1. Factorially designed experiments versus response surface methodology.
A conventional experimental approach investigates the effects of one independent variable while keeping all other variables constant. This approach faces two challenges (i) it is only valid when the underlying principles linking cause and effect are known with some certainty; (ii) variables may interact with each other and the magnitude of the effect caused by altering one factor will depend on the magnitude of one or more of the other variables (Armstrong, 2006). Factorial designs were developed to meet these challenges. They evaluate the impact of multiple variables simultaneously, assesses their relative importance, and determine the interactions between these factors, although careful consideration must be given to the factors to be investigated and the manipulated levels of each factor (Fisher, 1926). Full factorial designs are probably the most widely applied experimental approach in poultry nutrition research. They are particularly useful for identifying the significance of multiple influential factors, a useful step in preliminary stages of research to filter out unimportant variables. Once the importance of independent variables has been established, quantification of the magnitude of the response is usually required in further research for the purposes of optimisation and prediction. Response surface methodology can visualise the effects of variables by using contour plots or three-dimensional diagrams. The common approaches of response surface experimental design include Box-Behnken Design (BBD) and Central Composite Design (CCD) and to a lesser extent, mixture design.
Compared with response surface methodology, one major disadvantage of factorial experiments is that the number of treatments and/or replicates can become excessive very quickly. Table 1 compares the number of treatments and efficiency in factorial designs with two response surface designs. The efficiency was calculated by dividing the number of coefficients in the second order model with the number of treatment combination needed per number of factors being tested. As shown in Table 1, the central composite design and Box-Behnken design requires smaller number of treatments than factorial design; therefore, they have been used in poultry nutrition research occasionally.
Another potential challenge of factorial designs is that the interactions between independent variables often become difficult to interpret when several factors are involved. Response surface methodology may visualise the interaction clearly. For example, Liu et al. (2014a) investigated the effects of phytase supplementation on growth performance, nutrient utilisation and the importance of starch and protein digestive dynamics in relation to feed conversion efficiency. Figure 1 illustrates how response surface methodology detects the relative importance between the digestion rates of starch and protein in broiler chickens. The relationship indicates that feed conversion efficiency may be enhanced by manipulating starch and protein digestion rates. The synchrony of protein and carbohydrate inputs can influence protein synthesis and of feed efficiency so that starch and protein digestive dynamics should not be treated separately but in combination. A balanced provision of glucose and amino acids at sites of protein synthesis is a fundamental prerequisite for efficient growth (Geiger, 1950). In order to achieve the optimal feed conversion ratio, which is shown as the bright white area in Figure 1, the protein digestion rates are more sensitive and demanding than starch. This suggests that the digestion rate of protein is more integral to optimal feed conversion ratios in broiler chickens. Thus strategies to accelerate protein relative to starch digestion should be advantageous. Such strategies could include the incorporation of ‘fast’ proteins, free amino acids, and exogenous phytase or protease into broiler diets. Alternatively, whole grain feeding may retard starch digestion rates (Liu et al., 2014b) to generate a better balance between protein and starch digestion rates.
Table 1: Comparison of treatment numbers and efficiency in factorial design and response surface methodology [adapted from Ferreira et al. (2007); De Leon et al. (2010)].
Figure 1: Thin-plate spline response surfaces for the relationship of feed conversion ratio (FCR) with starch and protein (nitrogen) digestion rates (× 10-2 min-1) (Liu et al., 2014a).
Response surface methodology was adopted in two recent studies to predict requirements of methionine, lysine, and threonine in broiler chickens (Mehri et al., 2012, Mehri, 2014). As shown in Figure 2, Mehri et al. (2012) reported that the maximum weight gain at the stationary point may be obtained with 0.54, 1.12, and 0.78% of digestible methionine, lysine and threonine in the diet, respectively, and the optimal feed conversion may be obtained when the diet contains 0.53, 1.13, and 0.75% of digestible methionine, lysine and threonine, respectively. Estimated ideal ratios of digestible methionine and threonine to lysine were 48 and 70% for weight gain and 47 and 66% for FCR. Basically, response surface method is suitable to examine the effects of numerical variables; alternative applications in poultry nutrition include the investigations of exogenous enzyme efficacy (Zheng et al., 2014), interactions between minerals (Bradbury et al., 2014, Wilkinson et al., 2014), interactions between anti-nutritional factors in feed and optimal feed processing strategies.
