While each meat quality trait is often thought to be unique and independent, in reality most of the commonly measured meat quality traits are associated, or correlated, with each other.
The total variation among these correlated traits can be partitioned into variation caused by one or more common factors and a random amount of environmenta variation that is unique to each individual trait. Figure 1 shows a pie chart that represents the total variation in the meat quality traits in the trial. The pie can be divided into two slices; one slice is the variation common to all of the traits and the other slice is the random environmental variation that makes each measurement different from the next measurement. Seventeen traits, including days to 275 lbs. (125 kg), carcass backfat thickness, and 15 meat quality traits were evaluated on 436 pigs sired by PIC280, PIC327, and PIC337 boars.
These traits were analyzed using a statistical procedure called
Common Factor Analysis to identify factors that contribute to the common variation among the traits.
Common Factor Analysis is a data reduction technique to reduce the number of traits down to a smaller and more manageable group of factors. While all meat quality traits are interesting to examine from a scientific standpoint, identification of common factors associated with meat quality can lead to efficiencies in time and cost savings by collecting data only on the most predictive traits of overall quality. A summary of the results from the
Common Factor Analysis to identify factors associated with meat quality is described in Figure 2.
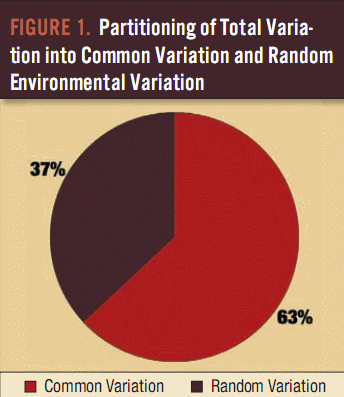
COMMON FACTOR ANALYSIS
A total of 436 commercial barrows and gilts sired by 14 PIC280 boars, 14 PIC327 boars,and 16 PIC337 boars were used in the analyses. The number of pigs ranged from 127 to 160 pigs from each sire line. The pigs were harvested at two commercial pork processing plants where carcass quality measurements were taken using a Fat-O-Meater. Loin pHu, Minolta color measurements, subjective loin color, marbling and firmness scores were recorded 22 hours post-mortem. Boneless loins were purchased by PIC and shipped to the University of Illinois where they were aged for 14 days prior to evaluation for additional meat quality and sensory traits. A brief description of the traits evaluated in the trial is presented in Table 1.
CORRELATIONS BETWEEN TRAITS
Presented in Table 2 are the correlations between the traits evaluated in the trial and used in the Common Factor Analysis. Through a series of statistical calculations, details of which are beyond the objectives of this article, the original 17 variables were further reduced down to 9 variables that explained the variation. The "rule of thumb" generally accepted by statisticians in a Common Factor Analysis is that a factor is important in explaining the common variation if there are a minimum of three variables that exceed a critical value of -.5 or +.5. So with nine traits, there are a maximum of three factors that explain the common variation. Shown in Table 3 are the nine variables and the three factors.
The variables for each factor that exceed the -.5 or +.5 requirement are shown in the bold font. The analysis is a stepwise approach where the first factor is the most important and accounts for the most variation, the second factor is the next most important and so forth.
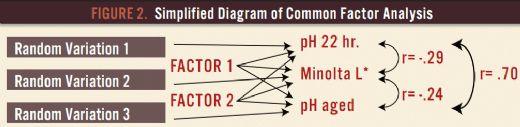
Common Factor Analysis is a multivariate statistical technique that partitions the variation among correlated traits into a smaller group of latent factors. Shown above is a diagram of a Common Factor Analysis involving three traits: loin pHu 22 hours post-mortem; loin Minolta L*; and loin pH after the loin has been aged for 14 days. Between each pair of traits is a correlation, which is designated as "r." A correlation ranges in value from -1 to +1. The closer the correlation coefficient is to -1 or +1, the stronger the association between the two traits. A correlation of 0 indicates there is no association between two traits. The correlation between pHu at 22 hours post-mortem and pH after 14 days of aging is .70.
