Introduction
Wheat, maize and sorghum are the three most commonly used grains in the poultry industry worldwide (Liu et al., 2014). These cereal grains usually constitute a major proportion (60 - 70%) of the diet fed to broilers (Black et al., 2005). Although cereals represent a major source of energy for birds, there are wide variations in the energy and nutrient content of different species and cultivars of cereals (Choct & Hughes, 1999). Specifically, wheat is perceived as having a high feeding value for poultry, yet, in reality, some studies have indicated that there are extreme variations in the apparent metabolisable energy (AME) values of wheat-based diets in chickens (Choct, Hughes & Annison (1999) and references within). Sorghum, the other major cereal, presents a number of economic advantages over wheat, since it can be grown under drier conditions, and is usually less costly (Liu, Selle & Cowieson, 2013). Also, in comparison to wheat, sorghum is thought to have a more consistent, higher energy density (Selle et al., 2010).
It is recognised that diet is the major determinant of the composition of the gastrointestinal microbiota (Shakouri et al., 2009). A number of components in the diet, such as content of proteins, fats and carbohydrates, can influence the composition of the gut microbiota (Hübener, Vahjen & Simon, 2002; Rehman et al., 2007). There is a symbiotic relationship between gut microbiota and the host. The complex community of microorganisms, dominated by bacteria, regulates processes related to host metabolism and immunity (Round & Mazmanian, 2009; Tremaroli & Bäckhed, 2012). Thus, it is of interest to the poultry industry to understand the influence of the gut microbiota on the host's nutrient and energy use efficiency. For instance, Stanley et al. (2013) found an association between caecal microbiota composition and productivity in chickens, showing that the abundance of a number of caecal bacteria were correlated with high growth performance values. Thus, a better understanding of the beneficial or detrimental role of intestinal bacteria and how to influence their abundance could result in improvements in broiler performance.
Few studies have analysed the impact of different diets on the structure of the gut microbial community. Additionally, there is limited information on the influence of cereal-based diets on specific microbial populations that could be associated with the health and productivity of the birds (Shakouri et al., 2009). For instance, Torok et al. (2011) found a number of OTUs within the caecum and the ileum which were negatively or positively correlated with feed conversion ratio (FCR) values. Lunedo et al. (2014) found increased enterobacteria abundance in the ileum, consistent with the low feed conversion performance of sorghum-fed chickens. In a recent study, our group identified a number of caecal bacteria associated with desirable productivity outcomes on chickens fed with the same diet, and suggested that further investigation could lead to their application as probiotics to improve bird performance (Stanley et al., 2016). In this study, we aimed to expand this information by analysing changes in broiler performance and caecal bacteria across wheat- or sorghum-based diets, and identifying which specific bacteria were associated with parameters of productivity in each dietary treatment.
Materials and methods
Animal ethics
The Animal Ethics Committees of the University of Adelaide (Approval No.S-2011-218) and the Department of Primary Industries and Resources, South Australia (Approval No. 25/11) approved this study. All animal work was conducted in accordance with the national and international guidelines for animal welfare.
Animal trials
Newly-hatched male Cobb 500 broiler chickens from the Baiada Hatchery, Willaston, SA, Australia, were transferred to two floor pens (one for each experimental diet) with wood sawdust and shavings bedding material in an environmentally controlled experimental animal facility. Feed and water were supplied ad libitum throughout the experiment. Two commercially prepared starter diets were used in this study. One was based on wheat (500 g/kg) as the main cereal component. The other diet was formulated to the same nutrient specifications but contained sorghum (300 g/k) and wheat (300 g/kg). Each diet provided 230 g/kg crude protein and 12.55 MJ/kg as fed, and both contained monensin (65 ppm), nicarbazin (45 ppm) and zinc bacitracin (75 ppm). Both diets also contained commercial exogenous phytase and xylanase enzyme products. The diets were fed from day of hatch (day 0) until the end of the experiment on day 25.
On day 13, chicks (n = 48 for each dietary treatment) were transferred in pairs to 48 metabolism cages in a temperature controlled room (23-25 ºC). Initial placing in metabolic cages in pairs was done to minimise stress and allow the birds to adjust to cages. At day 15, birds were moved into individual cages. Individual caging allowed the precise assessment of individual feed intake, energy in feed, and unused energy remaining in faeces. The experimental design eliminated competition for feed and reduced behavioural issues affecting feed intake. Single bird caging and individual measurements and sampling were implemented in order to allow direct correlation of microbiota structure and productivity measurements on a bird by bird basis. Birds were euthanised and necropsied on day 25 and caecal contents were collected from each bird and immediately transferred to a -20 ºC freezer. Samples from all birds were analysed.
