Introduction
The transcriptome is the momentary sum of all DNA transcribed in a cell. Classically, the transcriptome included the measurement of each of the ribosomal RNA, messenger RNA (mRNA), and transfer RNA. These include a relatively small portion of the whole genome.1 The rest of the DNA was considered, until recently, not to be transcribed.1 The accumulation of data in the last decade or so has provided vast evidence that most, if not all, of the DNA is actually transcribed.1 The transcribed DNA that does not belong to the three types of RNA listed above is termed noncoding RNA. 2 There is an increasing number of different noncoding RNAs. Among these, the first to be discovered and the first to have a clearly defined function are the micro-RNAs (miRNA).3
The miRNA are short RNAs (average 22 nucleotides) involved in posttranscriptional gene silencing.3 They are actively transcribed by the cells, and the regulation of their transcription is an active area of research. The miRNA are transcribed as pre-miRNA and undergo a multistep maturation process, providing a fully mature (and active) miRNA.3 The gene-silencing activity of the miRNA is based on complementarity with specific transcribed mRNA. If the complementarity is perfect, the miRNA binds to the mRNA, forming a double strand that induces faster mRNA degradation. If the complementarity is not perfect, the translation is inhibited, but the speed of mRNA degradation is not affected.4
The regulation of the expression of genes (ie, mRNA coding for proteins) is a complex and multifactorial phenomenon, including posttranscriptional regulations.5–7 The first and long-term level of regulation is carried out by epigenetic factors determining which part of the DNA is available for transcription, and the second and medium-length level of regulation is determined by transcriptional regulators or factors. The transcription regulators are proteins with the main purpose of regulating the expression of genes. This is done by interacting directly with short DNA sequences, termed response elements, in general present in regions upstream of the transcription start site of the gene.8 Therefore, the expression of genes in the short/medium term is determined by the abundance of the transcription factors (TFs) and by their activity. The latter is determined by compounds that directly or indirectly interact with the TFs, ie, upstream regulators. The concept of upstream regulators can include a relatively large diversity of compounds, ranging from lipids to proteins.
By knowing the change in the expression of downstream genes using transcriptomic analysis, it is possible, through bioinformatics means, to infer the upstream regulators responsible for the observed changes. The inference of upstream regulators is an important information to reveal the causes of the observed changes at the transcriptomic level9,10; however, the analysis of the transcriptome can only measure the abundance of mRNA available in the instant when the tissue or cells were harvested. The mRNA available for translation is not equal to the mRNA expressed by the DNA due to the presence of active posttranscriptional regulation. Therefore, the inference of upstream regulators is biased by posttranscriptional regulation. One of the most important posttranscriptional regulatory factors is miRNA. For this reason, our objective was to provide a more realistic analysis of upstream regulators by accounting for the effect of miRNA via a bioinformatics approach.
Materials and Methods
Ethics statement. All animal use was carried out in strict accordance with the Directions for Caring of Experimental Animals from the Institute of Animal Science, Chinese Academy of Agricultural Sciences. All efforts were made to minimize suffering.
Tissue sample preparation. Both liver and mammary gland tissue samples were obtained by biopsy from 10 primiparous lactating Holstein cows (body weight, 558 ± 10 kg; days in milk, 136 ± 37 days; daily milk yield, 21.1 ± 2.3 kg) as previously described.11,12 Briefly, throughout the experiment, cows were housed in a tie stall barn, and diet was formulated to meet the requirements according to the National Research Council.13 The cows were fed two different diets (n = 5/group) as previously described.14 The diets were prepared daily and fed ad libitum as total mixed ration. The diets were supplied twice a day at 07:00 and 19:00 hours in an equal amount that allowed for 10% residuals. Cows were milked twice daily at 07:00 and 19:00 hours and had free access to water. The liver and mammary biopsies were performed simultaneously (ie, within 40 minutes) at approximately 07:00 hours (post-AM milking).
Prior to the biopsies, the cows received a small dose of xylazine (0.05 mg/kg body weight (BW)) before applying a local anesthetic. Prior to the incision, 3–4 mL of lidocaine hydrochloride (2% solution) was injected subcutaneously as the local anesthetic. For the mammary biopsy, an incision using a sterilized scalpel blade was performed on the midsection of the left rear quarter. The parenchyma tissue was removed, and the mammary epithelium was exposed. Once the parenchyma was visible, a biopsy was performed using Bard® Magnum® Reusable Core Biopsy Instrument MN1213 (Bard Peripheral Vascular). Immediately after removal of the biopsy instrument, we applied pressure to stop bleeding using sterile gauze. Approximately 400 mg of mammary tissue was obtained from the biopsy. The liver tissue (approximately 300 mg) was collected via puncture biopsy. The large hepatic blood vessels were identified using a 3.5 MHz ultrasound probe and used to identify the site for biopsy. A 1.5-cm incision using a sterile scalpel blade was done between the 11th and 12th ribs on the right side of the cow. Following the skin incision, proper pressure using the sterile gauze was applied to the wound until visual signs of bleeding were absent. The biopsy of the liver was performed using a Rhone Merieux liver biopsy needle (9.5 mm diameter; Rhone Merieux). The tissue obtained through biopsy was immediately washed with phosphate buffer saline (PRS) buffer prepared with RNAase-free water and hydrated and stored in liquid nitrogen until RNA extraction. For both biopsies, the skin incision was closed with four or five Michel clips (11 mm; Henry Schein). The incision site was sprayed with topical antiseptic (10% povidoneiodine ointment; Taro Pharmaceutical Industries). Health was monitored postsurgery by recording rectal temperature, milk yield, and feed intake daily for seven days. Surgical clips were removed seven days postbiopsy.
RNA extraction and microarray. Total RNA was extracted using the TRIzol reagent (Cat# 74106; Life technologies) according to the manufacturer’s protocol. The total RNA was purified using the RNeasy Mini Kit (Cat# 74106; QIAGEN) and the RNase-Free DNase Set (Cat# 79254; QIAGEN). The concentration was measured by NanoDrop 1000 (Thermo Fisher Scientific). The OD260/OD280 values were $1.9. Integrity of the purified total RNA was assessed using 2100 Bioanalyzer (Agilent Technologies) and the RNA 6000 Nano Kit (Agilent Technologies). The RNA integrity number values were considered as satisfactory when the value was $8.0.
The mRNA transcriptomic analysis was performed using a 4 × 44 K bovine microarray chip (design ID: 023647; Agilent Technologies). Total RNA was amplified and labeled using the Low Input Quick Amp Labeling Kit, One-Color (Cat# 5190–2305; Agilent Technologies) according to the manufacturer’s instructions. Each slide was hybridized with 1.65 µg of Cy3-labeled cRNA using the Gene Expression Hybridization Kit (Cat# 5188–5242; Agilent Technologies) in a hybridization oven (Agilent Technologies). After 17 hours hybridization, slides were washed in staining dishes (Thermo Fisher) using the Gene Expression Wash Buffer Kit (Cat# 5188–5327; Agilent Technologies).
Slides were scanned using an Agilent Microarray Scanner (Agilent Technologies) with default settings (ie, dye channel: green, scan resolution = 5 µm, photomultiplier tubes (PMT), 100%, 10%, 16 bit). Data were acquired using the Feature Extraction Software 10.7 (Agilent Technologies). The microarray data presented in this article have been deposited at NCBI’s Gene Expression Omnibus (accession number GSE73980).
The miRNA profile was performed using the Agilent 8 × 15 K miRNA array V17.0 (Agilent Technologies) customized for bovine miRNA based on the miRBase (17.0). One-hundred nanograms of total RNA and a Labeling Spike-In solution (MicroRNA Spike-In Kit; Agilent Technologies) underwent a phosphatase treatment with calf intestinal alkaline phosphatase, and the dephosphorylated RNA was subjected to denaturing and labeling reactions with dimethyl sulfoxide (DMSO) and cyanine 3-pCp (Complete Labeling and Hybridization Kit; Agilent Technologies). Subsequently, the labeled RNA was desalted with spin columns (MicroBioSpin 6 Columns; Agilent Technologies), and the desalted labeled RNA was then hybridized to the microarray. After 20 hours of hybridization, slides were washed in staining dishes using the Gene Expression Wash Buffer Kit following the manufacturer’s instructions.
Slides were scanned using an Agilent Microarray Scanner with default settings. The presence or absence of a given miRNA was determined according to the difference between signal of the probe and the background. The Feature Extraction Software 10.7 was used to obtain the raw data.
JMP Genomics analysis. Agilent miRNA microarray data were analyzed using the JMP Genomics software (SAS Institute). Total gene signal, as calculated by the Agilent software, provides the mean of background-corrected expression across all probes for a given miRNA. A table with the total gene signal for each miRNA across all microarray runs was compiled for use in the JMP Genomics basic miRNA workflow. Shifted (+1) log2 transformation of the data was used for both quality control analysis and before normalization. Transformed expression values were normalized by standard deviation (STD; mean of zero, variance of one), interquartile range (IQR), or kernel density quantile (KDQ ) methods. We observed best data distribution using the KDQ. The KDQ-normalized set was therefore used for the analysis of variance.
