Take home messages
- Application of high-throughput technologies (e.g. microarrays, next-generation sequencing) in a systems biology framework to study the interactions of physiological state and nutrition on tissue function in livestock species is a powerful means to gain a holistic view of the underlying biological phenomena.
- Potential practical applications from applying such an approach in studies of mammary, adipose, and liver of dairy cows are emerging. Examples include manipulating the level of dietary energy prepartum as well as supplementation with lipids or amino acids.
- Application of novel bioinformatics approaches to transcriptomics data from studies with peripartal cows managed to consume different levels of energy or fed different sources of dietary lipid during the dry period uncovered functional gene networks in both adipose and liver that could be targeted via nutrition.
- Our in vitro results uncovered a strong effect of saturated long-chain fatty acids, particularly palmitate, on the PPAR network.
- Application of knowledge generated from functional genomics in livestock would have to be tested in the future and should encompass measures of disease incidence, fertility, and longevity.
Introduction
Application of high-throughput sequencing and transcriptomics technologies has dramatically accelerated the rate at which biological and genetic information can be generated. Together with whole-animal level information (e.g., blood metabolites/hormones, tissue chemical composition, performance), large-scale DNA and mRNA information have increased our understanding of mechanisms of metabolic regulation in agricultural species (e.g., Hocquette et al., 2009; Loor, 2010). Through the use of bioinformatics, clustering, and gene network analysis, transcriptomics is allowing the identification of regulatory mechanisms that are associated with functional development of tissues of agricultural animals. Continued application of genome-enabled (genotyping, microarrays, proteomics, gene silencing) and allied (e.g., phosphoproteomics) technologies will contribute to our understanding of animal biological adaptations to physiological phases or environmental cues. For instance, the use of those tools can allow uncovering regulatory points at different stages of lactation as well during muscle and fat growth. This information will provide new insights into opportunities for enhancing efficiency of animal production, e.g. ways to use gene-level data for developing practical nutritional (or general management) strategies for feeding dairy or beef cattle to achieve a desired outcome. Such outcomes could be health-related, e.g., mitigate metabolic/infectious disease in the transition period with cows, or animal product-related, e.g., produce better quality meat.
Dairy cows represent both an economically-important livestock species and a unique biological model of mammalian adaptations. The latter feature is partly due to the unrivalled requirement for dietary energy (Allen et al., 2005). Extensive microbial fermentation in the rumen results in a constant reliance on gluconeogenesis, a process that increases dramatically immediately after parturition. The transition from pregnancy into lactation in dairy cattle is characterized by metabolic adaptations in major organs (e.g. mammary, liver, adipose) that allow the animal to adjust to the need of synthesizing large amounts of milk. Different physiological stages during growth or the lactation cycle provide powerful models to study how long-term signals interact to affect long-term tissue and whole-animal function.
The peripartal dairy cow as a model for whole-animal systems biology
The nature of the physiologic and metabolic adaptations during the transition from pregnancy to lactation is multifaceted and involves interconnections between key tissues and cell types, further underscoring the need for a systems biology approach to identify control points which go beyond the classical metabolic pathways. We have recently proposed (Loor, 2010) that in order to address the complex metabolic phenotypes of the peripartal period there is a need to identify at the very least transcript variations in liver, mammary, and adipose that might underlie variation in metabolism and health. The approach could initially encompass a transcriptomic characterization of each tissue as well as immune cells (e.g., neutrophils) over a wider range of nutritional treatments of practical relevance, and also across cows of different genetic merit (Loor, 2010). Within individual experiments, the data generated would allow for the identification of underlying gene networks and networks within pathways and functions that can be linked to a particular metabolic or health phenotype.
