Introduction
An ideal technique for the measurement of body growth and composition in livestock animals is noninvasive, non-destructive, accurate, easy to perform and applicable to a wide range of ages and body weights (Ferrell & Cornelius, 1984). Non-invasive, also known as non-destructive, techniques allow tissue changes in the same animal to be followed to study development over different stages. However, invasive techniques, such as serial slaughter of collaterals or descendant animals and dissections, continue to be used to determine body composition or calibrate several devices. Based on image analysis, Font-i-Furnols et al. (2015) and Carabús et al. (2015a,b) analysed serial slaughter data from 30 to 120 kg for gilts of different genotypes and pigs of different sexes, respectively, and obtained prediction equations for body composition based on computed tomography images. In these studies, serial slaughter was used for device calibration and was replaced by in vivo estimations once the equations were validated. In others works, such as those by Gjerlaug et al. (2012) and Lambe et al. (2013), dissections were not used, and information was obtained directly from image analysis without the application of a prediction equation. Thus, in vivo estimations can be performed using non-invasive technologies based on image analysis. The five main non-invasive technologies are ultrasound (US), visual image analysis by monitoring (VIA), dual-energy X-ray absorptiometry (DXA), magnetic resonance imaging (MRI), and computed tomography (CT). Image processing technologies have been developed rapidly. These technologies are reliable and can quantitatively characterise complex sizes, shapes and densities of tissue in live animals. However, these five technologies are very different, and although they can be used for the same purpose, each one has different specifications that are described, in detail, in the present paper. The purpose of this paper is to provide an overview of the technologies and associated methodologies for studying pig composition in vivo, describe how these techniques work and their main applications and, finally, to present certain of the most relevant results obtained in body composition of live pigs using these technologies (US, VIA, DXA, MRI and CT).
Non-invasive technologies to evaluate pig composition
A method should be easy to understand and practical to apply, it should be user-friendly, with the procedures and concepts for taking samples or recording information easily understood and with little chance for misinterpretation.
Methods of estimating body composition are comprised of two types. They either describe the chemical composition of the body or the anatomical distribution of its tissues. In each case the techniques used can be either invasive or non-invasive to the animal (Szabó, 2001).
Several non-invasive techniques are commonly used in live pigs that are calibrated using invasive or destructive methods as a reference (slaughter and dissection, biopsy, etc). A common feature of most non-invasive techniques for body or carcass composition measurements is a reliance on electromagnetic or mechanical energy, which can pass completely or partially through body or carcass tissue, such as muscle, adipose tissue and bone (Scholz et al., 2015), with the exception of VIA, that provides images directly from one or several cameras.
The accuracy of estimation is one of the key points that will determine the time for the image acquisition and the cost of the device. Therefore, there are two main factors affecting this accuracy: correlation between the measurement on the image and on the body part and correlation between body part and body composition (tissue or chemical) (Szabó, 2001).
a) Visual image analysis by monitoring
The VIA is the acronym for visual image analysis by monitoring, also known as video image analysis and computed aided design. The VIA can include one or more cameras to acquire 2D images or video images. This technique was developed in the USA specifically for beef carcass evaluation in the early 1980s (Cross et al., 1983) and it is mainly used on line to estimate carcass characteristics. Nevertheless, it can also be used (not always on line) to predict the intramuscular fat or colour in chicken and turkey, with a R2 =0.22-0.74 (Chmiel et al., 2011), tenderness in beef (R2 =0.12-0.70; Li et al., 1999; Sun et al., 2012) and lean meat yield in live pigs (Doeschl Wilson et al., 2005). Published results have demonstrated that VIA can measure the growth rate and weight of pigs (Schoefield, 1990; White et al., 2004). Doeschl-Wilson et al. (2005) used it to describe pig growth in terms of size and shape and concluded that the analysis of shape data combined with composition data from dissected carcasses was significantly related (p<0.05) to carcass composition at all growth stages and that this relationship varied among genetic populations.
In addition, VIA has been extensively used to classify carcasses into payment categories and to improve the consistency of SEUROP classification compared to visual appraisal (Allen & Finnerty, 2000; Font i Furnols & Gispert, 2009; Craige et al., 2012; Engel et al., 2012). The image process to capture all the pig takes less than 1.5 s (Schofield, 2007).
This technique is useful in agricultural applications, and one of the biggest advantage is that no human-animal interaction is required. However, as a drawback, the information provided solely concerns the external portion of the body, and no internal image of the pig is obtained. To monitor a population within an area, observation stations should be set throughout the survey area of interest. The distribution of observation must be carefully considered relative to efficiency in obtaining adequate measurements of the animals being monitored and avoiding bias in the results that could be induced by station placement (Meek, 2012). The proximity of electrical outlets for non-portable cameras, the proper positions of cameras (without death nooks), the light intensity, sensor sensitivity, type of flash, field of view, the cleanliness and dust control of the farm are major factors to consider when using this equipment. The cost of each system will vary depending upon if it is a movable device or not, the computer used, the complexity of the installation and the software and hardware needed. However, the actual cost of a movable device in a basic form is under 8,000 € (Schofield, 2007), the cost of a fixed device will depend on the type and number of cameras, and the market offers a range of prices.
b) Ultrasound
The US enables the acquisition of internal images for use in body composition evaluation, and this is one of the biggest advantages compared to VIA.
The way it works is by acoustic waves that are propagated through materials as perturbations in their physical structure. Consequently, acoustic properties of materials can be correlated to its macroscopic composition and structure.
There are two models for ultrasonic imaging: Amode (amplitude modulation) and B-mode (brightness modulation). A-mode is the simplest type of US. A single transducer scans a line through the body, and the echoes are plotted on screen as a function of depth. In B-mode US, more commonly known as 2D mode, a linear array of transducers simultaneously scans a plane through the body that can be viewed as a twodimensional image on screen. Doppler and A-mode (amplitude depth) ultrasound technology has been applied to swine research and production for the last 20 to 25 years, however, its limited accuracy led to the use of B-mode (brightness modality), real-time ultrasound.
The US has been tested since the early 1950s and it has been performed in live animals or carcasses to measure fat thickness and assess the quality of meat (Fortin et al., 2003). The US has also been used to study intramuscular fat in live pigs (Newcom et al., 2002) and in other species, such in cattle to study the repeatability and accuracy of US in measuring backfat (Brethour, 1992) and sheep to study estimation of sheep carcass composition using real-time ultrasound (Silva et al., 2006). It has been similarly used, either in vivo or post mortem, to estimate raw meat quality characteristics, mainly intramuscular fat, with the highest reported R2 of 0.92 in pork (Newcom et al., 2002). The accuracy of US in predicting carcass traits is variable and is dependent on species, ultrasonic instrumentation, and (or) the skill of the technician.
Additional factors to consider are the costs of the equipment, length of battery life and time required for image acquisition. The cost differs among A-mode and B-mode US, ranging from $450 to $10,000 (Knox et al., 2008). The length of battery life for portable units, and the proximity of electrical outlets for non-portable units are also factors to be considered. For portable machines, batteries are scheduled to operate continuously for ~3 h up to 9 h, and most US evaluations require approximately 1-2 min per image acquisition (Newcom et al., 2002; Mörlein et al., 2005; Bahelka et al., 2009; Maignel et al., 2010; Lakshmanan et al., 2012).
c) Dual-energy X-ray absorptiometry
The DXA is an improved form of X-ray technology that is used mainly to measure bone density (Fig. 1) and that can generate also 2D images. The determination of body and carcass composition by dual X-ray absorptiometry is based on the different X-ray attenuation coefficients at low and high X-ray spectral levels for soft tissue and bone mineral. The DXA provides a 2D scan image of the whole body or region of interest and the image can be analysed as a whole or regionally by semi-automatically or manually defining regions of interest (Mitchell et al., 2002). In addition to the amounts of soft lean or fat tissue and bone mineral content, DXA also provides a measure of bone mineral density (g/cm2 ). The DXA has been applied on a variety of farm animal (chicken: Swennen et al., 2004; Schreiweis et al., 2005; pigs: Mitchell et al., 1996; Kremer et al., 2012; sheep: Rozeboom et al., 1998; Scholz & Mitchell, 2010 and calves: Scholz et al., 2003) to predicting carcass and body composition. Also, previous studies evaluating DXA measurements in pigs have demonstrated high degrees of precision when compared to chemically-determined values (Brunton et al., 1993; Mitchell et al., 1998).