We originally analyzed the miRNA dataset for the tissue, diet, and tissue × diet effect, but we found only a few miRNA affected by diet or tissue × diet with a false discovery rate (FDR) of ,0.05, and none were a large change. In comparison, we found a large amount of miRNA significantly affected by tissue. Therefore, we continued our analysis considering only miRNA different between liver and mammary tissue.
Significant changes in miRNA expression were tested for tissue models in a pairwise fashion, adjusting for random effects due to the subject animal, and with a multiple testing method of FDR # 0.05. After JMP Genomics analysis, one of the samples (L8496) was an outlier; therefore, the analysis was rerun with the L8496 sample removed from the data set. Agilent mRNA microarray data were also analyzed with JMP Genomics as stated earlier, with three exceptions. The basic expression workflow was used, only the STD normalization method was run, and none of the samples were excluded. The analysis was run independently on the original dataset and on miRNA-corrected datasets (described below).
miRNA target prediction databases. Four separate target prediction databases were queried with the significant miRNA determined using the JMP Genomics analysis. TargetScan15,16 was the primary database used as it housed Bos taurus-specific information with target scores predicted as the probability of conserved targeting (PCT) or the probability that the listed miRNA targeted and affected the listed mRNA. These values were used to calculate the relative effect of each miRNA on its predicted target mRNA. If a given miRNA:mRNA pair did not have a high PCT value, then the calculated effect of the miRNA was reduced for the overall mRNA correction calculation (described further below). Only miRNA and mRNAs listed in the TargetScan database were used for the correction calculation, therefore contributing to it being a conservative correction as not all miRNA:mRNA effects were able to be estimated.
The miRanda (http://microrna.org), DIANA17 version 3.0 (http://diana.cslab.ece.ntua.gr/microT/), and PicTar (http:// pictar.mdc-berlin.de/cgi-bin/PicTar_vertebrate.cgi) were also queried to make lists for comparison analysis of coverage to the TargetScan list. The TargetScan database lists miRNA– mRNA pairs with a wide range of target scores, including nearzero values. For this analysis, we used the absolute value of the target score. For our dataset, the miRNA–mRNA pair scores ranged between 0.0 and 1.3. When binned by 0.1, roughly 25% of the pair scores were greater than 0.3, with each lower bin containing another 25% of the pairs. We, therefore, broke the TargetScan data into four files: all data for the selected miRNA, the top 75%, 50%, or 25% scoring pairs. These lists were then reduced to nonduplicate mRNA targets and compared with nonduplicate mRNA target lists from the other three database source lists. When these lists were compared, 31.7% of the overall TargetScan-predicted mRNA targets were also predicted in the other three databases (92.1% in at least one other database), with 32.3% (92.4%), 33.9% (92.9%), and 36.4% (93.4%) shared in the top 75%, 50%, and 25% scoring pair lists, respectively. It appeared that the top 50% scoring pairs captured the majority of the fully overlapping data, but all four binned datasets were used for further analysis.
miRNA correction of mRNA expression. To calculate a better estimation of gene transcription levels and therefore to estimate which TFs are acting on the mRNA, we combined the calculated levels of mRNA and miRNA in combination with predicted target scores between miRNA:mRNA pairs. This is a rough estimation, as the levels of mRNA and miRNA were not accurately quantified and were only relative levels, and their ratio and the ratio of the impact of miRNA on the mRNA are unknown, which can change the overall effect.
In a typical animal cell, mRNA and miRNA concentrations can vary greatly, and miRNA levels are often higher than mRNA levels.18 In fact, one study shows that a large miRNA concentration is required to see a significant effect on mRNA, and only 30% of miRNA is significantly active.19 In one study by Ragan et al.18, the effects of various input miRNA and mRNA concentrations were tested, and they found that the absolute concentration of the miRNA had a greater effect on the mRNA than did the ratio between the two. In another study by Subkhankulova et al.20, the mRNA content of a single mammalian cell was analyzed. Over 85% of genes are expressed at less than 100 copies per cell, with an average of 10 copies per cell and a log–log-normal distribution. In a study by Liang et al.21 where the levels of miRNA in mammalian tissue were analyzed, they detected an average of approximately 500 copies per cell (ie, 5 × compared to the average copy per cell of mRNA20), which lends further support to the need for a higher miRNA concentration to have a posttranscriptional effect on mRNA. In the study by Ragan et al.18, where a set of known mRNA:miRNA pairs were assessed by an in silico approach, when the miRNA and mRNA were expressed at a level of 500 copies per cell, only 72% of the mRNAs were reduced by .30%. Using numbers from these experiments, we created a table of values to be used for linear regression analysis in an attempt to find a rough, conservative estimate for calculating the actual transcribed mRNA levels from the observed miRNA and mRNA levels (Table 1). The relationship between the concentration of miRNA and the fraction of significantly reduced (at least 30%) mRNA determined from their data was
Estimated_fraction_significant = 0.1096 × ln(miRNA nM)
The KDQ-normalized expression value for each miRNA or mRNA is reported by JMP Genomics in log2. For this analysis, we took the base-2 exponential of the JMP Genomics values to convert back to the normalized expression values. The normalized miRNA values were used as an estimate of molarity for the miRNA as they were comparable to the typical nanomolar range in a cell.18 Target scores were also accounted for in the analysis, and all miRNAs that were predicted to target a particular mRNA had their normalized value multiplied by their respective target score and summed together to arrive at an estimated fraction of significant gene reduction. For each sample, therefore, we took the normalized expression value for each miRNA targeting a particular mRNA, converted it out of the log2 scale (=2 JMP value), multiplied by the target score, and summed all miRNAs together for a final overall level of miRNA for each mRNA target. We compared the results obtained by considering the top 25%, 50%, and 75% or all predicted miRNA:mRNA pairs. This was then converted as above to an estimated fraction of significant gene reduction, and this was used to calculate the transcribed mRNA level as follows:
Transcribed_mRNA = Normalized_mRNA_observed/ (1-Est_frac_sig * 0.3)
This correction adds back additional mRNA to account for the estimated fraction that was significantly reduced, in this calculation 30% (ie, 0.3). We also estimated the transcribed mRNA levels using the 50%, 75%, or 83% mRNA reduction for comparison, as many of the mRNAs in the previous work were reduced much more than 30%. This correction method does not use the difference between samples for any corrective calculation but only uses the level of miRNA in a given sample to predict the transcribed level of mRNA before posttranscriptional regulation in that sample. The new mRNA levels were rerun through JMP Genomics and compared against the original dataset. The complete script for the analysis and the guideline are provided in Supplementary File 1.
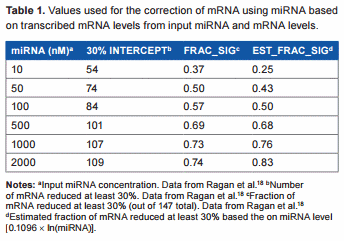
Analysis of upstream regulators by Ingenuity Pathway Analysis. In order to test the effect of the miRNA on the prediction of upstream regulators, especially TFs, the upstream regulator analysis was performed using the Ingenuity Pathway Analysis (IPA; QIAGEN). The whole dataset with the Entrez Gene ID, FDR, and expression ratio of the original transcriptomic dataset and the datasets corrected by miRNA were used. The annotated microarray was used as background in all analyses. The comparison analysis feature in IPA was used to visualize and download the results. The analysis of upstream regulators by IPA allows identification of the upstream regulators of differentially expressed genes using information in the Ingenuity® Knowledge Base. The analysis provides a predicted activation Z-score and an overlap P-value for each upstream regulator.
The activation Z-score is an estimate of the status of the upstream regulator using the level of gene expression of known target genes. Either a prediction of activation or inhibition (or no prediction) also accounts for the chance that random data generate significant predictions. Z-scores greater than 2 or smaller than −2 can be considered as significant.
The overlap P-value identifies transcriptional regulators that are able to explain the observed gene expression changes. The overlap P-value measures whether there is a statistically significant overlap between the dataset genes and the genes that are regulated by an upstream regulator. It is calculated using Fisher’s exact test, and the significance is generally attributed with P-values ,0.01.
Upstream TFs analysis using the Dynamic Impact Approach. The Dynamic Impact Approach (DIA) is a new bioinformatics approach for the analysis of transcriptomics data that substantially differs from the classical enrichment analysis tools.22 The main difference is that the DIA is not a statistical tool and uses the expression ratio and the statistical results (in this case obtained by JMP Genomics) to calculate the impact of any condition on several databases, including pathways. Different than the classical enrichment analysis is also the output. The DIA provides an absolute number of the impact and the direction of the impact (either induced or inhibited) of the condition(s) studied, allowing a quantitative comparison between results from different datasets. For this reason, it can allow for a more precise discovery of differences in upstream TFs due to correction by miRNA.