The concept of gene networks: a framework for practical applications of nutrition
Extensive work in non-ruminants has provided concrete evidence that metabolic regulation relies partly on transcriptional control as a long-term mechanism affecting the level of expression of several enzymes in a biochemical pathway (e.g., Desvergne et al., 2006). At the transcriptional level, enzymes within classical biochemical pathways can be viewed as a gene network or a collection of DNA segments which can interact either with a regulator such as a transcription factor (TF) or nuclear receptor (NR), but also with each other through their RNA and protein products and with other molecules in the cell (Wittkopp, 2007). These "global" interactions, thus, can govern the rates at which genes in the network are transcribed into mRNA and eventually into proteins which can catalyze a metabolic reaction. Three classical examples of TF and NR controlling lipogenesis and adipogenesis at the transcriptional level are sterol regulatory element binding factor 1 (SREBF1), MLX interacting protein-like (MLXIPL; formerly known as carbohydrate-responsive element binding protein or ChREBP), and the ligand-activated nuclear receptor PPAR? (PPARG), respectively (Fernyhough et al., 2007).
The family of PPAR are clear examples of nutrient-regulated NR which can determine the expression and ultimately function of a particular tissue (e.g. adipose, mammary) at a given physiological state (e.g. early lactation, dry period). Long-chain fatty acids (LCFA) are well-established activators of PPAR in non-ruminants and some of our work has provided evidence that the same is the case in ruminant cells (Kadegowda et al., 2009; Thering et al., 2009; Bionaz et al., 2011). We have proposed recently (Bionaz and Loor, 2008; Graugnard et al., 2009, 2010) that temporal examination of most if not all the known components of metabolic networks is a suitable means of addressing the issue of transcriptional regulation as it pertains to tissue metabolism in response to growth and/or nutritional management. Because of the complexity and interconnectivity, i.e., the different levels of aggregation from gene-to-protein-to-metabolite-to-function, of metabolic networks in mammals there is a need for implementing systems-level computational approaches that link (at the very least) transcript, metabolite, and phenotype relationships. These approaches will be effective for 1) the discovery of missing information, 2) the formulation of in silico models, and 3) as a structured context for analyzing high-throughput aggregated biological data sets (Palsson, 2010). Integrating transcriptomics data into existing models of metabolism and nutrient use in cattle can go a long way to help understand the critical genetic/environmental interactions in the growing and/or lactating animal (e.g., McNamara, 2010).
What is bioinformatics and how can we use it in ruminant nutrition/physiology ?
Bioinformatics involves the use of techniques including mathematics, statistics, and biochemistry to solve biological problems at the molecular level (Mo and Palsson, 2009). The core principle of these techniques is utilizing computer resources in order to solve problems on scales of magnitude far too great for human discernment. A bioinformatics approach will allow discerning the biological functions in tissues at a specific point of development or under a particular nutritional management, e.g. due to lipid or amino acid supplementation, level of dietary forage to concentrate. Biological functions uncovered are easily mined through the use of gene ontology (GO) analysis, which classifies genes into a molecular function, cellular component, or biological process based on published experimental evidence. One of the aims of the GO Consortium is to provide a controlled vocabulary that can be used to describe any organism. However, it is intuitive that many functions, processes, and components are not common to all life forms. Annotation with respect to the biological context of livestock would be an important undertaking. A recent paper provides an in-depth overview of the development of an animal trait ontology, which is essential for annotating genes/proteins to biological functions (Hughes et al., 2008).
Bioinformatics to study adipose and liver responses to nutritional management
In order to uncover functional gene networks that could be targeted via nutrition, we have applied a novel bioinformatics approach (DIA) together with ORA and network analysis to transcriptomics data from several studies including liver and adipose from peripartal cows managed to consume different levels of energy or fed different sources of dietary lipid during the dry period (Loor et al., 2011). For the study of adipose tissue's adaptations to level of energy prepartum, cows were fed 150% of energy requirement or a control diet (i.e., 100% requirement) for ca. 40 days prior to parturition, with cows fed the same diet (to meet ~100% of requirements) after parturition. Subcutaneous adipose tissue was collected before starting the dietary treatments and at several time points until ca. one month postpartum. Results clearly highlighted a strong and acute effect of overfeeding energy prepartum on the adipose transcriptome. The functional analysis of the differentially expressed genes between the energy-overfed vs. control cows uncovered enhanced lipid synthesis and energy utilization in overfed cows, with a central role of PPAR (peroxisome proliferator-activated receptors) signaling in coordinating expression of genes within those pathways. Network analysis of genes involved in lipid synthesis and energy utilization also suggested a central role of insulin in coordinating such adaptations. Interestingly, after parturition when all cows were fed the same diet, the differences in the transcriptome between groups virtually disappeared. This suggested that the transcriptome in adipose tissue adapts very rapidly to level of dietary energy. The re-wiring of the adipose transcriptome appeared to be quite strong, as suggested by the minimal carryover effect observed during the first month post-partum.