FCR was calculated as a ratio of feed eaten and weight gained. Thus birds with low FCR, that needed less feed per kg gained, were the most efficient in converting feed to mass. Gross energy (GE) was measured in feed and in faeces of each individual bird using a Parr isoperibol bomb calorimeter (Parr Instrument Company, Moline, IL). Apparent metabolisable energy (AME) in MJ/kg dry matter, was calculated as (AMEdiet D T(GEdiet x feed eaten) x (GEexcreta x dry excreta)U=feed eaten=dry diet content). Body weight gain (BWG) was calculated as [weight gain (g)/start weight (g)] and feed eaten (FE) was total amount of feed eaten during the 10 day measurement time period. All of the above measurements were taken from day 15 to day 25, during the time when single birds were housed in metabolic cages.
DNA preparation, PCR amplification of 16S rRNA gene sequences, and bioinformatic analysis
DNA was isolated using the method of Yu & Morrison (2004); briefly, raw DNA extracts were obtained by initial lysis of caecal content in lysis buffer at 70 ºC, followed by bead beating using a Precellys 24 instrument (Stretton Scientific Limited). This was followed by enzyme removal of RNA and protein and subsequent purification using Qiagen columns as per the supplier's instruction. The V1-V3 region of the 16S rRNA gene was amplified (forward primer (Lane, 1991), 50 AGAGTTTGATCCTGG 30; reverse primer W31 (Snell-Castro et al., 2005), 50 TTACCGCGGCTGCT 30) as detailed by Stanley et al. (2012). Pyrosequencing was performed using a Roche/454 FLX+ instrument and Titanium chemistry kits according to the manufacturer's instructions. Sff file processing was done using PyroBayes (Quinlan et al., 2008) and inspected for chimeric sequences using Pintail (Ashelford et al., 2005) and error corrected using Acacia (Bragg et al., 2012). Further trimming was done in QIIME (Caporaso et al., 2010) with sequence length 300-600 bases, no ambiguous sequences, minimum average quality score of 25 and maximum of 6 bases in homopolymer runs. OTU picking was done using Uclust (Edgar, 2010). Taxonomy was assigned using Blast against the GreenGenes database (DeSantis et al., 2006) and QIIME v.1.8 defaults. Additional taxonomic assignments for OTUs of interest was performed using EzTaxon database (Chun et al., 2007). The complete dataset is available on MG-RAST database (http://metagenomics.anl.gov/) under library ID mgl538406.
Statistical analysis
Permutational analysis of variance (PERMANOVA) (Anderson, 2001) was used to analyse differences between dietary treatments on each of the four performance measures (AME, FCR, BWG and FE) and also for differences in microbiota composition. The distance matrices were based on Euclidean distance (growth performance data) and both Weighted and Unweighted Unifrac distances (microbiota abundance data) (Lozupone & Knight, 2005). The PERMDISP routine was used to test for the homogeneity of dispersions (based on mean distance to group centroids), to ensure that dispersions were constant among groups (Anderson, 2006). Principal Coordinates Analysis (PCO) was used to visualise between-groups differences in microbiota composition, based on both Unifrac distances. Analysis of variance (ANOVA) was used to test for differences in the diversity of microbial community between dietary treatments using four diversity measures (i.e., evenness, richness, Shannon's and Inverted Simpson's indices). ANOVA was also used to test for differences in the abundance of individual microbial taxa between dietary treatments. Redundancy discrimination analysis (RDA) was used to analyse individual associations between microbiota community composition and each of the performance measures in both dietary treatments. Pearson's correlation tests were conducted to analyse the correlations between performance indicators. Spearman's correlation test was used to analyse correlations between individual OTUs and each of the performance indicators. For all ANOVA, RDA and correlation analyses, microbiota abundance data was normalised and square root transformed to reduce variance heterogeneity and increase predictive power. PERMANOVA, PERMDISP and PCO analyses were carried out using PRIMER software (v.6.1.16) with the PERMANOVA+add-on (v.1.0.6). RDA, Pearson's and Spearman's correlation tests, and analyses of community diversity and taxonomic structure were performed in Calypso (v.5.8) (Zakrzewski et al., 2016).
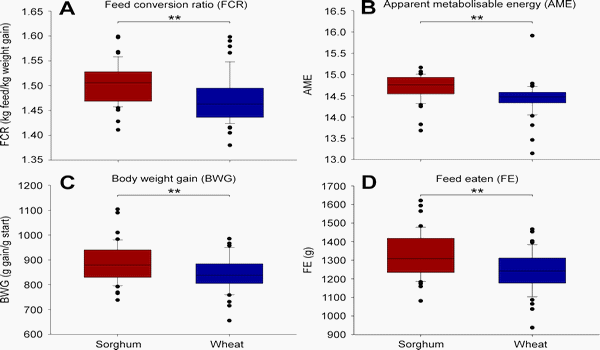
Figure 1.Boxplots showing differences in four growth performance measures between diet treatments. `Sorghum' refers to birds fed with a sorghum-based diet, whereas `wheat' refers to those fed with a wheat based diet. Significant differences between treatments are indicated (`**': P ≤ 0:01).