In order to have the DIA able to uncover the impact of upstream regulators, the human transcriptional regulatory interaction database (HTRIdb)23 was uploaded in the DIA, and the database was summarized using the TFClass, a classification of human transcriptional factors.24 The bovine orthologs of the human Entrez Gene IDs in the HTRIdb were obtained through bioDBnet.25 Data output from the JMP Genomics analyses were condensed for input into the DIA tool. The whole annotated Bovine (V2) Gene Expression Microarray 4 × 44 K was used as background. The selection criteria in DIA were FDR , 0.05.
Correlation analysis. Spearman’s correlation analysis was performed using the SigmaPlot software (version 11; Systat Software Inc.). The correlation was performed for expression ratio, FDR, and P-value between liver and mammary for all levels of miRNA corrections. In addition, a correlation between all comparisons of IPA and DIA outputs was performed.
Results and Discussion
Overall difference in miRNA expression between liver and mammary tissue in dairy cows. The statistical results of the 670 unique bovine miRNA detected in our analysis are reported in Supplementary File 2. Approximately 27% of all measured miRNAs were significantly different between the two tissues at an FDR , 0.05. Among these, 86 differentially expressed miRNAs were more expressed in liver vs. mammary (with a geometrical mean expression ratio of 2.2), and 101 differentially expressed miRNAs were more expressed in mammary vs. liver (with a geometrical mean expression ratio of 2.3; Supplementary File 2). Twenty differentially expressed miRNAs with the largest differences between the two tissues are reported in Table 2. The miRNA miR-122 had extremely large expression in liver vs. mammary. This is not surprising considering that this miRNA is liver specific in mouse (making up to 70% of liver miRNA) and human,26,27 and it is involved in the regulation of lipid metabolism28 and circadian rhythm29 in this organ. Also miR-192 and miR-194 had .70-fold higher expression in liver vs. mammary; miR-192 is among the most abundant miRNA in human liver,27 while miR-194 was proposed to be a marker of hepatic epithelial cells in mice.30 miR-205 was expressed .150-fold in the mammary tissue compared to liver (Table 2). This miRNA is associated with the mammary stem cells31 and the regulation of epithelial-to-mesenchymal transition32; however, miR-205 is not affected by lactation in bovine mammary,33 and a role in lactation has not been clearly defined. In addition, miR-10b is associated with the induction of metastatis in breast cancer,34 miR-141 controls the expression of STAT5 in bovine mammary cells,35 and miR-200a is associated with the formation of mammary epithelial cells.36 Several miR-200s and miR-10a and miR-10b are associated with normal regulation of human mammary cells.37 Overall, the comparison with the literature supports a consistency of our findings with what was previously known. Furthermore, the large difference in miRNA between the two tissues is consistent with the large difference in biological functions.
miRNA target prediction. Four methods were compared for miRNA target prediction, including TargetScan, miRanda, DIANA, and PicTar. TargetScan data were used as it had the best overlap between the different databases, and it had the best B. taurus-specific information as well as target scores predicted between the mRNA and miRNA pairs (Supplementary Fig. 1).
Effect of miRNA correction on mRNA dataset. The main aim of this work was to propose a method to correct for the posttranscriptional effects of miRNA on the measured mRNA expression and, thus, better estimate the real DNA transcription levels. This is important when considering the in silico analysis of upstream regulators using the transcriptomic datasets. The elimination of the miRNA effect can allow seeing a more real picture of the mRNA being expressed and can allow uncovering with higher precision the upstream transcription regulators partly responsible for the observed change on the transcriptome. The mRNA dataset before and after miRNA corrections is reported in Supplementary File 3.
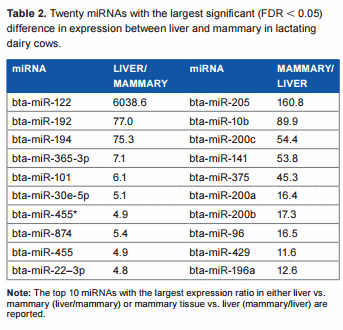
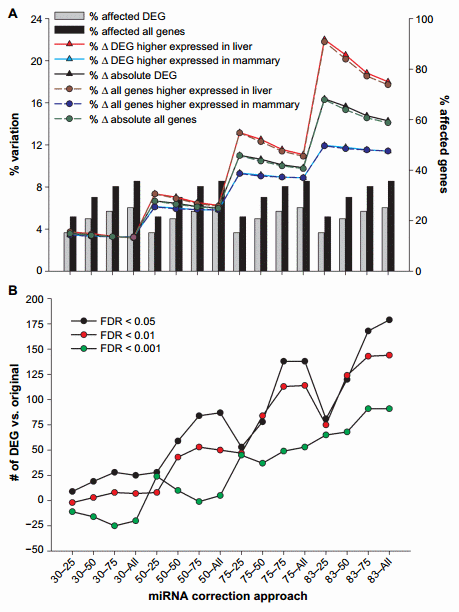
Figure 1. Effect of miRNA correction of mRNA expression ratio between liver and mammary tissue. Summarized are (A) the percentage of DEG between liver and mammary tissue or all genes affected by the miRNA correction compared to all measured genes, the percentage of DEG or all genes which expression ratio between liver and mammary tissue increased or decreased after correction, and the percentage of absolute change in expression ratio and (B) the number of DEG compared to the original dataset due to the correction of mRNA by miRNA using a combination of TargetScan score (top 25, 50, and 75 target scores or all miRNAs) with the proportion of hypothetical miRNA effect on mRNA (30%, 50%, 75%, or 83% mRNA reduction).
The overall effect of miRNA correction can be deduced from Figure 1.
The effect of miRNA on mRNA increased as the target score bin increased (ie, from 25 to all), and the magnitude of change increased as the hypothetical mRNA reduction by miRNA increased (ie, magnitude of miRNA effect or ME; from 30% to 83%), as expected. More than 6000 genes (35.6% of annotated genes measured) were affected by the miRNA correction when all miRNAs were used, and most of them were differentially expressed genes (DEG) between liver and mammary tissue (Fig. 1A and Supplementary File 3); this number was reduced to approximately 3700 genes (21.5% of annotated genes measured) when only the miRNA with the top 25% score was used. As expected, the number and percentage of mRNA affected were dependent only on the target bin used but not on the ME.
The expression ratio was affected by a combination of target score bin and ME (Fig. 1A). The change in the expression ratio of mRNA due to miRNA correction increased as the ME became larger but decreased as the target score bin increased, likely due to the higher target score miRNA:mRNA pairs being diluted out by the more prevalent lower score pairs. The variation in expression ratio was .15% with 83% ME and 25% target score bin (Fig. 1A), indicating that the miRNA had a substantial effect on the expression of genes; however, when a more conservative approach was taken (ie, 30% ME), the effect on expression ratio was ,5% (Fig. 1A). When considering only the genes that were differentially expressed between liver and mammary (FDR , 0.05) in the original dataset, the correction with miRNA tended to increase the expression ratio of liver over mammary tissue, especially when considering the more liberal miRNA effect (ie, 83% ME and 25% top target score or 83–25) indicating that most of the miRNAs measured had an overall larger effect on liver transcriptome (23.5% variation at 83–25) compared to mammary tissue (approximately 13.3% variation at 83–25). This is not simply due to the number of differentially expressed miRNA between the two tissues, as in fact mammary tissue had a larger number of differentially expressed miRNA more expressed compared to liver than vice versa (101 vs. 86). In addition, the data indicated that the effect of the miRNA was larger on mRNA that were differentially expressed compared to the ones that were not affected in the original dataset between liver and mammary tissue (Fig. 1A), indicating that the miRNA correction was more important on genes that participate to determine the difference between the two tissues, thus capturing the biologically relevant genes.
The combination of miRNA:mRNA target score and ME also affected the number of DEG. In general, we observed an increase in the absolute number of DEG with FDR , 0.05 and FDR , 0.01, as the target score bin increased and the ME increased, with almost 200 more DEG compared to the original dataset with the combination 83–all. When a FDR , 0.001 was considered, we observed a smaller change in the number of DEG, and there was a decrease in the number of DEG compared to the original dataset as the target score bin increased, which was visible for up to 50% ME. The number of DEG increased with higher ME. In all cases, the combination 83–all provided the largest change in the number of DEG with a maximum of 179 more DEG (Fig. 1B).
Furthermore, we have analyzed the percentage of variation in expression ratio between liver and mammary tissue in genes that were equally significant (FDR , 0.05) and equally nonsignificant (FDR . 0.05) in the original dataset and in the miRNA-corrected datasets (Fig. 2A). The trend was similar in both comparisons with an overall increase as the ME became larger. We detected an overall higher change in expression ratio in genes that were equally significant in the original dataset and in the miRNA-corrected dataset with almost 16% variation with the 83–25 combination compared to the equally nonsignificant genes (Fig. 2A). The genes that gained significance after miRNA correction had large increase in expression ratio as the ME increased with a tendency for a reduction as the target score bin increased. An increase in expression ratio up to 50% was detected with the higher ME (Fig. 2A). There were also more genes that acquired a statistical significance difference in expression ratio between liver and mammary tissue compared to the ones that lost significance after miRNA correction (Fig. 2B).