Previously-published transcriptomics data from liver of transition cows fed different level of energy (~80%, 100%, or >140% energy requirement) from dry-off to parturition (Loor et al., 2005, 2006) were re-analysed using a combination of k-means clustering analysis with the ORA approach (Loor et al., 2011) and also the DIA approach (Shahzad et al., 2011). The analysis uncovered 13 clusters with the highest predictive pattern of expression among the 4,790 differentially expressed genes (at an FDR ≤ 0.05 diet × time effect). It uncovered genes whose expression needs to be co-ordinately regulated in liver in response to energetic cues. We observed a remarkably similar pattern of genes within several clusters between the high and low energy diet, which highlighted the existence of "stress response" in the animal to the sub-optimal dietary conditions.
In contrast with the adipose tissue responses discussed above, the level of dietary energy fed pre-partum appeared to have carryover effects on the liver transcriptome. There were few clusters of genes with evident differences in temporal patterns of expression between the prepartal dietary energy levels. In addition, few pathways or functions were significantly enriched (FDR ≤ 0.05) within the gene clusters. Among those we observed a co-ordinated increase in expression of many genes involved in protein synthesis and a co-ordinated decrease in expression of genes involved in complement system-immune response in either energy restricted or overfed cows. Data also uncovered a synchronized increase in energy-restricted cows of genes coding for mitochondrial components.
The use of DIA to evaluate the dynamics of transcriptomics adaptations to energy level prepartum uncovered a large impact of dietary energy on biosynthesis of secondary metabolites, protein synthesis, energy metabolism, and cellular signaling and interactions. The detailed analysis of main pathways within those categories highlighted an increase in glucose metabolism and pentose phosphate pathway with both overfeeding and restricting energy intake prepartum. Cows fed restricted energy prepartum had a greater activation of oxidative phosphorylation and the TCA cycle, while liver of energy-overfed cows had greater synthesis of ketone bodies and steroids. Cell cycle was more induced in energy-overfed cows and antigen presentation in energy-restricted cows. The latter group also had a greater induction of PPAR signaling. Overall, data clearly support a strong effect of prepartal dietary energy on the dairy cow liver transcriptome with an apparent large degree of remodelling and metabolic adaptation of the tissue. In the context of the latter, the energy-restriction appeared to have induced a greater degree of activation of PPAR signalling.
The importance of PPAR in coordinating liver adaptations to energy level in the diet has also been previously observed by our group in cows induced to develop ketosis by feed restriction after parturition (Loor et al., 2007). Overall, results from the different studies outlined above point to a pivotal role of PPAR in both liver and adipose adaptations to dietary energy in dairy cows. A role for PPAR also has been observed in mammary gland of dairy cows during lactation with a potential role in coordinating expression of genes involved in milk fat synthesis (Bionaz and Loor, 2008; Kadegowda et al., 2009) . Those results have revealed the interesting possibility for potential practical uses of dietary lipid supplementation during the peripartal period in dairy cows because of the well-known response of PPAR to long-chain fatty acids (LCFA) in monogastrics. We have provided preliminary data demonstrating that PPAR in ruminants also responds to LCFA, particularly saturated LCFA, or PPAR agonists both in vitro (Bionaz et al., 2008; Thering et al., 2009; Bionaz et al., 2011) and in vivo (Litherland et al., 2010). These functional responses must be evaluated further under research and field conditions with particular emphasis on disease incidence, reproduction, and longevity.