Results
Growth performance measures
Dietary treatment caused significant differences in all four performance measures (Fig. 1). Chickens fed with sorghum had significantly higher FCR values (1.50 ± 0.01 [average ± standard error]) than those fed with wheat (1.47 ± 0.01) (P = 0:004) (Fig. 1A). Additionally, AME values were significantly higher in sorghum-fed chickens (14.71 ± 0.04) than in wheat-fed birds (14.44 ± 0.06) (P = 0:002) (Fig. 1B). BWG values were also significantly higher in birds fed with sorghum (886 ± 12) than in those fed with wheat (843 ± 10) (P = 0:004) (Fig. 1C). Lastly, chickens had significantly higher FE values when fed with sorghum (1331 ± 17) than when fed with wheat (1240 ± 16) (P = 0:002) (Fig. 1D). PERMDISP results showed that none of the latter PERMANOVA results were significantly affected by data dispersion (all with P >0:05).
Pearson's correlation test results indicated that in each dietary treatment, AME was significantly negatively correlated with BWG (wheat: r = - 0:382, P = 0:010; sorghum: r =- 0:410, P = 0:005) and FE (wheat: r =- 0:408, P D 0:005; sorghum: r = -0:506, P ≤ 0:001). However, FCR and FE were significantly positively correlated in wheat-fed chickens (r =0:311, P =0:037), but not in sorghum-fed chickens (r =0:155, P =0:308).
Figure 2. Caecal microbiota profiles showing normalised square root transformed abundances of the families in sorghum-fed chickens (A) and wheat-fed chickens (B).
Structure of the caecal microbial community
The microbiota profile comparison at the family level indicated high similarities between dietary treatments, both dominated by Lactobacillaceae, Lachnospiraceae and Ruminococcaceae (Fig. 2).
None of the four selected diversity measures (i.e., richness, evenness, Shannon's and inverted Simpson's indices) varied significantly between dietary treatments, neither at the OTU level, nor at any higher taxonomic level (P >0:05). Comparisons of the microbiota composition at the OTU level revealed significant differences between treatments when Unweighted Unifrac Distances were used (Pseudo-F = 2:65, P = 0:006) (Fig. 3A), but not when Weighted Unifrac Distances were used (Pseudo-F =2:59, P =0:063) (Fig. 3B). PERMDISP confirmed that the significant result observed using Unweighted Unifrac Distances did not occur due to differences in data dispersion (P =0:640).
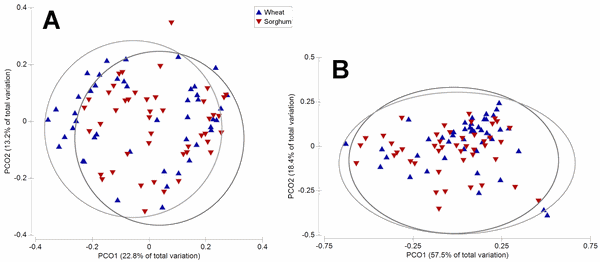
Figure 3. Principal Coordinates Analysis (PCO) showing a two-dimensional ordination of gut microbiota composition at the OTU level by dietary treatment (indicated by symbols). `(A)' plot is based on Unweighted Unifrac distance, whereas `(B)' plot is based on Weighted Unifrac distance. Overlayed grey ellipses in were created to help visualise the between-treatment differences in composition. Axes indicate the percentage of variation in the data.
Gut microbiota shifts induced by diet
Diet differences produced significant changes (P ≤ 0:05) in 98 out of 522 OTUs (18.8%). At the species level, sorghum-fed birds showed significantly higher abundances of a phylotype closest to Lactobacillus crispatus (identity assigned to 21 OTUs (n = 21), all DSM 20584(T) strains, with an averaged similarity (avg. sim.) of 92.67% [EzTaxon]) than wheat-fed birds (P ≤ 0:001) (Fig. 4A). Out of the 32 OTUs allocated to this species, OTU3 (with a similarity of 100%) was the most abundant and showed a similar trend as seen for the whole L. crispatus phylotype (P ≤ 0:001) (Fig. 4B). Showing an opposite trend, the numbers of a phylotypes most similar to Clostridium leptum (n = 30, all =SM753 (T), avg. sim. = 87:94%) were significantly higher in chickens fed with wheat than in those fed with sorghum (P =0:022) (Fig. 4A). At the genus level, wheat-fed birds showed significant lower abundances of Enterococcus and Coprococcus than sorghum-fed birds (P =0:016 and P D0:018, respectively) (Fig. 4C). Shifts in the Enterococcus genus (Fig. 4C) translated into equal changes at the family level (Enterococcaceae) (Fig. 4D). Also, the Lachnospiraceae family had significantly higher numbers in chickens fed with sorghum than in those fed with wheat (P =0:003). Additionally, two families, Clostridiales family XIII and Catabacteriaceae, showed significantly higher abundances in wheat-fed chickens than in sorghum-fed chickens (P =0:008 and P =0:012, respectively) (Fig. 4D). No other significant shifts were found at any other taxonomic level (P >0:05).