The above data support an overall large effect of miRNA correction on the mRNA expression. The data indicated a larger effect of miRNA on the liver transcriptome compared to the mammary tissue as there was a larger increase in the expression ratio of liver vs. mammary tissue compared to mammary tissue vs. liver (Fig. 2A).
The correction with the miRNA did not largely change the absolute number of DEG (Fig. 1B); however, the number of genes that gained or lost significance was relatively high with a peak of approximately 800 genes that change their statistical outcomes either gaining or losing significance (Fig. 2B). Thus, an important effect on the downstream bioinformatics analyses is expected.
The change in statistical outcomes can be better visualized by the correlation analyses of the FDR (ie, results of the statistical analysis; Fig. 3) and the expression ratio between liver and mammary (Supplementary Fig. 2) of each corrected dataset with the original transcriptomic dataset. The statistical analysis of the dataset was more affected by the miRNA correction than the overall expression ratio. Minimal correlations were observed between the 83–all and the original dataset for the FDR (Fig. 3) and for the expression ratio (Supplementary Fig. 2).
Effect of miRNA correction on the analysis of upstream regulators by IPA. The upstream regulator analysis was performed using the IPA and the DIA. The difference between the two approaches is quantitative with the IPA that provides an activation Z-score based on the significance and the expression ratio of the downstream genes plus an overlapping P-value (ie, statistical approach) and the DIA providing an impact and direction of the impact of the TF based on the significance and magnitude of expression ratio and proportion of differentially expressed target genes.22
An overall view of the effect of the miRNA correction on the activation Z-score is available in Figure 4. Overall, we observed a large correlation (.0.95) between the results of miRNA-corrected dataset vs. the original dataset with the lowest correlations detected with the ME of 83%. When the overlap P-value was considered (Supplementary Figure 3), the correlation was less, with minimum correlation of r = 0.905 detected for the 83–all combination. In the original dataset, 55 upstream regulators were identified to have an activation Z-score more than 2 (in absolute term, ie, both activated and inhibited). The correction with the miRNA identified from 1 (30–75 combination) to 10 (83–75 and 83–all combinations) upstream regulators that had passed the threshold of absolute Z-score of $2 in either the original dataset but not the corrected dataset or vice versa (Supplementary File 4). The correction with the miRNA identified up to seven upstream regulators in the combinations 75–all and 83–all that had a Z-score absolute value of $2 but were not identified in the original dataset to be relevant (ie, Z-score absolute value of ,2; Supplementary File 4). Similarly, using the overlap P-value of #0.01, the correction with miRNA identified up to six upstream regulators that were not captured by the analysis of the original dataset (Supplementary File 4).
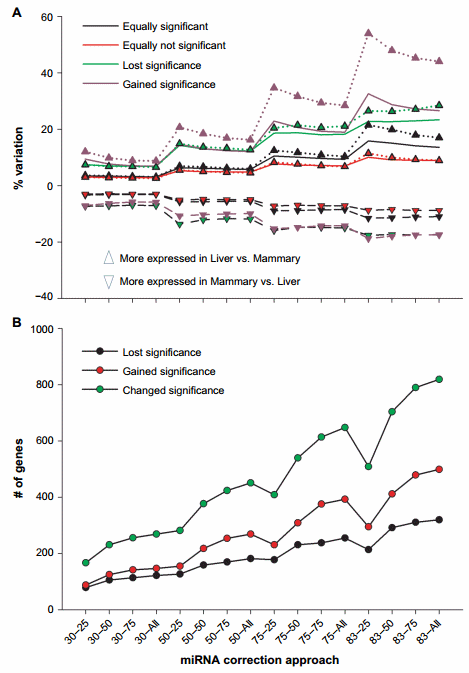
Figure 2. Effect of miRNA correction on statistical analysis. Summarized are (A) the percentage of variation on expression ratio between liver and mammary tissue of transcripts after miRNA correction divided into the ones that gained, lost, or did not change the significance (FDR # 0.05) in the expression between liver and mammary tissue and (B) the number of genes that gained, lost, or changed significance after the correction of mRNA by miRNA using a combination of TargetScan score (top 25, 50, and 75 target scores or all miRNAs) with the proportion of hypothetical miRNA effect on mRNA (30%, 50%, 75%, or 83% mRNA reduction).
The purpose of this work was to identify, by eliminating the posttranscriptional effect of miRNA, the genes whose transcription is really affected by the condition(s) studied. All genes are regulated by upstream regulators. Thus, the identification of upstream regulators estimated to be significantly perturbed in the miRNA-corrected dataset but not in the original dataset (or vice versa) is likely the upstream regulators that are hidden by the effect of miRNA. Among the hidden upstream regulators, some are of biological interest (see below). Figure 5 shows the upstream regulators that were identified as dissimilar between the original dataset and the miRNA datasets by the Z-score absolute value of $2 or the P-value of #0.01.
Z-score. Using the Z-score, we identified estrogen receptor 1 (ESR1), v-myc avian myelocytomatosis viral oncogene homolog (MYC), sphingosine-1-phosphate receptor 2 (S1PR2), and platelet-derived growth factor beta polypeptide as the most affected upstream regulators by the miRNA correction (Fig. 5A).
Figure 3. Effect of miRNA correction on statistical analysis. Spearman’s correlation analysis was performed on the FDR between the original dataset and each miRNA-corrected dataset using a combination of TargetScan score (top 25, 50, and 75 target scores or all miRNAs) with the proportion of hypothetical miRNA effect on mRNA (30%, 50%, 75%, or 83% mRNA reduction).
Estrogen is known to play a major role in mammary tissue development and function38,39 but also in liver, especially in females as observed in humans.40 In human liver, estrogen can play a role in metabolism and cancer prevention.41 Our data indicated that estrogen had a more important role in liver than mammary at the transcriptomic level and may have played a role in the difference between mammary tissue and liver.
MYC was identified to be highly relevant in the original dataset but not significant (Z-score absolute value of ,2) or not relevant at all (Z-score = 0) in miRNA-corrected datasets. MYC is a very important TF involved in a plethora of functions, chiefly cell cycle, apoptosis, cell differentiation, and cancer.42,43 In liver, MYC can also play a role in the inflammatory response,44 while in the mammary tissue, it is involved in development42 and apoptosis.45 The ubiquitous presence, including the roles in the mammary tissue and liver, and the multitude of functions46 make MYC an unlikely candidate to drive the transcriptomics difference between liver and mammary tissue. The correction with the miRNA seems to confirm this.
The S1PR2 is a G-protein-coupled receptor involved in 1-phosphate-induced cell proliferation. The correction of the original dataset with miRNA uncovered an important role of this receptor in the mammary tissue compared to the liver (Fig. 5A). This finding appears to contrast with the scientific literature, where an important role of S1PR2 has been identified in liver, such as a key role in the regulation of gene expression and lipid metabolism by bile acid,47,48 but to our knowledge no roles have been clearly identified in the mammary tissue.
For the other upstream regulators, the difference was about the magnitude of the Z-score. The angiotensinogen (AGT), prolactin (PRL), tumor necrosis factor (TNF), and vitamin A were detected to play an important role in the miRNAcorrected dataset but a less than significant role (Z-score absolute value of ,2) in the original dataset, ie, they would have been likely disregarded in the discussion of the results. Among the four upstream regulators, the PRL was induced in liver vs. mammary tissue. The PRL has a clear role in mammary gland49; however, a role in liver has been detected in rat as reviewed more than 20 years ago.50 Among the function of the PRL in the liver, there is an effect on ESR1 expression,51 indicating a connection between the higher activation of ESR1 and PRL in our study. Overall, the higher activation of estrogen (through ESR1) and PRL in liver than in the mammary tissue appears contradictory considering the larger importance of the two in the mammary tissue vs. liver. However, our data might indicate unknown major roles of the two upstream regulators in liver, which is partly supported by the relatively few studies cited earlier. The AGT, TNF, and vitamin A were more induced in the mammary tissue vs. liver (Fig. 5A). The AGT is a hormone produced by the liver with vasopressor function. Roles of AGT in mammary gland was reviewed52 and recently been detected to be important in mammary development in mice.53 The TNF is a proinflammatory cytokine known to play roles in the response of the mammary gland to mastitis.54 Vitamin A helps to prevent mastitis in mouse mammary gland,55 but it also increases the apoptosis of mammary epithelial cells during lactation in cows fed with enhanced vitamin A during the dry period.56
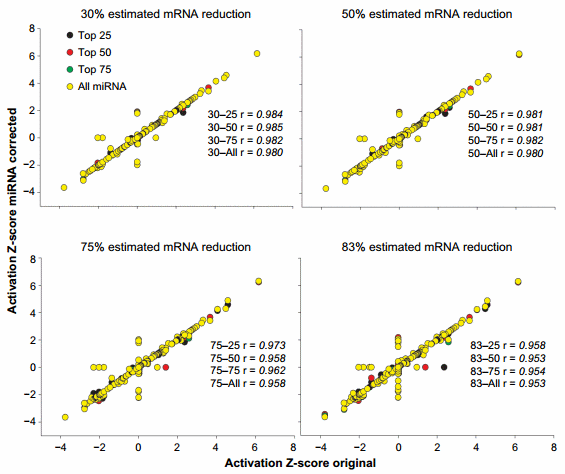
Figure 4. Effect of miRNA correction on activation Z-score. Spearman’s correlation analysis was performed on the activation Z-score of the upstream regulators obtained by IPA between the original dataset and each miRNA-corrected dataset using a combination of TargetScan score (top 25, 50, and 75 target scores or all miRNAs) with the proportion of hypothetical miRNA effect on mRNA (30%, 50%, 75%, or 83% mRNA reduction).