Nutritional management and skeletal muscle transcriptomics
Early weaning and nutrition
Weaning calves earlier than the traditional ~205 d age, in particular, is a practical management tool that can be used to enhance feed efficiency as well as produce high-quality beef (e.g., Schoonmaker et al., 2002). For example, weaning at ~150 d compared with traditional weaning resulted in 30% more calves grading average choice or above and also improved feed efficiency in the feedlot (Myers et al., 1999). Very few genes known to be associated with adipogenesis are upregulated in skeletal muscle (i.e., longissimus lumborum) of newborn WH compared with PH calves (Lehnert et al., 2007). Those observations coupled with the findings that 11 month JB vs. H cattle (Wang et al., 2005) had greater expression of genes associated with lipogenesis and adipogenesis suggested that the onset of marbling in animals occurs between birth and the early postweaning phase (Wang et al., 2009). However, the exact timing and development of marbling in cattle are still unknown. It is evident from the above studies that there is a time-frame during which early nutritional intervention could be used as a practical means to initiate precocious intramuscular fat development and, potentially, increase the likelihood of achieving greater marbling at slaughter (Graugnard et al., 2009, 2010). Once identified, these putative regulators of adipogenesis could potentially be used as markers of marbling in breeding programs.
Effects of early weaning and level of dietary starch on longissimus lumborum transcript profiles
Our research group (Graugnard et al., 2007) recently utilized purebred early-weaned (155 d age) Angus steers to examine skeletal muscle transcriptomics in response to feeding a high-starch (5980 kJ/kg dry matter) or low-starch (4970 kJ net energy for gain/kg) diet for 112 d (growing phase) followed by a common high-starch diet (6030 kJ/kg) for an additional 112 d (finishing phase). Longissimus lumborum was biopsied at 0, 56, 112, and 224 d of the start of feeding diets. Tissue RNA was subjected to microarray analysis using the 13,000 gene bovine microarray developed at the University of Illinois. Most of the gene expression changes in our study (FDR-adjusted P < 0.01) were due to time, i.e., physiological state or growth. However, comparisons of differentially expressed (DEG) between specific time points revealed that most of the changes during growth were observed during the first 56 d of the growing phase (1,471 DEG) and at the mid-point (224 d; 3,200-2,700 DEG) of the finishing phase relative to other points of the growing phase. It was apparent from these data that transcriptional adaptations in skeletal muscle of these early-weaned animals were "complete" during the first half of the growing phase despite the fact they consumed incremental amounts of feed (~6 kg/d to ~10 kg/d by 112 d) and gained body weight (~1.65 kg/d) through the end of the growing phase (i.e., 112 d on treatments). A second and more robust bout of transcriptional adaptations occurred after all animals consumed the same high-starch/high-energy finishing diet for ~112 d. During that time-frame, there were no differences in dry matter intake (~10 kg/d), estimated energy intake, or feed efficiency (0.15 kg average daily gain/dry matter intake) (Graugnard et al., 2009).
Adipogenic gene networks during growth
Effects of early weaning and level of dietary starch
Most current emphasis (e.g., Wang et al., 2005, 2009; Smith et al., 2009) on adipose metabolism has been on examination of selected components of adipogenic and lipogenic gene networks, e.g., SCD, PPARG, FASN, and acetyl-CoA carboxylase-α (ACACA) among others. Recent work from our group has used a network approach to study temporal adaptations of adipogenic networks in early-weaned Angus or Angus × Simmental steers (Graugnard et al., 2009, 2010). Exposure to high-starch diets during the early growing phase appeared to induce precocious pre-adipocyte differentiation and lipid filling through upregulation of PPARG and its target genes (SCD, diacylglycerol O-acyltransferase homolog 2 (mouse) (DGAT2), and FABP4). High-starch also led to greater SREBF1 and MLXIPL during the finishing phase, which coupled with greater glucose-6-phosphate dehydrogenase (G6PD) and ADIPOQ could help sustain/promote adipogenesis (Graugnard et al., 2010). The low-starch/high-fibre diet