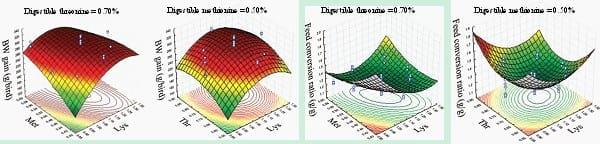
Figure 2: Response surface plots of weight gain and feed conversion ratio from broilers offered diets with different levels (%) of lysine, methionine and threonine from 3 to 16 days post-hatch (Mehri et al., 2012).
2. Right-angled mixture triangle.
Response surface methodology can be applied to explore the effects of numerical variables, for example, environmental temperature, humidity and lighting regimen on feed intake, or the steam-pelleting conditioning temperatures, retention times and feed additive inclusions on growth performance. However, poultry diets are mixtures of various feedstuffs including grains, protein meals, and a range of feed ingredients. Any alteration of the proportion of one component in the mixture must of necessity change the inclusion rate of at least one other component. Moreover, in practice, nutritionists formulate poultry diets based on apparent metabolisable energy. In poultry nutrition research, total dietary metabolisable energy (ME) is often maintained constant when investigating the effects of different nutritional components in the diet. For example, the change of dietary protein concentration will alter the concentrations of fat and starch in the diet to maintain total ME constant. Conventional factorial experimental designs and response surface methods introduced in the previous section cannot distinguish between the effects of protein concentration per se and the effects of starch and fat in the mixture. This demonstrated that mixture design is extremely suitable for poultry nutrition research, especially in studies involving isoenergetic and/or isonitrogenous diets.
Gous and Swatson (2000) used a mixture experiment to determine weight gain and food conversion efficiency of broiler chickens offered 1 of 13 combinations of three protein sources (fishmeal, sunflower oilcake meal and soybean oilcake meal) from 7 to 21 days post-hatch. Figure 3 shows the contour plots of the effects of the three protein sources on growth performance of broiler chickens. This is one of the very few studies embracing a mixture design in poultry nutrition. They compared the selections made by the birds when given a choice and the prediction of optimal weight gain and feed conversion based upon response surfaces. The study showed that the choices made by the broiler chickens coincided with those mixtures that maximised body weight gain and feed conversion efficiencies.
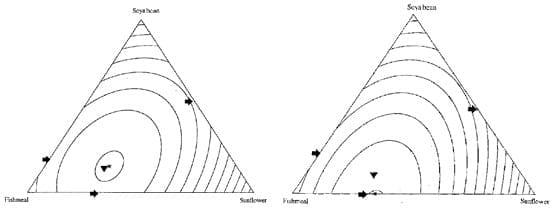
Figure 3: Contours in a 3-component mixture representing different combinations of soya bean oilcake meal, fishmeal and sunflower oilcake meal that result in similar weight gain (left) and food conversion efficiencies (right) (maximum*) (Gous and Swatson, 2000).
In 1993, an integrated approach to animal nutrition and feeding behaviour was introduced. It is termed the geometric framework for nutrition, and it satisfies the multiple-food-components requirement using a nutrient space which is a geometric space built of two or more axes, where each axis represents a food component that is suspected to play a role in influencing the responses of animals to the environment (Raubenheimer and Simpson, 1993, Simpson and Raubenheimer, 1993) (for a recent comprehensive review see Simpson and Raubenheimer (2012)). The geometric framework generates a spatial metaphor whereby relationships among biological components can be visualised and interpreted within the same multi-nutrient context. Such components might include the target intake towards which it would regulate if unconstrained (“intake target”, represented by a point or small region), its current nutritional state (a point), foods (lines called “nutritional rails” radiating from the origin into the nutrient space at an angle determined by the ratio of the nutrients in the food), and the constrained responses when the animal has available only foods that do not enable it to reach the intake target (“rules of compromise”). Together, these concepts provide a powerful means to study the appetite systems for different nutrients, and how these interact in the regulation of food intake and its consequences.