Overlap P-value. Different than the activation Z-score, the interpretation of the overlap P-value is more difficult because it only indicates that an upstream regulator is important but does not indicate for which tissue is more important. The association with the Z-score results can provide such information (Supplementary File 4). The use of the overlap P-value of #0.01 (or log10 P-value = 2) indicated that insulin, methionine adenosyltransferase I alpha, Niemann–Pick disease type C1, and progesterone receptor are important upstream regulators when the dataset was corrected by the miRNA but not in the original dataset, while lysine (K)-specific demethylase 8 was only important in the original dataset. All the above were more induced in the mammary tissue than in liver (Z-score in Supplementary File 4). PPARGC1B was not important when the original dataset was corrected with 75% and 83% ME with the all miRNAs or the top 75% of miRNA:mRNA pairing (Fig. 5B), but the Z-score analysis indicated a higher activation in liver than in the mammary tissue in the original dataset (Supplementary File 4).
Overall, we know that insulin and progesterone are very important regulators of lactation in the mammary gland.57,58 Methionine adenosyltransferase I alpha, a methionine adenosyltranferase, involved in epigenetic regulation and Niemann– Pick disease type C1 involved in the control of cholesterol trafficking are likely important for the liver rather than mammary tissue,59,60 although the Z-score indicated otherwise (Supplementary File 4). Lysine (K)-specific demethylase 8 plays a role in controlling the cell proliferation in a human mammary epithelial cell line61 via epigenetic regulation. Our analysis indicates that this upstream regulator would have been a false positive if the original dataset was not corrected by miRNA.
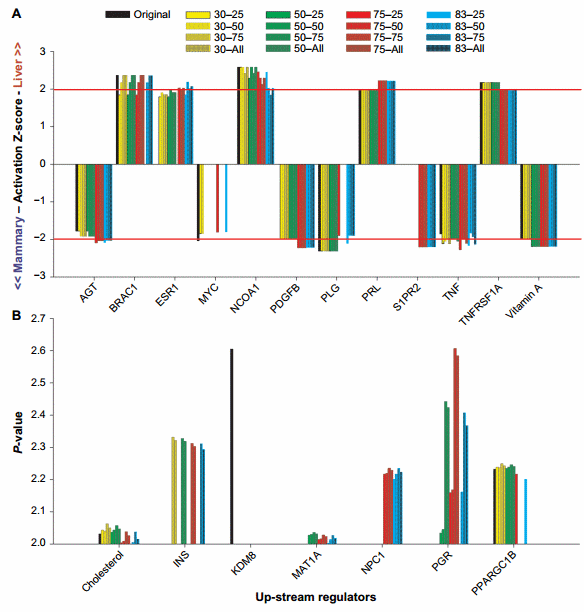
Figure 5. Top upstream regulators affected by the miRNA correction in IPA. Reported are the results of the upstream regulators that were dissimilar between the original dataset and the miRNA datasets by the Z-score $ 2 (A) or the P-value #0.01 (or 2.0 as −log10 overlap P-value; B). X-axis indicates the combination of TargetScan score (top 25, 50, and 75 target scores or all miRNAs) with the proportion of hypothetical miRNA effect on mRNA (30%, 50%, 75%, or 83% effect on reducing the mRNA abundance)
In summary, the correlation analysis suggested a relatively modest effect of the correction with miRNA on the upstream regulator analysis using the IPA. However, the effect was substantial upon specific upstream regulators. The correction with miRNA allowed identification of several new upstream regulators that were not identified by using the original dataset that may have important biological functions. Also, it allows the elimination of some upstream regulators that in reality are not important in the transcription regulation determining the difference between mammary tissue and liver.
Even though an effect of the miRNA correction on the upstream regulator was observed, when the upstream regulators with the highest Z-score are considered (eg, $[3], encompassing .30 upstream regulators; Supplementary File 4), the difference between the datasets was negligible, and overall, it would not have affected the final interpretation of the data. The lack of effect was likely due to the very large transcriptomic difference between liver and mammary tissue. It is plausible that the transcriptomic difference between the two tissues is mostly due to other epigenetic factors besides miRNA, such as methylation and histone modifications. The effect of miRNA on the transcriptome and the effect on the interpretation of upstream regulators would likely be larger if the analysis is carried out in a dataset with less drastic differences, such as an experiment performed in the same tissue under different conditions, where the effect of other epigenetic factors is likely minor.
Effect of miRNA correction on the analysis of upstream TFs by Dynamic Impact Approach. Complete results from the DIA analysis of upstream TFs are reported in Supplementary File 5. Limitations exist for the use of the human-annotated database with bovine: 130 out of 280 TFs (46.4%) have one annotated downstream target gene and 233 TFs (83.1%) have ,10 downstream target genes. When considering the one annotated in the microarray used in the present experiment, we had 17.5% of TF without downstream target genes, 33% of TF with only 1 downstream target gene, and 14% of TF with only 2 downstream target genes. In addition, the target genes of the TF might be very different between the two species; however, a similar database for bovine is not available.
In order to partly account for the above, we did not apply any cutoff criteria in the DIA analysis regarding the number of downstream target genes, but we discussed in more detail the TF with $2 annotated downstream target genes in the microarray. We have, however, reported the total number of annotated downstream target genes and the one differentially affected in each comparison in Supplementary File 5.
The correlation between using the original dataset and the miRNA-corrected dataset from the impact obtained by DIA is available in Figure 6 and that from the direction of the impact is available in Supplementary Figure 4. The miRNA correction had a minimal effect on the overall results of DIA analysis of upstream TFs with a correlation of .0.99 with the original dataset.
Even though the overall effect was minor, the effect on specific TFs was relatively large (Supplementary File 5). Figure 7 shows the results for the 12 TFs with at least two annotated downstream target genes in the microarray and with the largest change in the direction of impact due to miRNA correction.
Cut-like homeobox 1 (CUTL1) is a TF involved in morphogenesis and differentiation. Based on the literature, this TF appears to play a more important role in liver than in the mammary tissue.62,63 The correction of the dataset with the miRNA indicated a prominent role of this TF in liver over mammary (Fig. 7), supporting the findings from the literature.
Upstream TF 1 (USF1) is ubiquitously expressed and associated with hyperlipidemia and metabolic syndrome, particularly in liver.64 Our data indicated that this TF was more activated in liver than in the mammary tissue in the original dataset, and the correction by all miRNAs disregarding their target score revealed an even higher activation in liver vs. mammary tissue (Fig. 7).
The cAMP responsive element-binding protein (CREB1) 1 is a TF involved in glucose homeostasis, cell survival, and neurological functions.65 The importance of CREB1 in mammary tissue appears minor, with some role in breast cancer,66 while it is known to play more important roles in liver, such as in hepatic glucose metabolism.67 The impact of this TF decreased as the correction of the miRNA heightened, but a higher activation in the liver was revealed (Fig. 7).
The impact of the member of ETS oncogene family (ELK1) in participating to the transcriptomic difference between liver and mammary tissue was very large in the original dataset but decreased with the miRNA correction at 75% and 83% with the higher target score bins (Fig. 7). The effect was due to a single gene, the early growth response 1 (EGR1; Supplementary File 3), which was more expressed in mammary tissue vs. liver. Its level of expression ratio decreased in mammary tissue vs. liver after miRNA correction. This is the reason for the increase in the direction of the impact observed (Fig. 7). ELK1 plays an important role in liver regeneration,68 while its role in mammary tissue is not as clear.
The Spi-1 proto-oncogene (SPI1) plays a pivotal role in hematopoiesis. It is unclear why there is a higher activation in liver vs. mammary tissue; however, the liver is hematopoietic during the fetal stage. The correction with miRNA revealed an even larger activation of this TF in liver vs. mammary tissue despite the decrease in total impact (Fig. 7).