Figure 4.Caecal microbiota showing significant differences in normalised square root transformed abundance between dietary treatments (indicated by colours). `(A)', `(C)' and `(D)' show taxa at, respectively, the species, genus and family levels. `(B)' shows the shift in OTU3, which identity was assigned (using EzTaxon) to 100% L. crispatus DSM 20584(T) strain. Statistically significant differences are indicated (`*': P ≤ 0:05; `**': P ≤ 0:01; `***': P ≤ 0:001). L. crispatus phylotype was assigned to 21 OTUs, all DSM 20584(T) strains, with an averaged similarity (avg. sim.) of 92.67%. Clostridium leptum phylotype was assigned to 30 OTUs, all DSM 753(T) strains (avg. sim. =87:94%).
Associations between microbiota and performance measures
The RDA analysis showed that, at the OTU level in both dietary treatments, gut microbiota composition was significantly associated with AME (P =0:007), but not to FCR (P =0:206), BWG (P =0:156) or FE (P =0:061). Out of the 98 OTUs showing significant shifts between dietary treatments, 54 OTUs (53.02%) showed individual, significant correlations with at least one of the growth performance measures (Table 1). More than 50% of these OTUs (n=30) were significantly correlated withAMEvalues, and all the OTUs showing positive correlations (n=11) were significantly more abundant in sorghum-fed birds than in wheat-fed birds (Table 1). Particularly, four OTUs assigned to a phylotype closest to L. crispatus (OTUs 3, 221, 35 and 503) showed consistent positive correlations with AME values. Indeed, L. crispatus phylotypes were positively correlated with AME (p=0:268, P =0:011). On the contrary, all the OTUs showing negative correlations with AME (n = 19) were significantly more abundant in wheat-fed chickens. Most of these OTUs were assigned to either the genus Clostridium or the order Clostridiales (OTUs 401, 664, 299, 429, 127, 340, 244, 92, 261, 163, 16, 199, 342 and 148). Nevertheless, some other OTUs within the order Clostridiales were positively correlated with growth performance: nine OTUs assigned to this order showed significant, negative correlations with FCR values (OTUs 401, 127, 92, 261, 409, 550, 355, 682 and 416). Moreover, the family Clostridiaceae was positively correlated with AME values (p= 0:250, P = 0:017). Additionally, eight OTUs (assigned to various taxa) which were significantly prevalent in sorghum-fed birds, showed a significant positive correlation withBWGvalues (OTUs 123, 511, 162, 43, 54, 192, 321 and 9). At higher taxonomic levels, Lactobacillus helveticus was significantly negatively correlated with BWG (p=-0:210, P D 0:047). No other taxa at any other taxonomic level showed significant correlations with any of the growth performance measures.
Table 1 Correlation values based on Spearman's tests between OTUs showing significant shifts between dietary treatments and growth performance measures. p values were ordered from largest to smallest. The significant level of each correlation is indicated. Colours indicate whether a given OTU showed significantly higher mean abundance (normalised square root transformed values) in sorghum-fed birds (red) or wheat-fed birds (blue), and the significant level is indicated as `P (diet)'. Taxonomic assignment is based on the furthermost default QIIME taxonomy against GreenGenes database.
Discussion
Between-diet comparisons of growth performance
Overall, our results indicate that a sorghum-based diet increased AME, BWG and FE, but resulted in poorer FCR of chickens, compared to a wheat-based diet. These findings partially agree with other studies, showing that birds fed on a sorghum-based diet were more efficient in extracting energy from food (high AME values) (Black et al., 2005), and had higher BWG (Liu et al., 2015a; Selle et al., 2010; Torok et al., 2011) than those fed with wheat. However, contrary to our results, the latter studies found that sorghum-fed chickens had either better (Liu et al., 2015a; Selle et al., 2010; Torok et al., 2011) or similar feed conversion efficiency (Black et al., 2005) than wheat-fed chickens. Caecal microbiota produce short chain fatty acids which provide up to 10% of energy to the bird and additionally, caecal contents backflow seed the gut, both up and downstream, via reverse peristalsis or reflux of digesta (Sonnenburg & Backhed, 2016). These offer ways in which caecal microbiota are translocated into the intestinal tract thus affecting energy metabolism and performance both directly and indirectly.
Previous research indicated that chickens showing high AME values, tend to show low FCR values because of a low consumption of feed (Stanley et al., 2016). However, in our study, birds offered a sorghum-based diet ate more feed compared with those offered a wheat-based diet. These results may suggest a preference for sorghum- vs. wheat-based diets in chickens, in agreement with Liu et al. (2015a) and Liu et al. (2015b). Interestingly, only birds fed with a wheat-based diet showed a significant positive correlation between FE and FCR values (i.e., high feed intake leading to poor growth performance, or vice-versa). In either one or the other case, this correlation indicates that birds fed with wheat showed a trade-off between feed consumption and the ability to transform feed into body weight. Such a trade-off did not occur in sorghum-fed chickens, suggesting that their efficiency in converting feed to body mass was not compromised by the amount of feed they ate. Despite this advantage, birds fed on a sorghum-based diet showed comparatively higher FCR values than those fed on a wheat-based diet, indicating that energy in sorghum was used less efficiently than the energy in wheat. The issue of sorghum-based diets in the Australian poultry industry has been extensively reviewed (see Liu et al. (2015b) and references within), and some studies have linked sorghum-based diets to inconsistent or suboptimal broiler performance. These associations might have been produced by antinutritive factors present in sorghum (Selle, Liu & Cowieson, 2013). Particularly, kafirin concentrations in Australian sorghum cultivars might have increased as an accidental consequence of breeding programs. Also, these cultivars contain phenolic compounds (Liu et al., 2015b). These factors could have contributed to the lower utilisation of energy from sorghum-based diet relative to that from wheat-based diets found in this study. Despite this potential disadvantage, Liu et al. (2015b) encouraged to rectify these issues by using white sorghums with low kafirin levels. Our results support these findings based on the positive effects that this diet had on AME and BWG values.