apparently delayed adipocyte differentiation as indicated by increased THRSP, FASN, FABP4, SCD, and DGAT2 during the second half of the growing phase. Quite surprisingly, animals fed low-starch/high-fibre during the growing phase had additional increases in expression of THRSP, PPARG, FABP4, acyl-CoA synthetase medium-chain family member 1 (ACSM1), SCD, and DGAT2 during the finishing phase. However, the concomitant increase in nuclear receptor subfamily 2, group F, member 2 (NR2F2) expression (a repressor of adipocyte differentiation in rodents; Okamura et al., 2009) might have blunted the expected adipogenic response. Thus, these initial results pointed at the need to not only examine activators of a metabolic pathway but also potential "inhibitors" or "controllers". In addition, from these data we concluded that the sustained upregulation of MLXIPL and SREBF1 due to feeding high-dietary starch was suggestive of "metabolic imprinting", i.e., long-term upregulation of adipogenic transcription regulators due to exposure of high dietary starch at a young age. Such an effect, if it occurs in cattle intramuscular adipocytes, would lead to further enrichment (Rosen and MacDougald, 2006) of intramuscular adipocytes at a more mature age of the animal. Recent work revealed that methylation status of PPARγ in rodent preadipocytes can alter transcription of the gene and induction of cell differentiation (Fujiki et al., 2009), which suggests that adipogenic transcription regulators (and potentially enzymes) might be regulated via epigenetic mechanisms. Further detailed studies will be required to determine potential epigenetic regulation of adipogenic and lipogenic genes in cattle fed high-starch diets at an early age. We are currently testing potential metabolic imprinting in longissimus lumborum of early-weaned steers (Moisa et al., 2010).
Perspectives
Substantial amount of resources have been devoted to sequencing and annotating DNA sequences in the bovine genome. From a nutritional standpoint, practical use of this fundamental information can only be accomplished if there is better understanding of the functional relevance of gene networks and pathways that are affected due to nutrition and/or physiological state, e.g. PPAR signalling in adipose and liver, immune responses in liver, etc. However, a real challenge is to develop bioinformatics tools that would allow for a "true" systems biology approach capable of handling large amounts of data produced by the "old" (e.g., microarray) and new technologies (e.g., next generation sequencing). Such systems biology approach holds great promise for livestock science; in particular, we envision its use to improve diet formulation for dairy cows in the near-to-long term; that would constitute a concrete translational effort of the holistic biological knowledge generated by systems biology to the feedbunk. The ultimate goal of that effort is to improve the efficiency of agricultural systems and animal health to benefit society.
References
Allen, M. S., Bradford, B. J. and Harvatine, K. J. 2005. The cow as a model to study food intake regulation. Annu. Rev. Nutr. 25:523-547.
Ballou, M.A., Gomes, R.C., Juchem, S.O., and DePeters E.J. 2009. Effects of dietary supplemental fish oil during the peripartum period on blood metabolites and hepatic fatty acid compositions and total triacylglycerol concentrations of multiparous Holstein cows. J. Dairy Sci. 92:657-669.
Bionaz, M., Baumrucker, C. R., Shirk, E., Vanden Heuvel, J. P., Block, E., and Varga, G. A. 2008. Short communication: Characterization of Madin-Darby Bovine Kidney Cell Line for Peroxisome proliferator-Activated Receptors: Temporal Response and Sensitivity to Fatty Acids. J. Dairy Sci. 91:2808-2813.
Bionaz, M., Rodriguez-Zas, S. L., Everts, R. E., Lewin, H. A., and J. J. Loor. 2007b. MammOmics?: transcript profiling of the mammary gland during the lactation cycle in Holstein cows. J. Dairy Sci. 90(Suppl. 1):272 (Abs.). Bionaz, M. and J.J. Loor, 2008. Gene networks driving bovine milk fat synthesis during the lactation cycle. BMC Genomics 9:366.
Bionaz, M. and J.J. Loor, 2011. Gene networks driving bovine mammary protein synthesis during the lactation cycle. Bioinform. Biol. Insights 5:1-16.
Benjamini, Y. and Y. Hochberg. 1995. Controlling the false discovery rate - a practical and powerful approach to multiple testing. J. Royal Stat. Soc. B-Methodol. 57:289-300.