ESR1, as well as the DNA-damage-inducible transcript 3 (DDIT3) and the POU class 2 homeobox 1, (POU2F1) had a larger activation in the mammary tissue than in liver in the original dataset. For ESR1, the data from DIA (Fig. 7) appear to contrast with the Z-score data obtained through IPA (Fig. 5); however, as discussed earlier, ESR1 is known to play a more prominent role in the mammary tissue than in liver. Thus, the data from DIA are more supported by the literature. The data in the present analysis indicate that ESR1, with a large number of downstream target genes, likely plays an even more important role in the difference between liver and mammary tissue, as indicated by the larger activation in mammary after the miRNA correction (Fig. 7). DDIT3 and POU2F1 had a decreased activation in mammary after miRNA correction (Fig. 7). DDIT3 (a.k.a. CHOP) is known to play a proapoptotic role with, among others, an important role in the endoplasmic reticulum stress in liver.69 Endoplasmic reticulum stress also appears to be important for the lactating mammary tissue in bovine partly driven by an increase in the expression of CHOP70 during lactation. DDIT3 is more expressed in the mammary tissue than in liver (Supplementary File 3). The correction with the miRNA indicated that the activation of DDIT3 in mammary tissue is likely less pronounced than what is observed in the original dataset.
The importance of the nuclear receptor subfamily 3 group C member 1 (NR3C1; glucocorticoid receptor) as an upstream TF increased in liver vs. mammary tissue due to the miRNA correction (Fig. 7; see also NR3C2 in Supplementary File 5). Glucocorticoids play a major role in liver, especially in glucose homeostasis.71 In mammary tissue, their roles are not as prominent. However, these hormones are important in milk protein synthesis, especially for the mRNA stability of casein genes.72 Furthermore, a role of glucocorticoids in breast cancer has been established by recent data.73 Overall, more important active role of glucocorticoids should be expected in liver than in the mammary tissue.
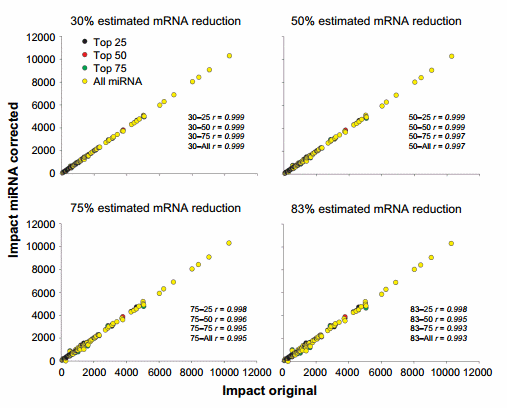
Figure 6. Effect of miRNA correction on the impact of TFs. Spearman’s correlation analysis was performed on the impact values of TFs obtained through the dynamic impact approach between the original dataset and each miRNA-corrected dataset using a combination of TargetScan score (top 25, 50, and 75 target scores or all miRNAs) with the proportion of hypothetical miRNA effect on mRNA (30%, 50%, 75%, or 83% mRNA reduction).
The ligand-dependent nuclear receptors, such as retinoidX-receptor beta (RXRB) and peroxisome proliferator-activated receptor gamma (PPARG), and the sterol response elementbinding factor 1 (SREBP1), were among the most affected TF by the miRNA correction. All three had a higher activation in liver vs. mammary tissue and a similar decrease in activation as the percentage of scoring pair increased (Fig. 7). This is due to the high miRNA correction of one common target gene, the LOC533894, or low-density lipoprotein receptor-related protein 1 (Supplementary File 4). All three TFs are somewhat involved in lipid metabolism. In bovine, PPARG and SREBP1 have been studied in more detail in the mammary tissue than in liver.74 Expression of all the genes coding for these TFs is higher in the mammary tissue than in liver (Supplementary File 3), but most of the differentially expressed target genes of these TFs in the HTRIdb were related to liver functions (eg, LRP1 and MMP1). Even though the correction with the miRNA did not reverse the activation status of the three TFs, it decreased their activation in liver than in the mammary tissue.
Overlap of TF results between DIA and IPA. Between IPA and DIA, 285 TFs were deemed to be partly responsible for differences in the transcriptome between liver and mammary tissue (ie, with a Z-score and/or an impact different than 0; Supplementary File 6). Among these TFs, only 38 (13%) TFs were present in the results of both bioinformatics approaches, likely due to the difference in databases used. When considering the estimated activation among the overlapping TFs, more than 65% were estimated to be activated in the same manner by both bioinformatics tools with a slight increase in concordance as the correction by the miRNA became more aggressive (65.8% in the original and 68.4% in 83–all; Supplementary File 6). Overall, the concordance of the upstream TF results between the two bioinformatics tools can be considered modest and tended to increase by the correction of the miRNA. Our data support the importance of using more than one bioinformatics tool to analyze the data and make conclusions, as previously suggested.75
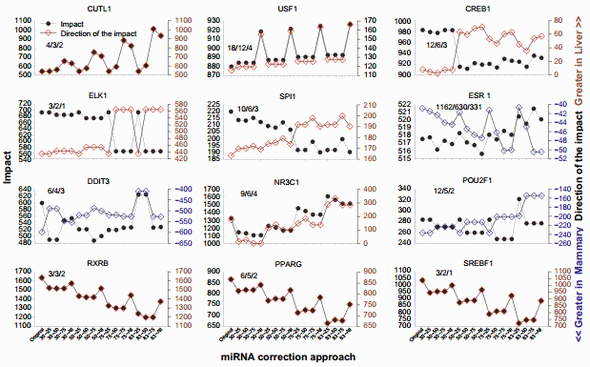
Figure 7. Top TFs affected by the miRNA correction in the dynamic impact approach. The top 12 TFs with at least two downstream target genes in the annotated microarray affected by the various combinations of miRNA correction of the original mRNA dataset (ie, TargetScan top 25%, 50%, and 75% target scores or all miRNAs with 30%, 50%, 75%, or 83% mRNA reduction). Shown are the impact (in the left Y-axis) and the direction of the impact (in the right Y-axis). The three numbers inside each graph denote the number of annotated target genes in the microarray, the number of differentially expressed target genes between liver and mammary, and the number of target genes affected by the miRNA correction, respectively
Summary and Conclusions
The liver and the mammary tissue have very specialized functions that are supported by the large difference in the transcriptome observed, including the miRNA transcriptome. The differentially expressed miRNA detected in this work was highly tissue specific and known to be related to either liver or mammary tissue. We have attempted to provide a bioinformatics method to correct the mRNA using the miRNA in order to improve the reliability of the upstream regulator analysis.
Our analysis indicated that the effect of miRNA was relatively large on the transcriptome, especially using the larger theoretical effect of the miRNA on the mRNA. The correction with the miRNA affected the statistical outcomes, revealing a larger number of DEG compared to what was observed in the original dataset. The change in the number of DEG had an effect on the upstream regulator analysis. The effect was more pronounced in the liberal miRNA:mRNA target score bins compared to the conservative approach (ie, top 25%) and in the higher assumed effect of miRNA on reducing the mRNA (ie, 83% mRNA reduction). The use of different criteria to correct the mRNA by the miRNA had a substantial effect on the dataset but did not have a large effect on the upstream regulator analysis.
The use of an enrichment analysis approach, yielding the activation Z-score and the overlap P-value, or DIA, which is not a statistical approach, revealed modest changes in the overall interpretation of upstream regulators. However, the effect on specific upstream regulators was substantial, ultimately affecting the interpretation of the data. Contrary to DIA outputs where the results were largely supported by the scientific literature, the results from the enrichment approach were not always supported by the scientific literature. We also detected a relatively modest overlap between the results of the two bioinformatics tools.
In conclusion, our approach allowed for a correction of the mRNA using the miRNA, and we demonstrated that this can affect the results of the upstream regulator analysis, particularly for specific upstream regulators.
The large transcriptomic difference between liver and mammary tissue observed might be resilient to miRNA effect, minimizing the possibility of observing large effects on the upstream regulator analysis. However, despite this, our approach was able to reveal the importance or reveal the lack of importance for several upstream regulators. For this reason, we expect the approach to have a larger effect in datasets where the differences between samples are not as large.
Author Contributions
Conceived and designed the experiments: DB, JW. Conceived the idea: MB. Conceived and developed the approach and the bioinformatics tool: SB. Analyzed the data: SB, MB. Wrote the first draft of the article: SB, MB. Contributed to the writing of the article: DB. Agreed with manuscript results and conclusions: SB, DB, JW, MB. Jointly developed the structure and arguments for the article: SB, MB. Made critical revisions and approved the final version: MB, DB. All authors reviewed and approved the final article.
Supplementary Material
Supplementary File 1. Scripts used to correct the mRNA using the miRNA.
Supplementary File 2. Complete statistical results for the miRNA dataset. Reported are the miRNA symbol, the FDR, the expression ratio between liver and mammary, and the number of calculated differentially expressed miRNA with two levels of FDR cutoff.
Supplementary File 3. Complete statistical results for the mRNA data for the original dataset (ie, not corrected with miRNA) and the dataset corrected with the miRNA using a combination of TargetScan score (top 25, 50, and 75 target scores or all miRNAs) with the proportion of hypothetical miRNA effect on mRNA (30%, 50%, 75%, or 83% effect on reducing the mRNA abundance).