Since the correlation is close to +1, this means there is a fairly strong relationship between the two traits. As the value of pHu at 22 hours post-mortem increases, so does the value for pH after 14 days of aging. The correlation between pHu at 22 hours post-mortem and Minolta L* is -.29. This means since the correlation is closer to 0 than -1, there is not as strong an association between the two traits. The minus sign means that as pH increases, Minolta L* decreases, thus indicating a darker muscle color. Looking closer at the diagram, there are two common factors (Factor 1 and Factor 2) which are believed to influence the three traits. These factors will be estimated in the statistical analysis. Random variation 1, 2 and 3 is the environmental variation that makes the measurements within each trait unique from pig to pig.
KEY RESULTS
FACTOR 1- Looking under the column "Factor 1" in Table 3, there are three traits with a value that exceeds the critical value of -.5 or +.5.
The traits are pHu at 22 hours post-mortem (.88), pH after the 14-day aging period (.73), and subjective loin firmness score (.59). Although subjective fi rmness score is not a direct measurement of muscle pHu, it is closely related to pHu, as indicated by the .60 correlation with pH at 22 hours post-mortem and the .46 correlation with pH after 14 days of aging (Table 2). Therefore, Factor 1 can be simply renamed as "pHu."
FACTOR 2- The three traits that exceed the -.5 or +.5 criteria listed under the column "Factor 2" in Table 3 are Minolta L* (.83), subjective Japanese color score (-.72) and Minolta b* (.69). All three of these traits are measurements of loin muscle color. Factor 2 in Table 3 can then be re-named "Muscle Color."
FACTOR 3- Finally, under the column heading "Factor 3" in Table 3, the three traits that met the -.5 and +.5 criteria are intra-muscular fat percentage (.91), moisture percentage (-.76) and subjective loin marbling score (.54). Factor 3 can be re-named "Marbling." Note that a trait reaches the critical value of -.5 or +.5 only once.
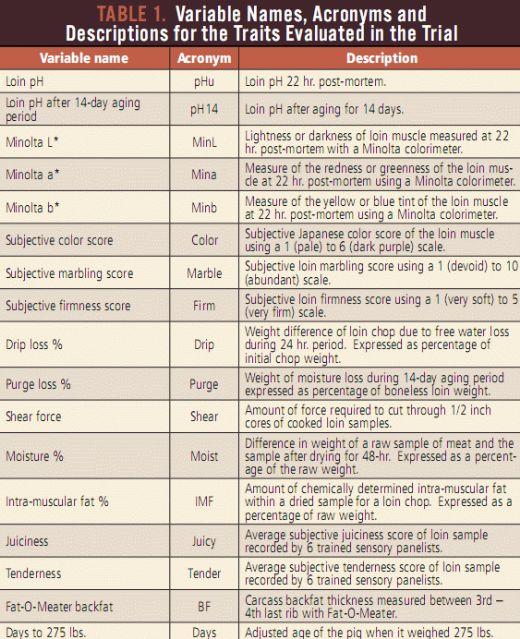
Click here to enlarge the image PRACTICAL APPLICATION
The Common Factor Analysis results reported here established the most important factor that accounts for the largest amount of common variation in meat quality was pHu, followed by color, and then marbling. While all meat quality measurements are interesting in their own respect from a scientific standpoint, if a person had to pick just one trait to measure, loin pHu is the trait of choice because it was the first factor identified in the analysis and thus accounts fothe most common variation between the meat quality traits. In PIC's genetic improvement program for meat quality, loin and ham pHu are measured in by-product pureline gilts and also in pedigreed commercial pigs as part of our Genetic Nucleus crossbred program. In 2007, more than 17,000 loin pH measurements were collected and used in PIC's genetic improvement program. Each data point adds to the accuracy of the crossbred breeding value of its sire. Measuringloin and ham pH is relatively easy to do in the cooler while carcasses are hanging on the rail. If additional information is desired on meat quality, then, based on results from the Common Factor Analysis, one would bone out loins, measure muscle color, and score the loins for the amount of intra-muscular fat or marbling. Interestingly, in this analysis, sensory traits such as tenderness and juiciness did not meet the critical values required to be a factor in predicting meat quality.
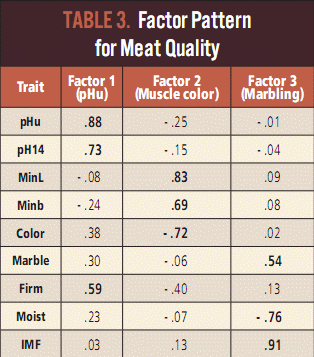