A number of studies have suggested a range of supplements can be added to sorghum based diets in order to improve productivity. For instance, reductions in FCR performance of cereal-fed chickens could be improved by adding distillers dried grains with solubles (DDGS) (Jacobs & Parsons, 2013). Also, the latter study indicated that DDGS might, under certain conditions, have additional benefits by increasing AME, caecal microbiota, and gizzard size. Alternatively, Selle et al. (2016) showed that adding sodium metabisulphite and protease in sorghum-based diets enhanced starch utilisation, ultimately improving bird growth performance by decreasing FCR and increasing AME and BWG. These supplements could improve the feed conversion efficiency in sorghum-fed chickens.
Diet-induced changes in caecal microbiota and associations with growth performance
Diet has been recognised as a major modulator of intestinal microbiota. This conclusion has been mainly drawn from microbiota comparisons following the application of diets that are fundamentally very different, such as high fat vs. low fat or, even with more extreme differences, in plant-based vs. Western diet (Sonnenburg & Backhed, 2016). In these cases, the differences in microbiota are just as extreme as the differences between the diets. Here we compared two similar grain-based diets and although we did find some differences, they were not large as the differences in dietary compositions were not large either. Between-diet comparisons at the microbiota community level showed differences in composition, but not in diversity. Differences in composition were only detected when using Unweighted Unifrac Distance (presence-absence). These results suggest that differences in diet produced a subtle yet significant impact in the structure of the caecal microbial community, confirmed by between-diet shifts in abundance of less than 19% of all OTUs. This is in contrast with Torok et al. (2011), who reported significant differences in microbiota composition in the ileum, but not in the caecum of wheat-fed chickens and sorghum-fed chickens. Despite the low effect of diet on caecal microbiota composition found in our study, a number of caecal bacteria showed strong and contrasting correlations with the growth performance of the birds. Particularly, our results show that the abundance of 30 OTUs were correlated with AME values. These results were supported by the finding that, at the community level, microbiota composition was associated with AME values. Our results are consistent with previous studies since Torok et al. (2011) and Stanley et al. (2012) found associations between the composition of caecal microbiota and avian growth performance, at least in wheat-based diets.
Most of the microbiota in both dietary treatments belonged to the orders Lactobacillales and Clostridiales, in agreement with other studies (Torok et al., 2011). Particularly, high numbers of phylotypes assigned to Lactobacillus crispatus were recorded, yet these were much higher in sorghum-fed birds than in wheat-fed birds, indicating that the abundance of this species is partly influenced by diet-related differences. However, a study found that chickens fed with sorghum showed lower numbers of lactobacilli than chickens fed with wheat (Shakouri et al., 2009), in disagreement with our results. Generally, L. crispatus is considered beneficial because it produces hydrogen peroxide, tolerates gastric juice and bile salts, and has high surface hydrophobicity (Mota et al., 2006). This taxon, along with other strains, is involved in the reduction of pathogen loads, such as Salmonella species (Van der Wielen et al., 2002). Despite it being generally recognised as a beneficial bacterium, its associations with chicken performance have been mixed. Stanley et al. (2016) found that the abundance of L. crispatus was associated with high bird performance, since a number of OTUs assigned to this taxon showed either positive correlations with AME values or negative correlations with FCR values. Moreover, Mignon-Grasteau et al. (2015) indicated that L. crispatus was associated with growth performance, since they found significantly lower abundance in high-FCR than in low-FCR birds. Contrary to the latter two studies, bacterial phylotypes related to L. crispatus along with two other Lactobacillus species were linked to low avian growth performance (Torok et al., 2011). Also, Konsak et al. (2013) found that an OTU which was most closely related to L. crispatus, was more abundant in birds with low AME values. Nevertheless, our results reinforce those previously found by our group (Stanley et al., 2016), suggesting that the abundance of L. crispatus is an indicator of high productivity, and that certain strains of this species may enhance bird growth performance. Although the mechanisms behind this beneficial effect remain unexplained, we hypothesise that L. crispatus could increase energy availability through production of short-chained fatty acids. Our hypothesis is supported by Meimandipour et al. (2010), who showed the butyrogenic effects of lactobacilli strains in a simulated chicken caecum. These findings support the conclusion that some strains/OTUs most closely related to L. crispatus could be performance-related bacteria that could be studied as candidates to enhance productivity in poultry.