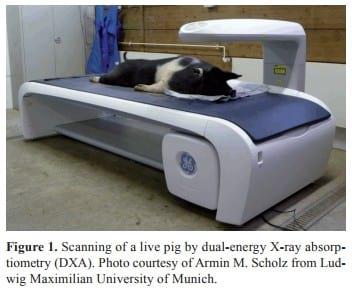
In any case, it is clear that the system manufacturer, instrument generation and software version can affect measurements (Tothill et al., 1994; Kistorp & Svendsen, 1998). Moreover, recent advances have markedly improved scan speeds (~3 min per scan) and measurement precision, increasing the attractiveness of DXA for body composition measurement of animals under both commercial and research conditions (Suster et al., 2003). However, regarding the time of image acquisition it is conditioned by the expense of accuracy, for instance, a whole body scan with a rather slow but very accurate pencil-beam scanner can take 35 min, whereas a whole body scan with a cone-beam scanner takes less than 3 min (Scholz et al., 2015). The expense to purchase a new unit averages $ 35,000 USD (Walpert, 2000).
d) Computed tomography
The CT has already been used in humans for many years for diagnostic and it is successfully utilized in experiments conducted with pig, sheep, poultry, rabbits and other species (Afonso, 1992; Romvári et al., 2005; Carabús et al., 2015a,b). It is a non-invasive technique that permits internal images of the patient, in this case, livestock animals (Fig. 2). The CT is based on the attenuation of X-rays passing through the body. The attenuation is the difference between the emitted X-ray and the X-ray received by the detector and is expressed in Hounsfield (HU) values in a matrix presented in the grey or HU scale, which represents colours from black (low density) to white (high density) (Fig. 3c). The CT image is, in most cases, a matrix of 256 × 256, 512 × 512 or 1064 × 1064 elements (pixels), depending on the setting and capacities of the instrument. The pixels are the smallest unit of a CT scan and it has a well described corresponding area. A cross section image of a body has always a slice thickness (1-10 mm usually). This means that, in fact, the pixel is a three-dimensional object, and it is called voxel. The object of interest is measured in a simple manner from many angles (360º), and thus the density of an individual voxel is not affected by the densities of the neighbouring voxels. Thus, structures of high and low density can be resolved, even if they are close to other structures. The distribution of the attenuation of an X-ray is mathematically calculated as a projection of reconstruction (Cann, 1988) and is presented by the software of the device as a 2D image. The image can be stored digitally and used later for evaluation or for analysis to generate prediction equations for carcass composition traits. Although the image is presented in 2D, the width of the X-ray used permits the calculation of the density and the real volume. As CT provides 3D images, the measurements obtained with this device are good predictors of body composition in live pigs (Luiting et al., 1995) and pig carcasses (Font i Furnols & Gispert, 2009). Due to the emission of X-rays, the equipment must be isolated in a room with leaded walls, and during scanning, the operator is in another room close to the device with a leaded window to visualise the scanning while it occurs.
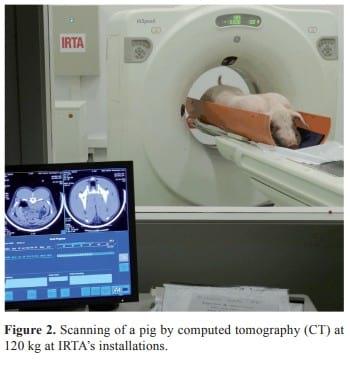
Computed tomography revolutionized diagnostic radiology with the introduction of spiral CT in the early 1990s, allowing, for the first time, the acquisition of volume data without the danger of misregistration or double registration of anatomical details. However, there was the necessity to make some advances such as to improve the volume coverage with increased longitudinal resolution, to include the simultaneous acquisition of more than one slice at a time and a reduction of the gantry rotation time. Advances took place in 1998, when multi-slice CT (MSCT) appeared, which typically offered simultaneous acquisition of four slices. The introduction of an eight-slice CT system in 2000 enabled shorter scan times, but did not yet provide improved longitudinal resolution. The latter was achieved with the introduction of 16-slice CT. In 2004, all major CT manufacturers introduced the next generation of MSCT systems with 32 or even 64 simultaneously acquired slices (Kohl, 2005). And nowadays 128 and more slices CT scans are also available.
An additional factor to consider is the cost of the equipment, which will directly depend on the CT type, supplier, technology and toolboxes. For instance, a new single slice CT ranges from $65,000 to $125,000 while a 64 slice CT (which is the most powerful) reaches from $250,000 to $450,000 (Block, 2014). Also, according to Kongsro et al. (2009) prices of a basic new CT scanner can vary between €300,000 and €600,000. However, it must be taken into account that there is an important market of second-hand devices from human medical facilities which are more economic. Finally, CT scanners can be mobile (Daumas & Monziols, 2011), i.e., placed inside a truck, which is very useful for working under farming conditions, or fixed, i.e., inside a room.
e) Magnetic resonance imaging
The MRI is a non-invasive diagnostic method that has been used in humans, domestic animals and, recently, livestock animals and carcass evaluation. Although the images acquired by means of MRI instruments look very similar to a CT image, the principle of the examination is entirely different (Baulain, 1997). In summary, during MRI examinations the atoms of the body, positioned in a strong magnetic field, take up energy from an external energizing source and re-emit as a function of time.
On an MRI scan some tissues appear to be brighter or darker than other tissues depending on the signal intensity. Relaxation times for protons can vary and two times are commonly measured − known as T1 and T2. T1 relaxation is known as longitudinal relaxation and T2 is known as spin-spin relaxation. White matter is brighter than grey matter in T1-weighted images and darker than grey matter in T2-weighted images. Consequently, T1 and T2 provide different intensities of images. The basic principle of this method relies on the properties of atomic nuclei with an odd number of protons or neutrons (or both), which absorb and reemit radio waves when placed in a powerful magnetic field. Tissues containing water molecules are used to create a signal that is processed to form an image of the body. Each tissue returns to its equilibrium state after excitation by the independent processes of T1 (spin-lattice) and T2 (spin-spin) relaxation. The intensity of the emitted signal is related to the number of protons present in a given volume. T1 and T2 are both very important and are constant values that depend on the studied tissue (and temperature). To generate a good MRI image, different tissues must be classified according to these constants. Either a T1 weighted image (the contrast between tissues is based on T1 differences) or T2 weighted can be generated. The MRI acquisitions are generally quite long, and T2 weighted acquisitions are longer than T1 weighted acquisitions. Thus, if T1 weighted acquisition enables sufficient contrast, the T2 weighted acquisition is not performed due to the long sequence required. Spin echo and gradient echo are the methods used to excite protons. The main difference between these methods is that the gradient echo sequence creates a chemical shift between water and fat, thereby improving the contrast between them. Gradient echo is generally used in “fat-suppression” sequences (Monziols et al., 2006). Spin echo is a more classic excitation method in which no chemical shift is induced, and the signal observed is directly linked to the T1 or T2 weighting. In fact, there are two types of MRI scanners: open MRI, also known as low-field scanner, and closed MRI, known as high-field scanner. High field scanner with 1.5-3 Tesla (measurement of magnetic force) provides high resolution, while open scanners usually have about 0.23 Tesla. The higher the field strength, the more powerful and faster the scanner.