Desvergne, B., Michalik, L., and W. Wahli, 2006. Transcriptional regulation of metabolism. Physiol. Rev. 86:465-514.
Fernyhough, M.E., Okine, E., Hausman, G., Vierck, J.L., and M.V. Dodson. 2007. PPAR and GLUT-4 expression as developmental regulators/markers for preadipocyte differentiation into an adipocyte. Domest. Anim. Endocrinol. 33:367-78.
Fujiki, K., F. Kano, K. Shiota, and M. Murata, 2009. Expression of the peroxisome proliferator activated receptor gamma gene is repressed by DNA methylation in visceral adipose tissue of mouse models of diabetes. BMC Biol. 7:38.
Graugnard, D., S.L. Rodriguez-Zas, D.B. Faulkner, L.L. Berger, R.E. Everts, H.A. Lewin and J.J. Loor, 2007. Temporal longissimus muscle gene expression profiles due to plane of dietary energy in early-weaned Angus steers. J. Dairy Sci. 90:540.
Graugnard, D., Piantoni, P., Bionaz, M., Berger, L.L., Faulkner, D.B., and J.J. Loor. 2009. Adipogenic and energy metabolism gene networks in longissimus lumborum during rapid post-weaning growth in Angus and Angus x Simmental cattle fed high- or low-starch diets. BMC Genomics 10:142.
Graugnard, D.E., Berger, L.L., Faulkner, D.B. and J. J. Loor, 2010. High-starch diets induce precocious adipogenic gene network up-regulation in longissimus lumborum of early-weaned Angus cattle. Br. J. Nutr. 103:953-63.
Grossman, M. and Koops, W. J. 2003. Modeling extended lactation curves of dairy cattle: A biological basis for the multiphasic approach. J. Dairy Sci. 86:988-998.
Hocquette, J.F., I. Cassar-Malek, A. Scalbert and F. Guillou, 2009. Contribution of genomics to the understanding of physiological functions. J. Physiol. Pharmacol. Suppl 3:5-16.
Huang da, W., Sherman, B. T. and R. A. Lempicki. 2009. Systematic and integrative analysis of large gene lists using david bioinformatics resources. Nature Protoc. 4:44-57.
Hughes, L.M., Bao, J., Hu, Z.L., Honavar, V., and J. M. Reecy. 2008. Animal trait ontology: The importance and usefulness of a unified trait vocabulary for animal species. J. Anim. Sci. 86:1485-91.
Kadegowda, A. K., Bionaz, M., Piperova, L. S., Erdman, R. A., and J. J. Loor. 2009. Peroxisome proliferator-activated receptor-gamma activation and long-chain fatty acids alter lipogenic gene networks in bovine mammary epithelial cells to various extents. J. Dairy Sci. 92:4276-4289.
Khan, M. J., E. Schmitt, M. A. Ballou, E. J. DePeters, S. L. Rodriguez-Zas, R. E. Everts, H. A. Lewin, J. K. Drackley, and J. J. Loor. 2010. Liver transcriptomics in Holstein cows fed lipid supplements during the peripartal period. J. Dairy Sci. 93(E-Suppl. 1):1060.
Lehnert, S.A., A. Reverter, K.A. Byrne, Y. Wang, G.S. Nattrass, N.J. Hudson and P.L. Greenwood, 2007. Gene expression studies of developing bovine longissimus muscle from two different beef cattle breeds. BMC Dev Biol. 7:95.
Litherland, N.B., M. Bionaz, R.L. Wallace, J.J. Loor, and J.K. Drackley. 2010. Effects of the peroxisome proliferator-activated receptor-alpha agonists clofibrate and fish oil on hepatic fatty acid metabolism in weaned dairy calves. J. Dairy Sci. 93:2404-2418.
Loor, J.J. 2010. Genomics of metabolic adaptations in the peripartal cow. Animal 4:1110-1139.