Additionally, sorghum-fed birds showed high abundance of another Lactobacillales member, the Enterococcus genus. This genus encompasses several species, with a few considered beneficial, such as E. faecium and E. faecalis (Devriese & Pot, 1995). Nevertheless, other species of Enterococcus found in the gastro-intestinal tract of chickens have been associated with infectious diseases, such as E. cecorum, related to osteomyelitis (Kense & Landman, 2011). One study associated the increased abundance of the Enterococcus genus and the Enterobacteriaceae family in the ileum with low feed conversion efficiency in sorghum-fed chickens (Lunedo et al., 2014). In our study, we did not observe any significant correlations between Enterococcus and FCR, but further research could clarify whether this genus is involved in the high FCR values observed in sorghum-fed birds.
Several taxa within the order Clostridiales showed different abundances between dietary treatments and contrasting correlations with growth performance measures. Particularly in this group, the Lachnospiraceae family was the most abundant taxon, showing higher numbers in sorghum- than in wheat-fed chickens. This family is a butyrate-producing group considered as beneficial plant degraders and strong producers of short chain fatty acids (Biddle et al., 2013), and its numbers are reduced in gastrointestinal illnesses such as chronic liver cirrhosis (Chen et al., 2011) and inflammatory bowel disease (Berry & Reinisch, 2013). Lachnospiraceae have been associated with improved growth performance in chickens (low FCR values) (Stanley et al., 2012). Furthermore, Torok et al. (2011) found 4 OTUs assigned to Lachnospiraceae associated with high performance (low FCR values), and 1 OTU assigned to the same taxon, associated with low performance (high FCR values) in the caecal microbiota of chickens. Our results support the latter two studies since one OTU assigned to this family was found to be positively correlated with BWG. Additionally, the Coprococcus genus was relatively more abundant in sorghum- than in wheat-fed chickens. One OTU assigned to this genus was positively correlated with BWG and FE, but another OTU was negatively correlated with FCR and AME, indicating mixed associations with growth performance. Previously, Stanley et al. (2016) found three different OTUs assigned to Coprococcus to be positively correlated with FCR, which indicates that this genus might have negative consequences for chicken growth performance.
Also, there were three bacterial taxa within the Clostridiales order showing higher abundance in wheat-fed than in sorghum-fed chickens: the families Catabacteriaceae and Clostridiales family XIII, and the Clostridium leptum phylotype. Catabacteriaceae, a relatively unknown family that has been found in polluted water (Codony et al., 2009), has been associated with poor feed conversion efficiency in chickens (Stanley et al., 2016). Also, although little is known about Clostridiales Family XIII, Singh et al. (2012) found Clostridiales Family XIII linked to broilers showing high FCR values. Additionally, Steelman et al. (2012) found significantly higher abundance of this taxon in horses with chronic laminitis than in healthy horses. In our study, we did not find any association between the three above-mentioned Clostridiales bacteria and chicken productivity. However, based on what other studies have reported, high abundance of these bacteria may result in poor bird performance. Future research efforts could study how differences in the proportions of cereals in feed influence the survival of these Clostridiales taxa in the chicken gut microbiota. Interestingly, a number of other bacteria within the Clostridiales order showed mixed associations with growth performance measures. Out of several OTUs (identified as either Clostridiales or Clostridium) which were prevalent in wheat-fed chickens, some showed negative correlations with AME, whereas others showed negative correlations with FCR. Such inconsistency of patterns might have been produced because Clostridiales is a high polyphyletic order. There seems to be some ambiguity in the taxonomy of several Clostridium species (Yutin & Galperin, 2013) despite Biddle et al. (2013) recommending major revisions. Species within this genus are a mixture of gram positive and negative bacteria, and some are not even anaerobes (Fåk & Bäckhed, 2012). Our results, in accordance with Stanley et al. (2016), suggest that some members of the Clostridium genus (from the Lachnospiraceae family) could be associated with better performance, whereas a number of other unidentified taxa in this order could be associated with low performance. Thus, in agreement with other studies (Rinttilä & Apajalahti, 2013; Stanley et al., 2016), the term clostridia should not be related with poor performance and/or health in chickens and beneficial and detrimental clostridia should be clearly distinguished.
Conclusions
In summary, our results suggest that differences in growth performance depending on cereal type used in the diet were correlated with changes in specific members of the microbiota in the caecum of chickens. Also, our study reinforces the hypothesis that specific bacteria could be used as probiotics to improve performance, given the associations of the caecal microbiota and growth performance measures that we found. Future studies could test this hypothesis in order to promote long-term sustainability for the poultry industry.
Acknowledgements
The data was analysed using the Isaac Newton High Performance Computing System at Central Queensland University. We wish to acknowledge the support from Jason Bell in all aspects of high performance computing. We also thank Derek Schultz, Evelyn Daniels and Kylee Swanson (SARDI) for their assistance with animal trials, and Honglei Chen (CSIRO) for operating the Roche 454 sequencer.