The MRI has substantial potential for livestock evaluation and as a non-invasive technique for estimating the composition of pigs with different live weights (Mitchell et al., 2001; Kremer et al., 2013). Moreover, as a radiation-free device, there are no concerns for the use of MRI in humans and animals. Its price is very high, although it depends on different factors such as the type of MRI scanner (open or closed), the magnetic field itself (very related with the resolution) and the speed of image acquisition. Nevertheless, it is possible to find second hand low-field MRI devices for less than €100,000 (Kremer et al., 2013), However, regarding the speed, due to the magnetic field, more time is required for acquisition compared to other devices. Portable devices are possible but no common. Table 1 presents the main advantages and disadvantages of these technologies and devices.
Methodology for live pigs evaluation using imaging technologies
This section deals with the steps that are needed for the evaluation of live pigs with imaging technologies, from the preparation of the animals for its evaluation, the measurement procedure, the image analysis process and the development of prediction models.
First step: Preparation of the animal
Preparation of the animal for VIA and US. Anaesthesia or sedation of pigs is not required for VIA and US, but stable readings can only be obtained when the animal is not moving, unless the reason for the study is to examine animal movement (Kongsro, 2013). Preparation of the animal for DXA, CT and MRI. Anaesthesia or sedation is required for DXA, MRI and CT. First, the pigs must be fasted for several hours. The examination, then, begins with weighing of the pigs to calculate the dose of anaesthetic or sedative. A combination of two or more products is generally used to anaesthetise pigs, depending on the country´s law. Kolstad (2001) used azaperon (4 mg/kg live weight - LW) followed by phentotal sodium (5 mg/kg LW). Giles et al. (2008) used Yohimbine (10 mg/ml), and Carabús et al. (2015a) sedated animals intramuscularly with azaperon (0.1 mg/kg body weight - BW) and anaesthetised them with ketamine (0.2 mg/kg BW) and propofol (0.22 mg/kg BW, intravenously in the ear). Propofol was only administered at heavy weights. Sedation without the use of anaesthetic is also possible. Aasmundstad et al. (2014) used only an intramuscular injection of azaperone. It is also possible to use several type of gases (such as isoflurane), although in this case a mask must be placed on the pig’s face, which is not always convenient. In any case, it must be taken into account that the time required to acquire a DXA, CT or MRI image depends on the device used and, as explained previously, it depends on the accuracy desired, on the slices scanned (single or multiple CT) and or the strength of the magnetic field of the MRI (open or closed). In general, CT is faster than MRI, which requires more time per image due to magnetic resonance. Consequently, the doses of anaesthetic or sedative will depend on the time required for scanning, the type of device and the number of images required per animal.
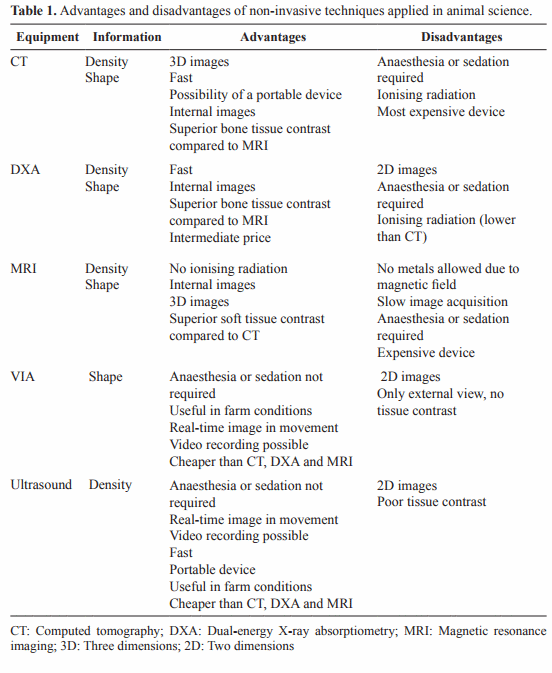
Differing from VIA and US, other major factors when working with DXA, CT and MRI and live animals is that an extra room close to the device is needed to perform the anaesthesia or sedation. Moreover, the decrease in body temperature due to anaesthesia must be compensated by providing a heating system, blankets or other options to avoid possible future health problems.
Second step: Measurement procedure
Measurement procedure for the VIA. A fixed position of the animal or human-pig interaction is not required. However, it is preferable to have calm pigs because the animals are monitored and this system provides images by video or photo camera from which measurements can be obtained manually or using specific software.
Measurement procedure for the US. Although anaesthesia is not required, the animal must be fixed by cage or by human restriction. Moreover, it is very important to keep the animal calm, and this technique must be performed by trained technicians. Once the animal is fixed, a specific gel that improves conductivity is applied to the region to be examined. Depending on the type of US device used, the results are presented automatically as an image on a portable screen (Fig. 3a) directly or as the value of the measurements obtained (subcutaneous fat, loin eye area, etc.).
Measurement procedure of DXA. This technique requires a calm, immobilised pig. The measurement of body composition by the DXA system is based on the differential attenuation of low and high-energy X-rays. The fat, lean and bone content are determined for each pixel of a total body scan. The most important variable when using a DXA device are the radiation source, the voltage (also known as photo peaks, which are the differential attenuation between low and high voltage), the scan speed and the longitudinal section distance. The basic theory and methodology for measuring body composition by DXA is similar to that for dual-energy photon absorptiometry, which has been described in detail by Peppler & Mazess (1981) and Gotfredsen et al. (1984). The whole body composition estimation is available immediately after the scan is completed. A regional analysis to quantify the 2D tissue distribution requires manual manipulation time, depending on the number and anatomical characteristics of the regions of interest (Scholz et al., 2015).
Measurement procedure of the CT. Similar to DXA, the use of CT for livestock animals requires anaesthetic or sedatives. The CT variables differ on the device and the purpose of the exam. One of the important instrumental settings for CT is the type of acquisition image: Helical or Axial. Helical CT is most prevalent because it is faster, but conventional step-and-shoot, axial technique, is used for high resolution. Other variables, such the voltage, the intensity, the matrix dimensions and the slice thicknesses are also considered in the CT image acquisition. The instrumental settings used by Carabús et al. (2015a) were 140 kV, 145 mA, pixels matrix of 512 × 512, axial, and two different slice thicknesses: 7 or 10 mm. Konsgro (2013) used two different energy levels of 80 kV and 140 kV, pixel spacing of 0.933 × 0.933 mm and 5-mm slice thickness and their combination to study intramuscular fat in live pigs. The accuracy and definition of the image will vary depending on these variables
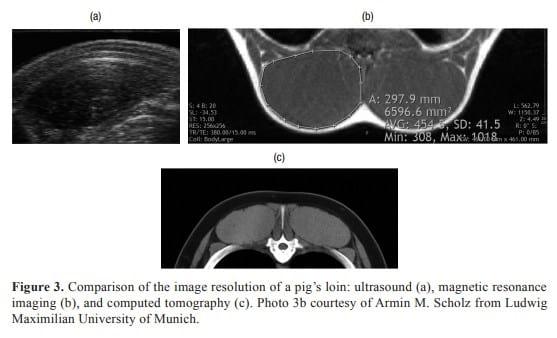
Measurement procedure of the MRI. As explained for DXA and CT, to perform MRI, the pig must be calm and immobile, which is primarily achieved by the use of anaesthesia or sedatives. Once the animal is anaesthetised or sedated, it is placed on the diagnostic table of the device and can be handled by a PVC cradle, special inflatable plastic or blankets (Mitchell et al., 2001). No magnetic object is allowed due to the magnetic field created. It is very important to check the pig’s ear tag and verify that it does not contain magnetic parts; otherwise, the exam will not be performed properly. An MRI or CT scan usually start with a so-called scout or localizer to define the zone studied and the positions and directions of the slices selected. The positions of the scans are determined by anatomical points selected by the operator. It is possible to scan a specific anatomical region or the whole pig. Figure 3b shows an image of a loin obtained by MRI. The MRI variables depend on each device and the requirements of the study. Important variables include the T1 and T2 constants, the sequence used (spin or echo), the time of repetition, the time between two consecutive radiofrequency pulse signals or between successive excitations (TR), the time between echoes, between the middle of the exciting radiofrequency pulse signal and the middle of spin echo production (TE), the flip angle (also called tip angle, is the amount of rotation the net magnetization experiences during application of a radiofrequency pulse and it is critical for determining both signal intensity as well as image contrast), matrix dimension and the slice thickness. Kremer et al. (2013) used a protocol consisting of a T1 weighted spin echo sequence (TR of 380 ms, TE of 15 ms, flip angle 90), a field of view of 461 × 461 mm with an image matrix of 256 × 256 pixels, and a transversal slice thickness of 15 mm with a distance factor of 0.25. For a T1- weighted sequence with a TR of 300 ms and a TE of 17 ms, the fat tissue pixels have rather high signals intensities, whereas the non-fat pixels have lower signal intensities (Scholz et al., 2015). However, this pattern differs for cold objects (Monziols et al., 2006). As in CT, the temperature of the object is important; a cold carcass and a live body will not present the same signal intensities, even for the same animal.