Loor, J. J., M. Bionaz, and G. Invernizzi. 2011. Systems biology and animal nutrition: insights from the dairy cow during growth and the lactation cycle. In Systems Biology and Livestock Science. M. F. W. te Pas, H. Woelders, and A. Bannink, ed., Wiley-Blackwell. (in press)
Loor, J. J., Dann, H. M., Everts, R. E., Oliveira, R., Rodriguez-Zas, S.L., Lewin, H.A., and J. K. Drackley. 2005. Temporal gene expression profiling of liver from periparturient dairy cows reveals complex adaptive mechanisms in hepatic function. Physiol. Genomics 23:217-226.
Loor, J. J., Dann, H. M., Janovick-Guretzky, N. A., Dann, H.M., Oliveira, R., Rodriguez-Zas, S.L., Lewin, H.A., and J. K. Drackley. 2006. Plane of nutrition prepartum alters hepatic gene expression and function in dairy cows as assessed by longitudinal transcript and metabolic profiling. Physiol. Genomics 27:29-41.
Loor, J. J., Everts, R. E., Bionaz, M., Dann, H.M., Morin, D.E., Oliveira, R., Rodriguez-Zas, S.L., Drackley, J.K., and H.A. Lewin. 2007. Nutrition-induced ketosis alters metabolic and signaling gene networks in liver of periparturient dairy cows. Physiol. Genomics 32:105-116.
McNamara, J.P., 2010. Integrating transcriptomic regulation into models of nutrient metabolism in agricultural animals. 3rd EAAP International Symposium on energy and protein metabolism and nutrition. Parma, Italy, September 6-10, 2010.
Mo, M.L., and B.Ø. Palsson. 2009. Understanding human metabolic physiology: a genome-to-systems approach. Trends Biotechnol. 27:37-44.
Moisa, S.J., D. W.Shike, D. B.Faulkner and J.J. Loor, 2010. Carbohydrate-responsive element binding protein (MLXIPL) and PPAR gene network expression in longissimus lumborum of early-weaned and normal-weaned Angus steers fed a high-starch diet during the growing phase. J. Dairy Sci. 93 (E-Suppl. 1):Abs(730).
Myers, S.E., D.B. Faulkner, T.G. Nash, L.L. Berger, D.F. Parrett and F.K. McKeith, 1999b. Performance and carcass traits of early-weaned steers receiving either a pasture growing period or a finishing diet at weaning. J Anim Sci. 77:311-22.
Okamura, M., H. Kudo, K. Wakabayashi, T. Tanaka, A. Nonaka, A. Uchida, S. Tsutsumi, I. Sakakibara, M. Naito, T.F. Osborne, T. Hamakubo, S. Ito, H. Aburatani, M. Yanagisawa, T. Kodama and J. Sakai, 2009. COUP-TFII acts downstream of Wnt/beta-catenin signal to silence PPARgamma gene expression and repress adipogenesis. Proc. Natl. Acad. Sci U S A. 106:5819-5824.
Palsson, B. 2010. Metabolic systems biology. FEBS Lett. 583:3900-4. Rosen, E.D., and O.A. MacDougald, 2006. Adipocyte differentiation from the inside out. Nat Rev Mol Cell Biol 7:885–896.
Schoonmaker, J.P., S.C. Loerch, F.L. Fluharty, H.N. Zerby and T.B. Turner, 2002. Effect of age at feedlot entry on performance and carcass characteristics of bulls and steers. J Anim Sci. 80:2247-54.
Shahzad, K., M. Bionaz, and J. J. Loor. 2011. Analysis of bovine liver transcriptomics data due to level of prepartal dietary energy using two bioinformatics approaches. J. Dairy Sci. 91(E-Suppl. 1):Abs.M249.
Smith, S.B., H. Kawachi, C.B. Choi, C.W. Choi, G. Wu and J.E. Sawyer 2009. Cellular regulation of bovine intramuscular adipose tissue development and composition. J Anim Sci. 87(14 Suppl):E72-82.
Thering, B. J., Graugnard, D. E., Piantoni, P., and J. J. Loor. 2009. Adipose tissue lipogenic gene networks due to lipid feeding and milk fat depression in lactating cows. J. Dairy Sci. 92:4290-300.