Additional infirmation and declarations
Funding
This research was conducted within the Poultry CRC, established and supported under the Australian Government's Cooperative Research Centres Program under project CRC 2.1.5. DS is ARC DECRA Fellow. The funders had no role in study design, data collection and analysis, decision to publish, or preparation of the manuscript.
Grant Disclosures
The following grant information was disclosed by the authors: Australian Government's Cooperative Research Centres Program.
Competing Interests
The authors declare there are no competing interests.
Author Contributions
- Eduardo Crisol-Martínez analyzed the data, wrote the paper, prepared figures and/or tables, reviewed drafts of the paper.
- Dragana Stanley and Robert J. Moore conceived and designed the experiments, analyzed the data, contributed reagents/materials/analysis tools, reviewed drafts of the paper.
- Mark S. Geier and Robert J. Hughes conceived and designed the experiments, performed the experiments, contributed reagents/materials/analysis tools, reviewed drafts of the paper.
Animal Ethics
The following information was supplied relating to ethical approvals (i.e., approving body and any reference numbers):
The Animal Ethics Committees of the University of Adelaide (Approval No.S-2011-218) and the Department of Primary Industries and Resources, South Australia (Approval No. 25/11) approved this study. All animal work was conducted in accordance with the national and international guidelines for animal welfare.
Data Availability
The following information was supplied regarding data availability:
The complete dataset is available on the MG-RAST database (http://metagenomics.anl. gov/mgmain.html?mgpage=project&project=mgp20398) under library ID mgl538406.
References
Anderson MJ. 2001. A new method for non-parametric multivariate analysis of variance. Austral Ecology 26(1):32_46 DOI 10.1111/j.1442-9993.2001.01070.pp.x.
Anderson MJ. 2006. Distance-based tests for homogeneity of multivariate dispersions. Biometrics 62:245_253 DOI 10.1111/j.1541-0420.2005.00440.x.
Ashelford KE, Chuzhanova NA, Fry JC, Jones AJ, Weightman AJ. 2005. At least 1 in 20 16S rRNA sequence records currently held in public repositories is estimated to contain substantial anomalies. Applied and Environmental Microbiology 71:7724_7736 DOI 10.1128/AEM.71.12.7724-7736.2005.
Berry D, Reinisch W. 2013. Intestinal microbiota: a source of novel biomarkers in inflammatory bowel diseases? Best Practice & Research Clinical Gastroenterology 27:47_58 DOI 10.1016/j.bpg.2013.03.005.
Biddle A, Stewart L, Blanchard J, Leschine S. 2013. Untangling the genetic basis of fibrolytic specialization by Lachnospiraceae and Ruminococcaceae in diverse gut communities. Diversity 5:627_640 DOI 10.3390/d5030627.
Black J, Hughes R, Nielsen S, Tredrea A, Macalpine R, Van Barneveld R. 2005. The energy value of cereal grains, particularly wheat and sorghum, for poultry. Proceedings of the Australian Poultry Science Symposium 17:21_29.
Bragg L, Stone G, Imelfort M, Hugenholtz P, Tyson GW. 2012. Fast, accurate errorcorrection of amplicon pyrosequences using Acacia. Nature Methods 9:425_426 DOI 10.1038/nmeth.1990.
Caporaso JG, Kuczynski J, Stombaugh J, Bittinger K, Bushman FD, Costello EK, Fierer N, Pena AG, Goodrich JK, Gordon JI. 2010. QIIME allows analysis of highthroughput community sequencing data. Nature Methods 7:335_336 DOI 10.1038/nmeth.f.303.
Chen Y, Yang F, Lu H, Wang B, Chen Y, Lei D, Wang Y, Zhu B, Li L. 2011. Characterization of fecal microbial communities in patients with liver cirrhosis. Hepatology 54:562_572 DOI 10.1002/hep.24423.
Choct M, Hughes R. 1999. Chemical and physical characteristics of grains related to variability in energy and amino acid availability in poultry. Crop and Pasture Science 50:689_702 DOI 10.1071/AR98161.
Choct M, Hughes R, Annison G. 1999. Apparent metabolisable energy and chemical composition of Australian wheat in relation to environmental factors. Australian Journal of Agricultural Research 50:447_451 DOI 10.1071/A98155.
Chun J, Lee J-H, Jung Y, Kim M, Kim S, Kim BK, Lim Y-W. 2007. EzTaxon: a webbased tool for the identification of prokaryotes based on 16S ribosomal RNA gene sequences. International Journal of Systematic and Evolutionary Microbiology 57:2259_2261 DOI 10.1099/ijs.0.64915-0.
Codony F, Adrados B, Pérez LM, Fittipaldi M, Morató J. 2009. Detection of Catabacter hongkongensis in polluted European water samples. Journal of Zhejiang University Science B 10:867_869 DOI 10.1631/jzus.B0920218.