Third step. Image analysis procedure
Image analysis can be performed with varying degrees of automation, and an important issue is the software used. Each device typically includes its own software, but researchers usually require more information than that generally provided. Software used in published works include Visual Pork (Bardera et al., 2012), ATAR (Animal Tomogram Analysis Routines)- STAR (Lambe et al., 2013), Osirix (Rosset et al., 2004), Dicom Works 1.3.5, and Lunar 4.7e (Kremer et al., 2013). Images can be analysed in three different ways: using phenotypic measurements, such as linear measurements, areas or volumes; using segmentation based on the application of algorithms to classify each voxel according to its density or signal intensity; or using the volume distribution by HU value or signal intensity.
Phenotypic measurements of specific regions such as the 3rd-4th last rib or P2 (fat thickness measured at the midline at the level of the head of the last rib) in livestock animals have been studied extensively (Font i Furnols & Gispert, 2009; Engel et al., 2012) for three main reasons. First, these measurements in live pigs are good predictors of carcass composition, that is, the measurements obtained from the image are related to the lean content of the carcass, and the scientific community uses these measurements for prediction. Second, the meat industry uses these measurements in carcasses to estimate the lean meat content. Third, measuring the same region in live pigs or carcasses allows the results of different experiments to be compared.
Segmentation uses the differences between values or colours in grey-scale to classify tissues as lean, fat and bone. However, segmentation demands special attention when using measurements obtained from pixels or voxels because there is not an agreed standard for segmentation based on HU values or signal intensity and segmentation depends on the specific equipment, its calibration and researcher preference. Differences in CT protocols may lead to variations of HU values of up to 20% (Scholz et al., 2015).
Image segmentation can be divided into different approaches. Thresholding is the method used in the majority of experiments involving CT, MRI and live animals. Thresholding segmentation is based on assumptions of specific mass attenuation coefficients for different body or carcass tissues. The histogram shapebased method analyses the peaks, valleys and curvatures of the smoothed histogram. Histograms have been used and studied extensively in CT of growing pigs. Font-i-Furnols et al. (2015) used histograms to segment fat, lean and bone tissue in live pig images using the volumes of fat, lean and bones obtained by the sum of voxels distributed with values of HU between -149 and 0, 1 and 150, and 151 and 1400, respectively. Chang et al. (2011) reported HU values between -20 and -200 for visceral and subcutaneous fat in minipigs, and Gjerlaug-Enger et al. (2012) distinguished fat, lean and bones based on HU values of -200 to 0, 0 to 200 and greater than 200, respectively. Image segmentation is the last step if the imaging technique provides sufficient information to obtain the parameters of interest. However, external factors such as environmental temperature during CT scanning or the animal’s internal temperature can affect the tissue density and, consequently, the values obtained. Variations of temperature and differences in the acquisition parameters of each device underlie the lack of global segmentation for all devices. According to Scholz et al. (2015), differences in the calculation of CT densities for lean meat result in different lean meat weights for similar lean meat volumes, complicating the harmonisation of acquisition parameters among different countries or among various CT scanners.
Another method of image analysis is the development of prediction equations using the distribution of pixels (voxels) values as predictors, with or without additional linear or area measures obtained directly from images (Carabús et al., 2015b; Font-i-Furnols et al., 2015). In this case, a fourth step is needed as explained below.
Image analysis procedure for VIA. External linear measurements, perimeters and areas can be obtained from a 2D image, and reconstruction is feasible, including volume measurements, when an extra image is added. However, most of the information obtained is related to shape (White et al., 2004; Doeschl-Wilson et al., 2005) and behaviour and evaluation of gait analysis (Kongsro, 2013). No internal images are obtained.
Image analysis procedure for US. The US can provide areas, perimeters and linear measurements in addition to intramuscular fat content. The technician uses the machine to measure the area of the loin eye, its depth and how much fat is deposited over the loin eye. Different locations and measurements have been reported (Table 2). McKeith et al. (2010) studied loin muscle area, loin muscle depth, and backfat depth at the 10th rib and last rib from commercial finishing pigs. Image analysis procedure for DXA, CT and MRI. Linear measurements, perimeters and areas are obtained by these three devices. The volumes at any point and between any points can also be obtained by MRI and CT. Although DXA generally produces 2D images, 3D reconstruction images are also possible (Humbert et al., 2012); however, even though the three devices allow the same types of measurements, the image resolution differs among the devices and depends on the tissue analysed, its density and the target. Thus, DXA is more specific for dense tissues with low hydration, such as bones. The CT has adequate resolution for dense and medium-dense tissues. While MRI does not differ substantially from CT, MRI has better resolution and provides detailed results for soft tissues (Szabó et al., 2009). Table 2 presents examples of measures obtained using the different technologies.
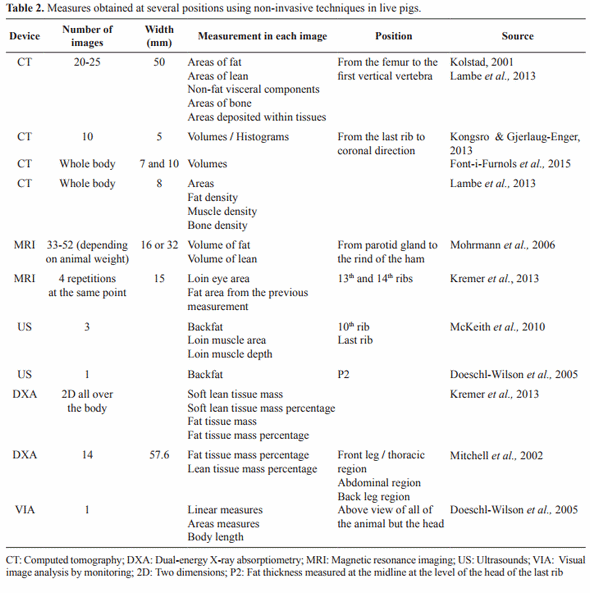
Fourth step: Predictions
Prediction is the last step (not always necessary) and transforms the data from the image analysis into variables of interest for the pig sector (kilograms of fat, lean meat percentage, etc.) by applying previously developed prediction equations. Accurate precision of the prediction is important to obtain reliable results. Thus, the technique used must be well calibrated and previous calibration or validation using dissections is occasionally necessary. Predictions are acquired from different sources, including the measurements obtained from the devices (backfat, loin muscle area, volumes, segmentation, etc.) and external data such as body weight, genotype, sex, diet, health status, and farm density (Lambe et al., 2013; Carabús et al., 2015b). To obtain a reasonable prediction, the prediction equation must be accurate, as indicated by a high coefficient of determination (R2 ) and a low error (root mean square error- RMSE). Examples of predictions obtained from pig image analysis for the carcass characteristics of live pigs and their accuracy are presented in Table 3.