Supplementary File 4. Complete results of upstream regulators from IPA. Reported are the results for the activation Z-score and for the overlap P-value for the original dataset (ie, not corrected with miRNA) and the dataset corrected with the miRNA using a combination of TargetScan score (top 25, 50, and 75 target scores or all miRNAs) with the proportion of hypothetical miRNA effect on mRNA (30%, 50%, 75%, or 83% effect on reducing the mRNA abundance).
Supplementary File 5. Complete results of upstream TFs from dynamic impact approach. Reported are the summary and detailed results for the impact and direction of the impact of the upstream TFs for the original dataset (ie, not corrected with miRNA) and the dataset corrected with the miRNA using a combination of TargetScan score (top 25, 50 and 75 target scores or all miRNAs) with the proportion of hypothetical miRNA effect on mRNA (30, 50, 75, or 83% effect on reducing mRNA abundance). Shown are also the number of differentially expressed downstream target genes for each TF and the calculation of the percentage of variation in impact and direction of the impact of the upstream TFs for all corrected dataset vs. the original dataset.
Supplementary File 6. Concordance of upstream analysis results between DIA and IPA.
Supplementary Figure 1. Venn diagram of target prediction for four databases. The Venn diagram reports the number of target genes that were identified by TargetScan, miRanda, DIANA, and PicTar and their overlap.
Supplementary Figure 2. Effect of miRNA correction on expression ratio between liver and mammary. Spearman’s correlation analysis was performed on the expression ratio between liver and mammary between the original dataset and each miRNA-corrected dataset using a combination of TargetScan score (top 25, 50 and 75 target scores or all miRNAs) with the proportion of hypothetical miRNA effect on mRNA (30%, 50%, 75%, or 83% effect on reducing mRNA abundance).
Supplementary Figure 3. Effect of miRNA correction on overlap P-value. Spearman’s correlation analysis was performed on the −log10 overlap P-value of the upstream regulators obtained by IPA between the original dataset and each miRNA-corrected dataset using a combination of TargetScan score (top 25, 50 and 75 target scores or all miRNAs) with the proportion of hypothetical miRNA effect on mRNA (30%, 50%, 75%, or 83% effect on reducing the mRNA abundance).
Supplementary Figure 4. Effect of miRNA correction on direction of the impact of transcriptional factors. Spearman’s correlation analysis was performed on the direction of the impact values of transcriptional factors obtained through the dynamic impact approach between the original dataset and each miRNA-corrected dataset using a combination of TargetScan score (top 25, 50, and 75 target scores or all miRNAs) with the proportion of hypothetical miRNA effect on mRNA (30%, 50%, 75%, or 83% effect on reducing mRNA abundance).
This article was originally published in Bioinformatics and Biology Insights 2015:9(S4) 33–48 doi: 10.4137/BBI.S29332. This is an Open Access article distributed under the terms of the Creative Commons CC-BY-NC 3.0 License.
References
1. Elgar G, Vavouri T. Tuning in to the signals: noncoding sequence conservation in vertebrate genomes. Trends Genet. 2008;24(7):344–52.
2. Huang B, Zhang R. Regulatory non-coding RNAs: revolutionizing the RNA world. Mol Biol Rep. 2014;41(6):3915–23.
3. Zeng Y. Principles of micro-RNA production and maturation. Oncogene. 2006;25(46):6156–62.
4. Dalmay T. Mechanism of miRNA-mediated repression of mRNA translation. Essays Biochem. 2013;54:29–38.
5. Jacobs E, Mills JD, Janitz M. The role of RNA structure in posttranscriptional regulation of gene expression. J Genet Genomics. 2012;39(10):535–43.
6. Sen R, Ghosal S, Das S, Balti S, Chakrabarti J. Competing endogenous RNA: the key to posttranscriptional regulation. Scientific World Journal. 2014;2014: 896206.
7. Maas S. Posttranscriptional recoding by RNA editing. Adv Protein Chem Struct Biol. 2012;86:193–224.
8. Shlyueva D, Stampfel G, Stark A. Transcriptional enhancers: from properties to genome-wide predictions. Nat Rev Genet. 2014;15(4):272–86.
9. Ditlevsen DK, Owczarek S, Berezin V, Bock E. Relative role of upstream regulators of Akt, ERK and CREB in NCAM- and FGF2-mediated signalling. Neurochem Int. 2008;53(5):137–47.
10. Yu FX, Mo JS, Guan KL. Upstream regulators of the Hippo pathway. Cell Cycle. 2012;11(22):4097–8.
11. Drackley JK, Veenhuizen JJ, Richard MJ, Young JW. Metabolic changes in blood and liver of dairy-cows during either feed restriction or administration of 1,3-butanediol. J Dairy Sci. 1991;74(12):4254–64.
12. Bionaz M, Loor JJ. Identification of reference genes for quantitative real-time PCR in the bovine mammary gland during the lactation cycle. Physiol Genomics. 2007;29(3):312–9.
13. National Research Council (U.S.). Subcommittee on Dairy Cattle Nutrition. Nutrient Requirements of Dairy Cattle. 7th rev. ed. Washington, DC: National Academy Press; 2001.
14. Zhao S, Zhao J, Bu D, Sun P, Wang J, Dong Z. Metabolomics analysis reveals large effect of roughage types on rumen microbial metabolic profile in dairy cows. Lett Appl Microbiol. 2014;59(1):79–85.
15. Lewis BP, Burge CB, Bartel DP. Conserved seed pairing, often flanked by adenosines, indicates that thousands of human genes are microRNA targets. Cell. 2005;120(1):15–20.
16. Friedman RC, Farh KKH, Burge CB, Bartel DP. Most mammalian mRNAs are conserved targets of microRNAs. Genome Res. 2009;19(1):92–105.
17. Vlachos IS, Paraskevopoulou MD, Karagkouni D, et al. DIANA-TarBase v7.0: indexing more than half a million experimentally supported miRNA:mRNA interactions. Nucleic Acids Res. 2015;43(Database issue):D153–9.
18. Ragan C, Zuker M, Ragan MA. Quantitative prediction of miRNA-mRNA interaction based on equilibrium concentrations. PLoS Comput Biol. 2011;7(2):e1001090.
19. Mullokandov G, Baccarini A, Ruzo A, et al. High-throughput assessment of microRNA activity and function using microRNA sensor and decoy libraries. Nat Methods. 2012;9(8):840–6.
20. Subkhankulova T, Gilchrist MJ, Livesey FJ. Modelling and measuring single cell RNA expression levels find considerable transcriptional differences among phenotypically identical cells. BMC Genomics. 2008;9:268.
21. Liang Y, Ridzon D, Wong L, Chen C. Characterization of microRNA expression profiles in normal human tissues. BMC Genomics. 2007;8:166.
22. Bionaz M, Periasamy K, Rodriguez-Zas SL, Hurley WL, Loor JJ. A novel dynamic impact approach (DIA) for functional analysis of time-course omics studies: validation using the bovine mammary transcriptome. PLoS One. 2012;7(3):e32455.
23. Bovolenta LA, Acencio ML, Lemke N. HTRIdb: an open-access database for experimentally verified human transcriptional regulation interactions. BMC Genomics. 2012;13:405.
24. Wingender E, Schoeps T, Donitz J. TFClass: an expandable hierarchical classification of human transcription factors. Nucleic Acids Res. 2013;41(Database issue):D165–170.
25. Mudunuri U, Che A, Yi M, Stephens RM. bioDBnet: the biological database network. Bioinformatics. 2009;25(4):555–6.
26. Lagos-Quintana M, Rauhut R, Yalcin A, Meyer J, Lendeckel W, Tuschl T. Identification of tissue-specific microRNAs from mouse. Curr Biol. 2002;12(9):735–9.
27. Hou J, Lin L, Zhou WP, et al. Identification of miRNomes in human liver and hepatocellular carcinoma reveals miR-199a/b-3p as therapeutic target for hepatocellular carcinoma. Cancer Cell. 2011;19(2):232–43.
28. Elmen J, Lindow M, Silahtaroglu A, et al. Antagonism of microRNA-122 in mice by systemically administered LNA-antimiR leads to up-regulation of a large set of predicted target mRNAs in the liver. Nucleic Acids Res. 2008;36(4):1153–62.
29. Gatfield D, Le Martelot G, Vejnar CE, et al. Integration of microRNA miR- 122 in hepatic circadian gene expression. Genes Dev. 2009;23(11):1313–26.
30. Meng ZP, Fu XH, Chen XS, et al. miR-194 is a marker of hepatic epithelial cells and suppresses metastasis of liver cancer cells in mice. Hepatology. 2010;52(6):2148–57.
31. Chao CH, Chang CC, Wu MJ, et al. MicroRNA-205 signaling regulates mammary stem cell fate and tumorigenesis. J Clin Invest. 2014;124(7):3093–106.
32. Gregory PA, Bert AG, Paterson EL, et al. The miR-200 family and miR-205 regulate epithelial to mesenchymal transition by targeting ZEB1 and SIP1. Nat Cell Biol. 2008;10(5):593–601.