The right-angled mixture triangle (RMT) was introduced into the geometric framework system, which differs from the conventional equilateral mixture triangle (EMT) design. Figure 4 illustrates the geometric difference between EMT and RMT designs (Raubenheimer, 2011). Although the information content of RMT and EMT is equivalent, in EMT the angle subtending any two axes is 60°; whereas, RMT provides rectangular spaces which are more straightforward and readily interpreted. The rectangular space has the advantage that it more intuitively parses the relationships among components in the model into two- and three-way interactions, thus aiding the user to interpreting complex patterns (Raubenheimer 2011). To illustrate, figure 5 provides a comparison between EMT and RMT where the same data, fructose–glucose–sucrose composition of floral nectar in 26 plant species from temperate forests in South America, are plotted in both formats. The pattern of data scatter is equivalent in the two plots, but the point of difference between the two plots is that for many the patterns can more readily be related to the axes in the RMT than in the EMT. Fig. 5A clearly shows that the balance of fructose and glucose was comparatively constant across samples (the positive relationship between these variables), while the sucrose concentration varied widely (the spread of data along the glucose: fructose balance vector).
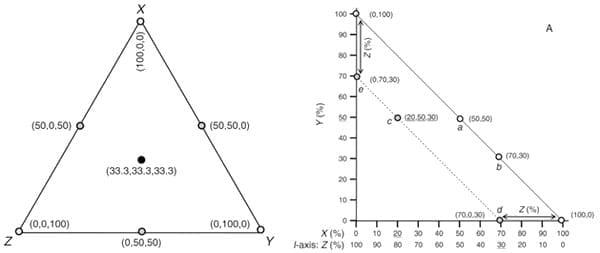
Figure 4: Left: Equilateral mixture triangle defining the mixture space of all possible blends of three components X, Y, and Z. Shown in parentheses are the X, Y, Z coordinates for seven mixtures: pure ingredients of X, Y, and Z (open circles), 50%:50% mixtures of two ingredients (grey circles), and an equal mixture of all three components (black circle). Right: A three-component right-angled mixture triangle with focal axes X and Y, and implicit axis Z (labelled using the generic notation I). Any two-component mixture of X+Y (i.e., where Z=0%) will fall on the concentration isoline described by equation Y=100-(X+0) (i.e., X+Y=100%), shown as a solid diagonal in the figure (e.g., mixtures a and b). If Z is added at 30% then, by the same logic, the possible mixtures fall on the dashed isoline X+Y=70% (e.g., mixtures e, c, and d). In general, the X+Y isoline for any value of Z can be plotted as a line joining the point 100%-Z on the X-axis with the same value on the Y-axis (an exception is where Z = 100%, in which case the isoline reduces to a point with (X, Y) coordinates (0, 0)). Because these X+Y isolines are given by the equation Z=100-(X+Y), in the special case where the isoline contacts the X-axis (i.e., Y=0), this reduces to Z=100-X. The value of Z can thus be read off an axis that transforms X by this function (axis I in the figure). As an example, the (X, Y, Z) coordinates for point c are underlined on the three axes (20, 50, 30).
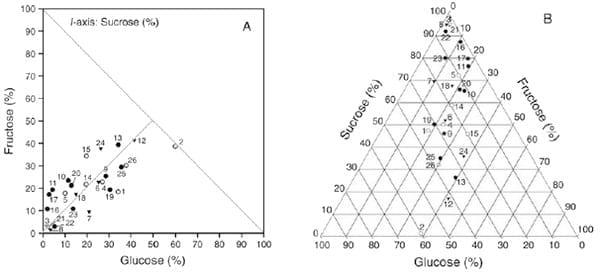
Figure 5: (A) RMT and (B) EMT showing the fructose–glucose–sucrose composition of floral nectar in 26 plant species from temperate forests in South America, which are associated with one of four pollinator groups (solid circles, hummingbirds; open circles, diurnal longtongued insects; solid triangles, diurnal short-tongued insects; open triangles, nocturnal insects) (Raubenheimer, 2011)