Utility of these technologies for livestock animals
Non-invasive technologies have a number of applications for research, industry and both purposes: breeding and selection: effect of genetic and sex type; nutrition: effect of diet; health: veterinary diagnostic; medicine: animal as a model for human research; slaughter plant: carcass and cuts composition; processing plants: cutting optimisation and cuts composition (virtual butcher). This paper primarily provides an overview of the first two applications.
Effect of genetic type evaluated by image analysis
At slaughter weight, carcass characteristics differ depending on genetics (Gispert et al., 2007). Kolstad et al. (1996) compared Landrace and Duroc growing pigs fed at maintenance. Landrace pigs contained more internal fat (2.28 vs 2.20 kg) and less inter/intramuscular fat (1.90 vs 2.26 kg) at the start of the maintenance feeding period than the Duroc pigs. Margeta et al. (2007) used MRI to study the influence of malignant hyperthermia syndrome (MHS) -genotype and feeding regime on the growth and development of muscle and fatty tissue in the whole body as well as in hams of hybrid pigs and concluded that different feeding regimes and MHS genetic statuses of pigs do not significantly influence the growth of muscle and fatty tissue in hams. The stage of maturity is a primary reason for reported genotype-dependent differences in carcass composition, and the effects are more pronounced when pigs of different mature weights are compared at the same weight than at the same age. There is some evidence that breeds differ in the relative growth rates of tissue in discrete anatomical regions, independent of degree of maturity (Fortin et al., 1987). Information on sow lines carrying genes for prolificacy (Fisher et al., 2003) is very valuable for breeding companies. Gjerlaug-Enger et al. (2012) used CT to calculate genetic variables for the growth rate of muscle, carcass fat, bone and non-carcass tissue from birth to 100-kg live weight of Landrace and Duroc genotype pigs. Mitchell et al. (2002) used DXA to determine the feasibility of predicting total body composition of live pigs of three different genotypes based on a single cross-sectional measurement. Carabús et al. (2011) used CT to study the phenotypic characteristics of three different genotypes at 30, 70, 100 and 120 kg. The Pietrain cross type exhibited a greater amount of ham, which is useful information for companies that use Pietrain pigs for their lean potential. As an example of an application not involving meat, Kongsro (2013) applied CT in live pigs to diagnose osteochondrosis, its heritability and genetic correlations to weight gain in specific age intervals, useful information for breeding companies. Ley (2013) declared CT “part of the routine genetic selection programs in modern times”. Modern CT permits the acquisition of more than 1100 slices per live pig in less than 1 min (Gjerlaug-Enger et al., 2012). Accordingly, testing 24 boars per day is a routine application at Topigs-Norsvin facilities in Norway. The information from the 1100 slices per potential breeding boar is processed to determine body composition phenotypes such as lean meat, fat, bone, primal cuts, live and carcass weight (Scholz et al., 2015).
Evaluation of the effect of sex by image analysis
Giles et al. (2008) used CT to study the differential growth and development of pigs and determined that differences in the weights of body components by sex were minimal at the starting 30 kg target BW but increased with increasing target BW (up to 150 kg).
Doeschl-Wilson et al. (2005) used VIA to evaluate the relationship between the body dimensions of live pigs and their carcass composition by sex and identified significant differences in the regression results between boars and gilts. Mitchell et al. (2001) used MRI in live pigs, including females and entire males. Four different experiments were performed, with the main objective of predicting carcass composition. The results of the different experiments were compared to identify the most accurate prediction of fat and lean content obtained by analysing the fat and muscle values of a specified number of slices within the ham and loin regions.
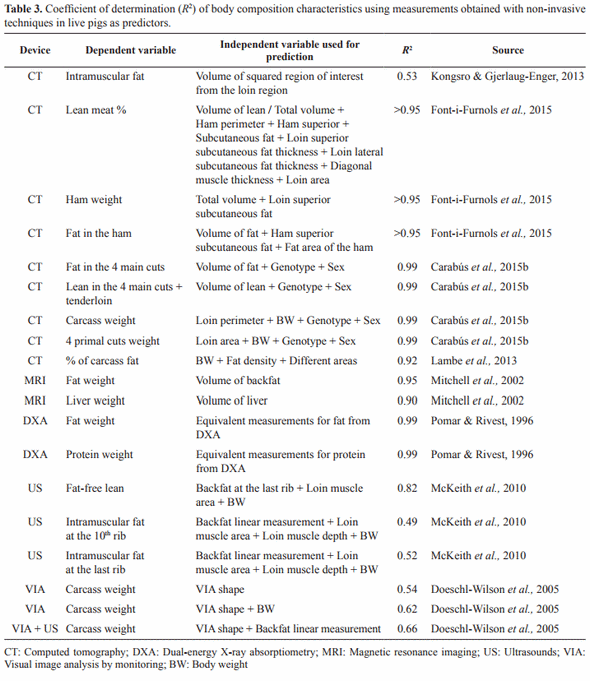
European interest in animal welfare and the prospect of legislation in several countries limiting the current practice of surgical castration without anaesthesia have encouraged the swine industry to reconsider its traditional approach to the control of boar taint and inves tigate alternatives (Gispert et al., 2010). One alternative is the immunocastration vaccine, and thus the immunocastrated male must be considered as another sexual condition to be studied. Regarding immunocastration, Carabús et al. (2015b) used CT to evaluate growing pigs of different sexes, including females, entire males, castrated males and immunocastrated males, and different live weights. Females exhibited significantly greater loin width than entire males, immunocastrated males and castrated males (211.7 mm vs. 209.63 mm vs. 203.2 mm and 201.7 mm). Castrated males presented greater subcutaneous fat of the loin and ham compared to the others sexes. Castrated males and entire males exhibited different subcutaneous lateral ham fat growth rates, whereas the growth rates of females were similar to those of castrated and immunocastrated males. Immunocastrated males were significantly leaner than castrated males.
Nutrition: Evaluation of the effect of diet by image analysis
Meat composition, growth rate and feed conversion are directly related to dietary composition. Lambe et al. (2013) studied the effects of feeding pig diets with different protein and amino acid levels on compositional changes during the growing-finishing period (40-115 kg) using CT scanning (at 60, 85 and 115 kg live weight). Different factors such as genotype, sex or feeding regime are typically studied together to optimise the use and potential of these non-invasive technologies. Kusec et al. (2007) used MRI to study the effect of the MHS (malignant hyperthermia syndrome) gene on intensive and restrictive feeding and observed significantly higher feed intake, daily gain and feed conversion ratio in pigs maintained under intensive feeding conditions compared with restrictive feeding regime. The growth of muscle tissue in pigs was not influenced by the feeding regime. The intensive feeding was designed to ensure optimal or possibly enhanced muscle growth capacity of hybrid pigs, but the study indicated that the restricted feeding regime supported the muscle growth just as effectively, which is very valuable information for nutrition companies. Noninvasive technologies have been used more often as a tool in live pigs for breeding and selection applications than for the study of diet itself. Some of these studies are presented in Table 4.
The application of non-invasive techniques in farming pigs is useful and adequate to study animal growth, in other words, to model their growth, because the same animal can be evaluated several times during its growth. Non-invasive techniques enable growth to be modelled depending on the genotype, sex, diet and many other factors. Modelling the growth of a certain group of pigs from birth to death and studying the same animals in each period is one of the best applications of modelling functions to predict BW, mature BW, fat weight, muscle weight, etc. at a certain future weight or to study the deposition speed of cuts and tissues, as suggested by the allometric function.
Several functions are available for the description of growth, including Brody’s function, the logistic function, the allometric function, the Gompertz function, the von Bertalanffy function, and the four-parameter Richards function, which combines aspects of all of the above growth functions into a single function. Growth functions are not the topic of the present paper but certainly exploit many of the advantages of noninvasive techniques.
