., Waldron, L., Gevers, D., Miropolsky, L., Garrett, W.S., and Huttenhower, C. (2011) Metagenomic biomarker discovery and explanation. Genome Biol 12: R60. Segata, N., Waldron, L., Ballarini, A., Narasimhan, V., Jousson, O., and Huttenhower, C. (2012) Metagenomic microbial community profiling using unique clade-specific marker genes. Nat Methods 9: 811–814.
Simpson, J.C., Niven, R.M., Pickering, C.A., Fletcher, A.M., Oldham, L.A., and Francis, H.M. (1998) Prevalence and predictors of work related respiratory symptoms in workers exposed to organic dusts. Occup Environ Med 55: 668–672.
Sonesson, A., Larsson, L., Fox, A., Westerdahl, G., and Odham, G. (1988) Determination of environmental levels of peptidoglycan and lipopolysaccharide using gas chromatography with negative-ion chemical-ionization mass spectrometry utilizing bacterial amino acids and hydroxy fatty acids as biomarkers. J Chromatogr 431: 1–15.
Spekreijse, D., Bouma, A., Koch, G., and Stegeman, A. (2013) Quantification of dust-borne transmission of highly pathogenic avian influenza virus between chickens. Influenza Other Respir Viruses 7: 132–138.
Thedell, T.D., Mull, J.C., and Olenchock, S.A. (1980) A brief report of Gram-negative bacterial endotoxin levels in airborne and settled dusts in animal confinement buildings. Am J Ind Med 1: 3–7.
Thorn, J., and Rylander, R. (1998) Inflammatory response after inhalation of bacterial endotoxin assessed by the induced sputum technique. Thorax 53: 1047–1052.
Viegas, S., Faisca, V.M., Dias, H., Clerigo, A., Carolino, E., and Viegas, C. (2013) Occupational exposure to poultry dust and effects on the respiratory system in workers. J Toxicol Environ Health A 76: 230–239.
Zuskin, E., Mustajbegovic, J., Schachter, E.N., Kern, J., Rienzi, N., Goswami, S., et al. (1995) Respiratory function in poultry workers and pharmacologic characterization of poultry dust extract. Environ Res 70: 11–19.