3. Application in poultry nutrition.
Only recently, the geometric framework has been applied in poultry nutrition (Bao et al., 2012, Bradbury et al., 2014, Wilkinson et al., 2014). The interaction between calcium (Ca) and phosphorus (P) represents a complex relationship in broiler chickens, which is influenced by vitamin D and phytase (Selle et al., 2000). Numerous studies have been reported in the literature to determine Ca and P requirements for optimal growth performance and skeletal integrity. However, the interactions between Ca, P and possibly, other minerals have resulted in widely inconsistent recommendations for dietary levels of Ca and P in the literature. By using geometric framework approach, Bradbury et al. (2014) examined the interaction between calcium (Ca) and non-phytate phosphorus (nPP) in growth performance and skeletal integrity. Fifteen dietary treatments with five different ratios of Ca : nPP (4, 2.75, 2.1, 1.5 and 1.14 : 1) and three total densities of Ca and nPP (12, 13.5 and 15 g/kg) were offered to broiler chickens from 7-28 days post-hatch. It was found that broilers are willing to over consume nPP to defend a Ca intake target more so than they are willing to over consume Ca to defend an nPP target; overall dietary nPP was more influential on performance metrics. However, from the data, it appears that birds assign higher priority to Ca than nPP intakes – i.e. when confined to one of a range of diets varying in the Ca:nPP ratio, they will let nPP intake vary with diet composition to meet the Ca target. Figure 6 illustrates the interactive effect of dietary Ca and nPP on broiler body weight gain and feed conversion ratio from 7 to 28 days post-hatch in the Bradbury et al. (2014) study. At low Ca and high nPP or high Ca and low nPP diets, birds had reduced feed intake, body weight gain, poorer feed efficiency and lower tibia ash. Similarly, from the same feeding study, Wilkinson et al. (2014) reported that the ratio of Ca : nPP is more influential to mineral digestibility than the absolute dietary concentration of each macro-mineral and nitrogen digestibility showed discrete optima around 10.0 and 5.0 g/kg nPP and Na digestibility was maximised around 8 to 9.0 g/kg Ca and 4.5 to 5.4 g/kg nPP (Figure 7).
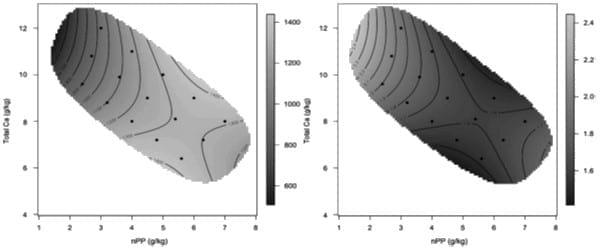
Figure 6: Graphical representation of the interactive effect of dietary Ca and nPP on broiler body weight gain (g/kg) (left) and feed conversion ratio (g/g) (right) from 7 to 28 days post-hatch (Bradbury et al., 2014).
Figure 7: Graphical representation of the interactive effect of dietary Ca and nPP on digestibility of sodium (left) and nitrogen (right) at 28 days post-hatch (Wilkinson et al., 2014).
A recently completed study by the Poultry Research Foundation assessed interactions between starch, protein and fat on growth performance in broiler chickens offered synthetic diets (unpublished data). The feeding study comprised 21 dietary treatments with various concentrations of starch and protein by using right-angled mixture triangle design. The experimental diets were based on corn-starch, casein, isolated soy protein, synthetic amino acids, sunflower oil and other minor ingredients. These isoenergetic diets were offered to broiler chickens from 10-23 days post-hatch. Interestingly, energy derived from protein has a more pronounced impact on weight gain than ‘non-protein energy’. Fat had nearly no impact on feed conversion ratios in comparison with protein energy intake; however, fat may influence energy utilisation and carcass compositions, which will be reported in subsequent publications (Figure 8). More importantly, as shown in Figure 9, the optimal weight gain (529g/bird) was achieved when dietary energy contributions of starch, protein and fat equalled to 39.98%, 47.21% and 12.81%, respectively. These equate to 326, 414 and 51 g/kg dietary starch, protein and fat, respectively, in a diet containing 3250 kcal/kg (13.60 MJ/kg) of metabolisable energy. The predicted optimal FCR (1.398) was achieved when energy contributions of starch, protein and fat equalled to 48.19%, 41.00% and 10.81%, respectively, which equates to 393 g/kg starch, 360 g/kg protein and 43 g/kg fat in the diet.
4. Conclusions.
Relationships between food compositions, diet composition, diet utilisation and performance are complex and multi-dimensional. The geometric framework offers an approach for conceptualising these relationships, and constructing models which identify key relationships needed to understand how the nutritional regulatory systems of animals interact with the compositions of foods to determine outcomes such as growth, body composition, feed conversion efficiency, fecundity and so forth. Understanding these relationships is an important step towards identifying the key managing the key control points to manage these for optimal production outcomes. As yet, the use of this approach in poultry production is in its infancy, but a detailed example illustrating these points can be found for finfish production in aquaculture (Ruohonen et al., 2007).