In summary, US, VIA, MRI, DXA and CT are the most popular techniques for image acquisition in livestock animal evaluation. Whereas VIA is usually used to capture external attributes, MRI, US, CT, and DXA can be used to inspect internal structure. However, the accuracy of the images and the predictions obtained differ among techniques. Predictions with the highest resolution are generated from CT and MRI, followed by DXA, VIA and US. The CT presents superb results for the estimation of the amount of fat and lean mass, but its accuracy to predict IMF is not acceptable and requires improvement. The US is not sufficiently accurate in estimating the body composition of pigs if highly precise information is needed for research purposes. However, the information obtained can be used for performance testing in the field or to successfully classify pigs before slaughter. For livestock animals, portable devices must also be considered.
Moreover, the time required for image acquisition differs greatly among devices, with MRI the slowest. Combining a less-expensive device such US or VIA as a first selector with a second device such as CT or MRI to obtain 3D images in the selected animals could minimise the sample studied and, consequently, reduce the cost (Scholz & Baulain, 2009). Moreover, according to Scholz et al. (2015), the combination of phenotypic data obtained from non-invasive techniques with genome data could provide deeper information and knowledge of the growth and body composition of farm animals (Aasmundstad et al., 2013).
As conclusions, image analysis has applications in the livestock field. However, it is important to find, for each trait of interest the most appropriate technology in terms of accuracy, cost, efficiency and other requirements. Some devices are expensive, and to satisfy the demand for cost-effective techniques, inexpensive multipurpose image processing systems that yield higher-accuracy predictions must be developed. Portable or mobile devices make technology feasible for farming conditions and easier to obtain income by renting the device, particularly if the device is expensive. Improving the processing speed of the scan or image analysis by technicians and integrating specific image processing algorithms would improve the value of the technology. Cheaper and faster solutions have enabled image processing in live animals to assume and maintain an important role.
Acknowledgements
The authors would like to thank Armin M. Scholz from Ludwig Maximilian University of Munich for the photo and image provided.
Citation: Carabús, C.; Gispert, M.; Font-i-Furnols, M. (2016). Imaging technologies to study the composition of live pigs: A review. Spanish Journal of Agricultural Research, Volume 14, Issue 3, e06R01. http://dx.doi.org/10.5424/sjar/2016143-8439
Received: 10 Aug 2015. Accepted: 30 Jun 2016 Copyright © 2016 INIA. This is an open access article distributed under the terms of the Creative Commons Attribution-Non Commercial (by-nc) Spain 3.0 Licence, which permits unrestricted use, distribution, and reproduction in any medium, provided the original work is properly cited.
Funding: Instituto Nacional de Investigación y Tecnología Agraria y Alimentaria (INIA) (Project No. RTA2010-00014-00-00 and scholarship to Anna Carabús).
Competing interests: The authors have declared that no competing interests exist.
Correspondence should be addressed to Maria Font-i-Furnols: maria.font@irta.cat
References
Aasmundstad T, Kongsro J, Wetten M, Dolvik NI, Vangen O, 2013. Osteochondrosis in pigs diagnosed with computed tomography: heritabilities and genetic correlations to weight gain in specific age intervals. Animal 7(10): 1576-1582. http://dx.doi.org/10.1017/S1751731113001158.
Aasmundstad T, Grindflek E, Nordbø Ø, Kongsro J, Vangen O, 2014. Osteochondrosis in Duroc pigs scored by computed tomography; heritabilities based on genomic and pedigree relationship matrices. Proc 10th World Congress of Genetics Applied to Livest. Prod., Vancouver, Canada.
Afonso JJM, 1992. Factors affecting growth and body composition in sheep. PhD, University of New England, Armidale, New Zealand. Allen P, Finnerty N, 2000. Objective beef carcass classification - A report of a trial of three VIA classification systems. The National Food Centre, Dublin.
Bahelka I, Oravcová M, Peškovicová D, Tomka J, Hanusová E, Lahucký R, Demo P, 2009. Comparison of accuracy of intramuscular fat prediction in live pigs using five different ultrasound intensity levels. Animal 3: 1205-1211. http://dx.doi.org/10.1017/S1751731109004480.
Bardera A, Mart?nez R, Boada I, Font-i-Furnols M, Gispert M, 2012. VisualPork towards the simulation of a virtual butcher. Proc. 1st FAIM Conf., Dublin, Ireland. Baulain U, 1997. Magnetic resonance imaging for the in vivo determination of body composition in animal science. Comput Electron Agr 17: 189-203. http://dx.doi. org/10.1016/S0168-1699(96)01304-X.
Block J, 2014. Your guide to medical imaging equipment. http://info.blockimaging.com/bid/93368/GE-CT-ScannerCost-Price-Info [March 10th 2016]. Brethour JR, 1992. The repeatability and accuracy of ultrasound in measuring backfat of cattle. J Anim Sci 70(4): 1039-1044. Brunton JA, Bayley HS, Atkinson SA, 1993.Validation and application of dual energy X-ray absorptiometry to measure bone mass and body composition in small infants. Am J Clin Nutr 58: 839-845.
Cann CE, 1988. Quantitative CT for determination of bone mineral density: a review. Radiology 166(2): 509-522. http://dx.doi.org/10.1148/radiology.166.2.3275985.
Carabús A, Gispert M, Rodriguez P, Brun,A, Francàs C, Soler J, Font-i-Furnols M, 2011. Differences in body composition between pigs crossbreds of 30 kg measured in vivo by computed tomography. Book of Abstracts, 62 Ann Meeti Eur Fed Anim Sci 17: 104.
Carabús A, Gispert M, Brun A, Rodríguez P, Font-i-Furnols M, 2015a. In vivo computed tomography evaluation of the composition of the carcass and various cuts of growing pigs of three commercial crossbreeds. Livest Prod Sci 170: 181- 192. http://dx.doi.org/10.1016/j.livsci.2014.10.005.
Carabús A, Sainz RD, Oltjen JW, Gispert M, Font-i-Furnols M, 2015b. Predicting fat, lean and the weight of primal cuts of pigs of different genotypes and sexes using computed tomography. J Anim Sci 93(3): 1388-1397. http:// dx.doi.org/10.2527/jas.2014-8697.
Chang J, Jung J, Lee H, Chang D, Yoon J, Choi M, 2011. Computed tomographic evaluation of abdominal fat in minipigs. J Vet Sci 12: 91-94. http://dx.doi.org/10.4142/ jvs.2011.12.1.91.
Chmiel M, Lowinski M, Dasiewizc K, 2011. Application of computer vision systems for estimation of fat content in poultry meat. Food Control 22: 1424-1427. http://dx.doi. org/10.1016/j.foodcont.2011.03.002.
Craigie CR, Navajas EA, Purchas RW, Maltin CA, Bünger L, Hoskin SO, Ross DW, Morris ST, Roehe R, 2012. A review of the development and use of video image analysis (VIA) for beef carcass evaluation as an alternative to the current EUROP system and other subjective systems. Meat Sci 92: 307-318. http://dx.doi.org/10.1016/j.meatsci.2012.05.028.
Cross HR, Gilliland DA, Durland PR, Seideman S, 1983. Beef carcass evaluation by use of a video image analysis system. J Anim Sci 57: 908-917.
Daumas G, Monziols M, 2011. An accurate and simple computed tomography approach for measuring the lean meat percentage of pig cuts. 57th Int Cong Meat Sci Technol, 7-12 August 2011, Ghent-Belgium.
Doeschl-Wilson AB, Green DM, Fisher AV, Carroll SM, Schofield CP, Whittemore CT, 2005. The relationship between body dimensions of living pigs and their carcass composition. Meat Sci 70: 229-240. http://dx.doi. org/10.1016/j.meatsci.2005.01.010.
Engel B, Lambooij E, Buist WG, Vereijken P, 2012. Lean meat prediction with HGP, CGM and CSB-Image-Meater, with prediction accuracy evaluated for different proportions of gilts, boars and castrated boars in the pig popula-
tion. Meat Sci 90: 338-344. http://dx.doi.org/10.1016/j. meatsci.2011.07.020. Ferell CL, Cornelius CG, 1984. Estimation of body composition of pigs. J Anim Sci 58: 903-912.