Wang, Y.H., K.A. Byrne, A. Reverter, G.S. Harper, M. Taniguchi, S.M. McWilliam, H. Mannen, K. Oyama and S.A. Lehnert, 2005a. Transcriptional profiling of skeletal muscle tissue from two breeds of cattle. Mamm Genome. 16:201-10.
Wang, Y.H., N.I. Bower, A. Reverter, S.H. Tan, N. De Jager, R. Wang, S.M. McWilliam, L.M. Cafe, P.L. Greenwood and S.A. Lehnert, 2009. Gene expression patterns during intramuscular fat development in cattle. J Anim Sci. 87:119-30.
Wittkopp, P.J., 2007. Variable gene expression in eukaryotes: a network perspective. J. Exp. Biol. 210:1567-1575.
Figure 1. From Wikipedia: A DNA microarray is a collection of microscopic DNA spots attached to a solid surface. Scientists use DNA microarrays to measure the expression levels of large numbers of genes simultaneously or to genotype multiple regions of a genome. Probetarget hybridization is usually detected and quantified by detection of fluorophore-labeled targets to determine relative abundance of nucleic acid sequences in the target. The core principle behind microarrays is hybridization between two DNA strands, the property of complementary nucleic acid sequences to specifically pair with each other by forming hydrogen bonds between complementary nucleotide base pairs. Fluorescently labeled target sequences that bind to a probe sequence generate a signal that depends on the strength of the hybridization determined by the number of paired bases, the hybridization conditions (such as temperature), and washing after hybridization.
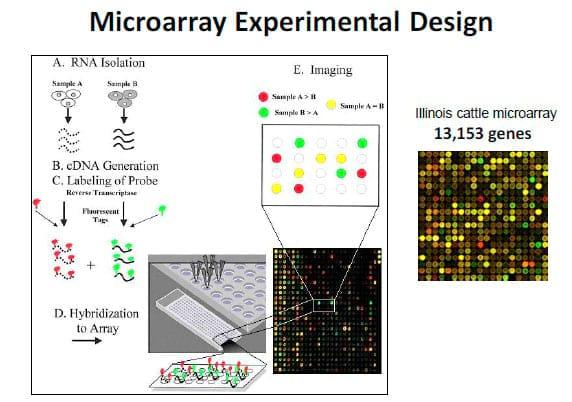
Figure 2. From Wikipedia: Bioinformatics is the application of computer science and information technology to the field of biology and medicine. Bioinformatics deals with algorithms, databases and information systems, web technologies, etc, for generating new knowledge of biology and medicine. Web-accessible bioinformatics tools include KEGG and DAVID. This flowchart represents the framework used in the Loor laboratory for microarray data mining.
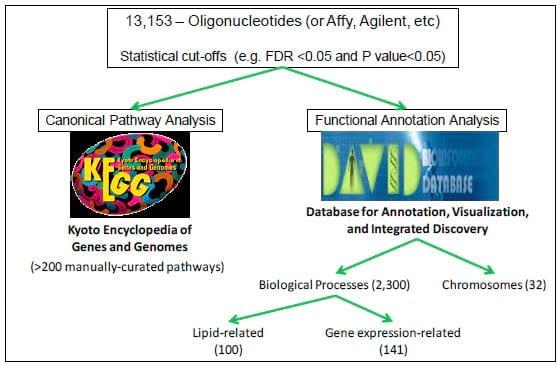
Figure 3. From Wikipedia: A gene regulatory network or genetic regulatory network (GRN) is a collection of DNA segments in a cell which interact with each other indirectly (through their RNA and protein expression products) and with other substances in the cell, thereby governing the rates at which genes in the network are transcribed into mRNA. Our recent work has identified PPARgamma as an important nuclear receptor that seems to be an important regulator of adipogenesis in adipose tissue and milk fat synthesis in the mammary gland (see Bionaz and Loor, 2008; Loor, 2010). Shown is the network encompassing PPARgamma in subcutaneous adipose tissue and the known target genes that are present in the Illinois microarray platform. Red denotes upregulation and green downregulation of expression in cows overfed a moderate energy diet vs. controls at ca. -14 days relative to parturition.