33. Wang M, Moisa S, Khan MJ, Wang J, Bu D, Loor JJ. MicroRNA expression patterns in the bovine mammary gland are affected by stage of lactation. J Dairy Sci. 2012;95(11):6529–35.
34. Ma L, Reinhardt F, Pan E, et al. Therapeutic silencing of miR-10b inhibits metastasis in a mouse mammary tumor model. Nat Biotechnol. 2010;28(4):341–7.
35. Li Z, Liu H, Jin X, Lo L, Liu J. Expression profiles of microRNAs from lactating and non-lactating bovine mammary glands and identification of miRNA related to lactation. BMC Genomics. 2012;13:731.
36. Eades G, Yao Y, Yang M, Zhang Y, Chumsri S, Zhou Q. miR-200a regulates SIRT1 expression and epithelial to mesenchymal transition (EMT)-like transformation in mammary epithelial cells. J Biol Chem. 2011;286(29):25992–6002. 37. Vrba L, Garbe JC, Stampfer MR, Futscher BW. Epigenetic regulation of normal human mammary cell type-specific miRNAs. Genome Res. 2011;21(12):2026–37.
38. Manavathi B, Samanthapudi VS, Gajulapalli VN. Estrogen receptor coregulators and pioneer factors: the orchestrators of mammary gland cell fate and development. Front Cell Dev Biol. 2014;2:34.
39. Stingl J. Estrogen and progesterone in normal mammary gland development and in cancer. Hormones Cancer. 2011;2(2):85–90.
40. Villa E. Role of estrogen in liver cancer. Women’s Health. 2008;4:41–50.
41. Zhao Y, Li Z. Interplay of estrogen receptors and FOXA factors in the liver cancer. Mol Cell Endocrinol. 2015;418(pt 3):334–9.
42. Hynes NE, Stoelzle T. Key signalling nodes in mammary gland development and cancer: Myc. Breast Cancer Res. 2009;11(5):210.
43. Stine ZE, Walton ZE, Altman BJ, Hsieh AL, Dang CV. MYC, metabolism, and cancer. Cancer Discovery. 2015;5(10):1024–39.
44. Liu T, Zhou Y, Ko KS, Yang HP. Interactions between Myc and mediators of inflammation in chronic liver diseases. Mediators Inflamm. 2015;2015:276850.
45. Sutherland KD, Lindeman GJ, Visvader JE. Knocking off SOCS genes in the mammary gland. Cell Cycle. 2007;6(7):799–803.
46. Cowling VH, Cole MD. Mechanism of transcriptional activation by the Myc oncoproteins. Semin Cancer Biol. 2006;16(4):242–52.
47. Nagahashi M, Takabe K, Liu RP, et al. Conjugated bile acid-activated S1P receptor 2 is a key regulator of sphingosine kinase 2 and hepatic gene expression. Hepatology. 2015;61(4):1216–26.
48. Kwong E, Li Y, Hylemon PB, Zhou H. Bile acids and sphingosine- 1-phosphate receptor 2 in hepatic lipid metabolism. Acta Pharmaceutica Sinica B. 2015;5(2):151–7.
49. Trott JF, Schennink A, Petrie WK, Manjarin R, VanKlompenberg MK, Hovey RC. Triennial lactation symposium: prolactin: the multifaceted potentiator of mammary growth and function. J Anim Sci. 2012;90(5):1674–86.
50. Gustafsson JA, Mode A, Norstedt G, Eneroth P, Hokfelt T. Central control of prolactin and estrogen receptors in rat liver – expression of a novel endocrine system, the hypothalamo-pituitary-liver axis. Annu Rev Pharmacol Toxicol. 1983;23:259–78.
51. Frasor J, Gibori G. Prolactin regulation of estrogen receptor expression. Trends Endocrinol Metab. 2003;14(3):118–23.
52. Vinson GP, Barker S, Puddefoot JR. The renin-angiotensin system in the breast and breast cancer. Endocr-Relat Cancer. 2012;19(1):R1–19.
53. Murata K, Baasanjav A, Kwon C, Hashimoto M, Ishida J, Fukamizu A. Angiotensin II accelerates mammary gland development independently of high blood pressure in pregnancy-associated hypertensive mice. Physiol Rep. 2015;3(9):e12542.
54. Gunther J, Liu SZ, Esch K, Schuberth HJ, Seyfert HM. Stimulated expression of TNF-alpha and IL-8, but not of lingual antimicrobial peptide reflects the concentration of pathogens contacting bovine mammary epithelial cells. Vet Immunol Immunopathol. 2010;135(1–2):152–7.
55. Chew BP, Zamora CS, Luedecke LO. Effect of vitamin-a-deficiency on mammarygland development and susceptibility to mastitis through intramammary infusion with staphylococcus-aureus in mice. Am J Vet Res. 1985;46(1):287–93.
56. Puvogel G, Baumrucker CR, Sauerwein H, et al. Effects of an enhanced vitamin A intake during the dry period on retinoids, lactoferrin, IGF system, mammary gland epithelial cell apoptosis, and subsequent lactation in dairy cows. J Dairy Sci. 2005;88(5):1785–800.
57. Akers RM. Major advances associated with hormone and growth factor regulation of mammary growth and lactation in dairy cows. J Dairy Sci. 2006;89(4): 1222–34.
58. Massimo Bionaz, Walter Hurley and Juan Loor (2012). Milk Protein Synthesis in the Lactating Mammary Gland: Insights from Transcriptomics Analyses, Milk Protein, Dr. Walter Hurley (Ed.), InTech, DOI: 10.5772/46054. Available from: http://www.intechopen.com/books/milk-protein/milk-protein-synthesisin-the-lactating-mammary-gland-insights-from-transcriptomics-analyses.
59. Corrales FJ, Perez-Mato I, Sanchez Del Pino MM, et al. Regulation of mammalian liver methionine adenosyltransferase. J Nutr. 2002;132(8 Suppl):2377S–81S.
60. Yu XH, Jiang N, Yao PB, Zheng XL, Cayabyab FS, Tang CK. NPC1, intracellular cholesterol trafficking and atherosclerosis. Clin Chim Acta. 2014;429: 69–75.
61. Hsia DA, Tepper CG, Pochampalli MR, et al. KDM8, a H3K36me2 histone demethylase that acts in the cyclin A1 coding region to regulate cancer cell proliferation. Proc Natl Acad Sci U S A. 2010;107(21):9671–6.
62. Sansregret L, Nepveu A. The multiple roles of CUX1: insights from mouse models and cell-based assays. Gene. 2008;412(1–2):84–94.
63. Liu KC, Lin BS, Zhao M, Wang KY, Lan XP. Cutl1: a potential target for cancer therapy. Cell Signal. 2013;25(1):349–54.
64. Shoulders CC, Naoumova RP. USF1 implicated in the aetiology of familial combined hyperlipidaemia and the metabolic syndrome. Trends Mol Med. 2004;10(8):362–5.
65. Mayr B, Montminy M. Transcriptional regulation by the phosphorylationdependent factor CREB. Nat Rev Mol Cell Biol. 2001;2(8):599–609.
66. Chhabra A, Fernando H, Watkins G, Mansel RE, Jiang WG. Expression of transcription factor CREB1 in human breast cancer and its correlation with prognosis. Oncol Rep. 2007;18(4):953–8.
67. Lee D, Le Lay J, Kaestner KH. The transcription factor CREB has no nonredundant functions in hepatic glucose metabolism in mice. Diabetologia. 2014;57(6):1242–8.
68. Wuestefeld T, Pesic M, Rudalska R, et al. A direct in vivo RNAi screen identifies MKK4 as a key regulator of liver regeneration. Cell. 2013;153(2):389–401.
69. Malhi H, Kaufman RJ. Endoplasmic reticulum stress in liver disease. J Hepatol. 2011;54(4):795–809.
70. Invernizzi G, Naeem A, Loor JJ. Short communication: endoplasmic reticulum stress gene network expression in bovine mammary tissue during the lactation cycle. J Dairy Sci. 2012;95(5):2562–6.
71. Kuo T, McQueen A, Chen TC, Wang JC. Regulation of glucose homeostasis by glucocorticoids. Adv Exp Med Biol. 2015;872:99–126.
72. Rhoads RE, Grudzien-Nogalska E. Translational regulation of milk protein synthesis at secretory activation. J Mammary Gland Biol Neoplasia. 2007;12(4):283–92.
73. Kach J, Conzen SD, Szmulewitz RZ. Targeting the glucocorticoid receptor in breast and prostate cancers. Sci Transl Med. 2015;7(305):305s319.
74. Bionaz M, Osorio J, Loor JJ. TRIENNIAL LACTATION SYMPOSIUM: nutrigenomics in dairy cows: nutrients, transcription factors, and techniques. J Animal Sc. 2015;93(12):5531–53.
75. Huang da W, Sherman BT, Lempicki RA. Bioinformatics enrichment tools: paths toward the comprehensive functional analysis of large gene lists. Nucleic Acids Res. 2009;37(1):1–13.