Fisher AV, Green DM, Whittemore CT, Wood JD, Schofield CP, 2003. Growth of carcass components and its relation with conformation in pigs of three types. Meat Sci 65: 639-650. http://dx.doi.org/10.1016/S0309-1740(02)00266-8.
Font i Furnols M, Gispert M, 2009. Comparison of different devices for predicting the lean meat percentage of pig carcasses. Meat Sci 83: 443-446. http://dx.doi.org/10.1016/j. meatsci.2009.06.018.
Font-i-Furnols M, Carabús A, Pomar C, Gispert M, 2015. Estimation of carcass and cuts composition from computed tomography images of growing live pigs of different genotypes. Animal 9: 166-178. http://dx.doi. org/10.1017/S1751731114002237.
Fortin A, Wood JD, Whelehan OP, 1987. Breed and sex effects on the development, distribution of muscle, fat and bone, and the partition of fat in pigs. J Agric Sci 108: 141-153. http://dx.doi.org/10.1017/S0021859600064212.
Fortin A, Tong AKW, Robertson WM, Zawadski, SM, Landry SJ, Robinson DJ, Liu T, Mockford RJ, 2003. A novel approach to grading pork carcasses: computer vision and ultrasound. Meat Sci 64: 451-462. http://dx.doi. org/10.1016/S0309-1740(02)00104-3.
Giles LR, Eamens GJ, Arthur PF, Barchia IM, James KJ, Taylor RD, 2008. Differential growth and development of pigs as assessed by X-ray computed tomography. J Anim Sci 87: 1648-1658. http://dx.doi.org/10.2527/ jas.2008-1437.
Gispert M, Font i Furnols M, Gil M, Velarde A, Diestre A, Carrión D, Sosnicki AA, Plastow GS, 2007. Relationships between carcass quality parameters and genetic types. Meat Sci 77: 397-404. http://dx.doi.org/10.1016/j.meatsci.2007.04.006.
Gispert M, Oliver MA, Velarde A, Suárez P, Pérez J, Fonti-Furnols M, 2010. Carcass and meat quality characteristics of immunocastrated male, surgically castrated male, entire male and female pigs. Meat Sci 85: 664-670. http:// dx.doi.org/10.1016/j.meatsci.2010.03.021.
Gjerlaug-Enger E, Kongsro J, Ødega J, Aass L, Vangen O, 2012. Genetic parameters between slaughter pig efficiency and growth rate of different body tissues estimated by computed tomography in live boars of Landrace and Duroc. Animal 6: 9-18. http://dx.doi.org/10.1017/ S1751731111001455.
Gotfredsen A, Borg J, Christiansen C, Mazess RB, 1984. Total body bone mineral in vivo by dual photon absorptiometry. I. Measurement procedures. Clin Physiol 4: 343-355. http://dx.doi.org/10.1111/j.1475-097X.1984. tb00809.x.
Humbert L, Whitmarsh T, Craene MD, Del Río Barquero LM, Frangi AF, 2012. Technical note: comparison between single and multiview simulated DXA configurations for reconstructing the 3D shape and bone mineral density distribution of the proximal femur. Med Phys 39(8): 5272- 5276. http://dx.doi.org/10.1118/1.4736540.
Kistorp CN, Svendsen OL, 1998. Body composition results by DXA differ with manufacturer, instrument generation and software version. Appl Radiat Isot 49: 515-516. http:// dx.doi.org/10.1016/S0969-8043(97)00063-8.
Knox R, 2008. Using real-time ultrasound for pregnancy diagnosis in swine. FactSheet. Pork Information Gateway. North Carolina State University, Raleigh, NC, USA. Kohl G, 2005. The evolution and state-of-the-art principles of multislice computed tomography. Proc Am Thorac Soc Vol 2: 470-476. http://dx.doi.org/10.1513/pats.200508- 086DS.
Kolstad K, 2001. Fat deposition and distribution measured by computer tomography in three genetic groups of pigs. Livest Prod Sci 67: 281-292. http://dx.doi.org/10.1016/ S0301-6226(00)00195-0. Kolstad K, Jopson NB, Vangen O, 1996. Breed and sex differences in fat distribution and mobilization in growing pigs fed at maintenance. Livest Prod Sci 47: 33-41. http:// dx.doi.org/10.1016/S0301-6226(96)01001-9.
Kongsro J, 2013. Development of a computer vision system to monitor pig locomotion. Open J Anim Sci 3: 254-260. http://dx.doi.org/10.4236/ojas.2013.33038.
Kongsro J, Røe M, Kvaal K, Aastveit AH, Egelandsdal B, 2009. Prediction of fat, muscle and value in Norwegian lamb carcasses using EUROP classification, carcass shape and length measurements, visible light reflectance and computer tomography (CT). Meat Sci 81: 102-107. http:// dx.doi.org/10.1016/j.meatsci.2008.07.004.
Kongsro J, Gjerlaug-Enger E, 2013. In vivo prediction of intramuscular fat in pigs using computed tomography. J Anim Sci 3: 321-325.
Kremer PV, Fernández-Fígares I, Förster M, Scholz AM, 2012. In vivo body composition in autochthonous and conventional pig breeding groups by dual-energy X-ray absorptiometry and magnetic resonance imaging under special consideration of Cerdo Ibérico. Animal 6: 2041- 2047. http://dx.doi.org/10.1017/S1751731112001267.
Kremer PV, Förster M, Scholz AM, 2013. Use of magnetic resonance imaging to predict the body composition of pigs in vivo. Animal 7: 879-884. http://dx.doi.org/10.1017/ S1751731112002340.
Kusec G, Baulain U, Kallweit E, Glodek P, 2007. Influence of MHS genotype and feeding regime on allometric and temporal growth of pigs assessed by magnetic resonance imaging. Livest Sci 110: 89-100. http://dx.doi.org/10.1016/j. livsci.2006.10.007.
Lakshmanan S, Koch T, Brand S, Männicke N, Wicke M, Mörlein D, Raum K, 2012. Prediction of the intramuscular fat content in loin muscle of pig carcasses by quantitative time-resolved ultrasound. Meat Sci 90: 216-225. http://dx.doi.org/10.1016/j.meatsci.2011.07.004.
Lambe NR, Wood JD, McLean KA, Walling GA, Whitney H, Jagger S, Fullarton P, Bayntun J, Bünger L, 2013. Effects of low protein diets on pigs with a lean genotype 2. Compositional traits measured with computed tomography (CT). Meat Sci 95: 129-136. http://dx.doi.org/10.1016/j. meatsci.2013.04.038.
Ley C, 2013. Pig genetics is all about sustainable profitability. Pig Int 43: 4-7.
Li J, Tan J, Martz FA, Heymann H, 1999. Image texture features as indicators of beef tenderness. Meat Sci 53: 17-22. http://dx.doi.org/10.1016/S0309-1740(99)00031-5.
Luiting P, Kolstad K, Enting H, Vangen O, 1995. Pig breed comparison for body composition at maintenance: analysis of computerized tomography data by mixture distributions. Livest Prod Sci 43: 225-234. http://dx.doi. org/10.1016/0301-6226(95)00044-L.
Maignel L, Daigle JP, Gariépy C, Wilson D, Sullivan D, 2010. Prediction of intramuscular fat of live pigs using ultrasound technology and potential use in selection. 9th World Congress of Genetics Applied to Livestock Production, Leipzig, Germany, 1st-6th August.
Margeta V, Kralik G, Kušec H, Baulain U, 2007. Lean and fat development in the whole body and hams of hybrid pigs studied by magnetic resonance tomography. Czech J Anim Sci 52(5): 130-137.