Figure 8: Thin-plate spline response surfaces of weight gain and FCR for protein, starch, or fat (unpublished data).
Figure 9: The contour of starch and protein percentage energy contributions on weight gain (left) and FCR (right) (unpublished data).
References.
1. Armstrong NA (2006) Pharmaceutical experimental design and interpretation, 2 edn. CRC press: Boca Raton, Florida.
2. Bao Y, Romero LF, Cowieson AJ (2012) A systematic geometric approach to the predicition of feed enzyme efficacy in broilers. Proceedings of the 23rd Annual Australian Poultry Science Symposium, Sydney, New South Wales, Australia, 19-22 February 2012, 75.
3. Bradbury EJ, Wilkinson SJ, Cronin GM, Thomson PC, Bedford MR, Cowieson AJ (2014) Nutritional geometry of calcium and phosphorus nutrition in broiler chicks. Growth performance, skeletal health and intake arrays. Animal 8, 1071-1079.
4. De Leon AC, Kidd MT, Corzo A (2010) Box-Behnken Design: alternative multivariate design in broiler nutrition research. Worlds Poultry Science Journal 66, 699-706.
5. Ferreira SLC, Bruns RE, Ferreira HS, Matos GD, David JM, Brandao GC, da Silva EGP, Portugal LA, Reis PS, Souza AS, dos Santos WNL (2007) Box-Behnken design: An alternative for the optimization of analytical methods. Analytica Chimica Acta 597, 179-186.
6. Fisher RA (1926) The design of experiments. Oliver & Boyd: Edinburgh.
7. Geiger E (1950) The role of the time factor in protein synthesis. Science 111, 594-599.
8. Gous RM, Swatson HK (2000) Mixture experiments: a severe test of the ability of a broiler chicken to make the right choice. British Poultry Science 41, 136-140.
9. Liu SY, Cadogan DJ, Péron A, Truong HH, Selle PH (2014a) Effects of phytase supplementation on growth performance, nutrient utilisation and digestive dynamics of starch and protein in broiler chickens offered maize-, sorghum- and wheat-based diets. Animal Feed Science and Technology 197, 164-175.
10. Liu SY, Truong HH, Selle PH (2014b) Whole grain feeding for chicken-meat production: Possible mechanisms driving enhanced energy utilisation and feed conversion Animal Production Science, http://dx.doi.org/10.1071/AN13417.
11. Mehri M, Davarpanah AA, Mirzaei HR (2012) Estimation of ideal ratios of methionine and threonine to lysine in starting broiler chicks using response surface methodology. Poultry Science 91, 771-777.
12. Mehri M (2014) Optimization of response surface and neural network models in conjugation with desirability function for estimation of nutritional needs of methionine, lysine, and threonine in broiler chickens. Poultry Science 93, 1862-1867.
13. Raubenheimer D, Simpson SJ (1993) The geometry of compensatory feeding in the locust. Animal Behaviour 45, 953-964.
14. Raubenheimer D (2011) Toward a quantitative nutritional ecology: the right-angled mixture triangle. Ecological Monographs 81, 407-427.
15. Ruohonen K, Simpson SJ, Raubenheimer D (2007) A new approach to diet optimisation: A re-analysis using European whitefish (Coregonus lavaretus). Aquaculture 267, 147-156.
16. Selle PH, Ravindran V, Caldwell RA, Bryden WL (2000) Phytate and phytase: consequences for protein utilisation. Nutrition Research Reviews 13, 255-278.
17. Simpson SJ, Raubenheimer D (1993) A multilevel analysis of feeding-behavior - the geometry of nutritional decisions. Philosophical Transactions of the Royal Society of London Series B-Biological Sciences 342, 381-402.
18. Simpson SJ, Raubenheimer D (2012) The nature of nutrition: A unifying framework from animal adaptation to human obesity. Princeton University Press: New Jersey.
19. Wilkinson SJ, Bradbury EJ, Thomson PC, Bedford MR, Cowieson AJ (2014) Nutritional geometry of calcium and phosphorus nutrition in broiler chicks. The effect of different dietary calcium and phosphorus concentrations and ratios on nutrient digestibility. Animal 8, 1080-1088.
20. Zheng ZJ, Huang Y, Wu RJ, Zhao LM, Wang CF, Zhang RJ (2014) Response surface optimization of enzymatic hydrolysis of duck blood corpuscle using commercial proteases. Poultry Science 93, 2641-2650.