McKeith RO, Lowe BK, Safko JA, Pitzer RM, Segers J R, Stelzleni AM, Azain MJ, Pringle TD, 2010. Prediction of carcass ad fat-free lean using ultrasound measures at the last and tenth rib in swine. 43rd Meeting of Midwest Am Soc Anim Sci, Des Moines, IA, 15-17
March. Meek PD, 2012. Refining and improving the use of camera trap technology for wildlife management and research in Australia and New Zealand. The Winston Churchill Memorial Trust of Australia, Canberra.
Mitchell AD, Conway JM, Scholz AM, 1996. Incremental changes in total and regional body composition of growing pigs measured by dual energy X-ray absorptiometry. Growth Dev Aging 60: 95-105.
Mitchell AD, Scholz AM, Conway JM, 1998. Body composition analysis of pigs from 5 to 97 kg by dual energy X-ray absorptiometry. Appl Radiat Isot 49: 521-523. http://dx.doi.org/10.1016/S0969-8043(97)00066-3.
Mitchell AD, Scholz AM, Wang PC, Song H, 2001. Body composition analysis of the pig by magnetic resonance imaging. J Anim Sci 79: 1800-1813.
Mitchell AD, Scholz A, Pursel V, 2002. Prediction of the in vivo body composition of pigs based on cross-sectional region analysis of dual energy X-Ray absorptiometry (DXA) scans. Arch Tierz, Dummerstorf 45: 535-545.
Mohrmann M, Roehe R, Susenbeth A, Baulain U, Knap PW, 2006. Association between body composition of growing pigs determined by magnetic resonance imaging, deuterium dilution technique, and chemical analysis. Meat Sci 72: 518–531. http://dx.doi.org/10.1016/j.meatsci.2005.08.020.
Monziols M, Collewet G, Bonneau M, Mariette F, Davenel A, Kouba M, 2006. Quantification of muscle, subcutaneous fat and intermuscular fat in pig carcasses and cuts by magnetic resonance imaging. Meat Sci 72: 146-154. http:// dx.doi.org/10.1016/j.meatsci.2005.06.018.
Mörlein D, Rosner F, Brand S, Jenderka KV, Wicke M, 2005. Non-destructive estimation of the intramuscular fat content of the longissimus muscle of pigs by means of spectral analysis of ultrasound echo signals. Meat Sci 69: 187-199. http://dx.doi.org/10.1016/j.meatsci.2004.06.011.
Newcom DW, Baas TJ, Lampe J, 2002. Prediction of intramuscular fat percentage in live swine using real-time ultrasound. J Anim Sci 80: 3046-3052.
Peppler WW, Mazess RB, 1981. Total body bone mineral and lean body mass by dual-photo absorptiometry. Theory and measurement procedure. Calcif Tissue Int 3: 353- 359. http://dx.doi.org/10.1007/BF02409455.
Pomar C, Rivest, J, 1996. The effect of body composition and data analysis on the estimation of body composition of pigs by dual energy X-ray absortiometry (DEXA). Ann Meet Can Soc Anim Sci, Lethbridge, Alberta, 7-11 July.
Romvári R, Szabó A, Kárpáti J, Kovách G, Bázár G, Horn P, 2005. Measurement of belly composition variability in pigs by in vivo computed tomographic scanning. Acta Vet Hung 53(2): 153-162. http://dx.doi.org/10.1556/AVet.53.2005.2.1.
Rosset A, Spadola L, Ratib O, 2004. OsiriX: An open-source software for navigating in multidimensional DICOM images. J Digit Imaging 17: 205-216. http://dx.doi.org/10.1007/ s10278-004-1014-6.
Rozeboom KJ, Thomas MG, Hillman L, Chanetsa F, Lipsey RJ, Keisler DH, 1998. Use of dual-energy X-ray absorptiometry in estimating the carcass composition of wether lambs. J Anim Sci 76 (Suppl. 1): 149.
Schofield CP, 1990. Evaluation of image analysis as a means of estimating the weight of pigs. Agr Eng Res 47: 287- 296. http://dx.doi.org/10.1016/0021-8634(90)80048-Y.
Schofield CP, 2007. Portable image-based pig weight monitoring systems. Eur Conf Precis Livestock Farm, Skiathos (Greece).
Scholz AM, Baulain U, 2009. Methods of determination of body composition in living animals. Zuechtungskunde 81: 86-96.
Scholz AM, Mitchell AD, 2010. Body composition: indirect measurement. Encyclopedia of Animal Science, 2nd Ed, 1: 152-156.
Scholz AM, Nüske S, Förster M, 2003. Body composition and bone mineralization in calves of different genetic origin by using dual energy X-ray absorptiometry. Acta Diabetologica 40 (Suppl. 1): S91-S94. http://dx.doi. org/10.1007/s00592-003-0037-7.
Scholz AM, Bunger L, Kongsro J, Baulain U, Mitchell AD, 2015. Non-invasive methods for the determination of body and carcass composition in livestock: dual-energy X-ray absorptiometry, computed tomography, magnetic resonance imaging and ultrasound: invited review. Animal 9(7): 1250- 1264. http://dx.doi.org/10.1017/S1751731115000336.
Schreiweis, MA, Orban J, Ledur MC, Moody DE, Hester PY, 2005. Validation of dual-energy X-Ray absorptiometry in
live white leghorns. Poult Sci 84: 91-99. http://dx.doi. org/10.1093/ps/84.1.91.
Silva SR, Alfonso JJ, Santos VA, Monteiro A, Guedes CM, Azevedo JMT, Dias-da-Silva A, 2006. In vivo estimation of sheep carcass composition using real-time ultrasound with two probes of 5 and 7.5 MHz and image analysis. J Anim Sci 84 (12): 3433-3439. http://dx.doi.org/10.2527/ jas.2006-154.
Sun X, Chen KJ, Maddock-Carlin KR, Anderson VL, Lepper AN, Schwartz CA, Keller WL, Ilse BR, Magolski JD, Berg EP, 2012. Predicting beef tenderness using color and multispectral image texture features. Meat Sci 92: 386- 393. http://dx.doi.org/10.1016/j.meatsci.2012.04.030.
Suster D, Leury BJ, Ostrowska E, Butler KL, Kerton DJ, Wark JD, Dunshea FR, 2003. Accuracy of dual energy X-ray absorptiometry (DXA), weight and P2 back fat to predict whole body and carcass composition in pigs within and across experiments. Livest Prod Sci 84: 231- 242. http://dx.doi.org/10.1016/S0301-6226(03)00077-0.
Swennen Q, Janssens GPJ, Geers R, Decuypere E, Buyse J, 2004. Validation of dual-energy X-Ray absorptiometry for determining in vivo body composition of chickens. Poult Sci 83: 1348-1357. http://dx.doi.org/10.1093/ps/83.8.1348.
Szabó CS, 2001. Determination of protein and fat content in growing and fattening pigs by the means of computed tomograph, using different lysine to digestible energy ratios in the diet. Doctoral (PhD) dissertation, University of Kaposvár, Hungary.
Szabó CS, Babinszky L, Verstegen MWA, Vangen O, Jansman AJM, Kanis E, 1999. The application of digital imaging techniques in the in vivo estimation of the body composition of pigs: a review. Livest Prod Sci 60: 1-11. http://dx.doi.org/10.1016/S0301-6226(99)00050-0.
Tothill P, Avenell A, Love J, Reid DM, 1994. Comparisons between Hologic, Lunar and Norland dual energy X-ray absorptiometers and other techniques used for whole-body soft tissue measurements. Eur J Clin Nutr 48: 781-794.
Walpert B, 2000. With ancillary services, you can do well by doing good. In: American College of Physicians Observer, October, 8.2.2011. http://www.acpinternist.org.
White RP, Schofield CP, Green DM, Parsons DJ, Whittemore CT, 2004. The effectiveness of a visual image analysis (VIA) system for monitoring the performance of growing/ finishing pigs. Anim Sci 78: 409-418.