DeSantis TZ, Hugenholtz P, Larsen N, Rojas M, Brodie EL, Keller K, Huber T, Dalevi D, Hu P, Andersen GL. 2006. Greengenes, a chimera-checked 16S rRNA gene database and workbench compatible with ARB. Applied and Environmental Micro- biology 72:5069_5072 DOI 10.1128/AEM.03006-05.
Devriese L, Pot B. 1995. The genus Enterococcus. In: Wood BJB, Holzapfel WH, eds. The genera of lactic acid bacteria. New York: Springer, 327_367.
Edgar RC. 2010. Search and clustering orders of magnitude faster than BLAST. Bioinfor- matics 26:2460_2461 DOI 10.1093/bioinformatics/btq461.
Fåk F, Bäckhed F. 2012. Lactobacillus reuteri prevents diet-induced obesity, but not atherosclerosis, in a strain dependent fashion in Apoe ??/ ?? mice. PLOS ONE 7:e46837 DOI 10.1371/journal.pone.0046837.
Hübener K, Vahjen W, Simon O. 2002. Bacterial responses to different dietary cereal types and xylanase supplementation in the intestine of broiler chicken. Archives of Animal Nutrition 56:167_187.
Jacobs C, Parsons CM. 2013. The effects of coarse ground corn, whole sorghum, and a prebiotic on growth performance, nutrient digestibility, and cecal microbial populations in broilers fed diets with and without corn distillers dried grains with solubles. Poultry Science 92:2347_2357 DOI 10.3382/ps.2012-02928.
Kense M, Landman WJ. 2011. Enterococcus cecorum infections in broiler breeders and their offspring: molecular epidemiology. Avian Pathology 40:603_612 DOI 10.1080/03079457.2011.619165.
Konsak BM, Stanley D, Haring VR, Geier MS, Hughes RJ, Howarth GS, Crowley TM, Moore RJ. 2013. Identification of differential duodenal gene expression levels and microbiota abundance correlated with differences in energy utilisation in chickens. Animal Production Science 53:1269_1275 DOI 10.1071/AN12426.
Lane D. 1991. 16S/23S rRNA sequencing. In: Stackebrandt E, Goodfellow M, eds. Nucleic acid techniques in bacterial systematics. New York: John Wiley and Sons, 115_175.
Liu S, Cadogan D, Péron A, Truong H, Selle P. 2014. Effects of phytase supplementation on growth performance, nutrient utilization and digestive dynamics of starch and protein in broiler chickens offered maize-, sorghum- and wheat-based diets. Animal Feed Science and Technology 197:164_175 DOI 10.1016/j.anifeedsci.2014.08.005.
Liu S, Cadogan D, Péron A, Truong H, Selle P. 2015a. A combination of xylanase, amylase and protease influences growth performance, nutrient utilisation, starch and protein digestive dynamics in broiler chickens offered maize-, sorghum-and wheatbased diets. Animal Production Science 55:1255_1263 DOI 10.1071/AN14657.
Liu SY, Fox G, Khoddami A, Neilson KA, Truong HH, Moss AF, Selle PH. 2015b. Grain sorghum: a conundrum for chicken-meat production. Agriculture 5:1224_1251 DOI 10.3390/agriculture5041224.
Liu SY, Selle PH, Cowieson AJ. 2013. Strategies to enhance the performance of pigs and poultry on sorghum-based diets. Animal Feed Science and Technology 181:1_14 DOI 10.1016/j.anifeedsci.2013.01.008.
Lozupone C, Knight R. 2005. UniFrac: a new phylogenetic method for comparing microbial communities. Applied and Environmental Microbiology 71:8228_8235 DOI 10.1128/AEM.71.12.8228-8235.2005.
Lunedo R, Fernandez-Alarcon M, Carvalho F, Furlan L, Macari M. 2014. Analysis of the intestinal bacterial microbiota in maize-or sorghum-fed broiler chickens using realtime PCR. British Poultry Science 55:795_803 DOI 10.1080/00071668.2014.975781.
Meimandipour A, Shuhaimi M, Soleimani AF, Azhar K, Hair-Bejo M, Kabeir BM, Javanmard A, Anas OM, Yazid AM. 2010. Selected microbial groups and short-chain fatty acids profile in a simulated chicken cecum supplemented with two strains of Lactobacillus. Poultry Science 89:470_476 DOI 10.3382/ps.2009-00495.
Mignon-Grasteau S, Narcy A, Rideau N, Chantry-Darmon C, Boscher M-Y, Sellier N, Chabault M, Konsak-Ilievski B, Le Bihan-Duval E, Gabriel I. 2015. Impact of selection for digestive efficiency on microbiota composition in the chicken. PLOS ONE 10:e0135488 DOI 10.1371/journal.pone.0135488.
Mota RM, Moreira JLS, Souza MR, Horta MF, Teixeira SM, Neumann E, Nicoli JR, Nunes ÁC. 2006. Genetic transformation of novel isolates of chicken Lactobacillus bearing probiotic features for expression of heterologous proteins: a tool to develop live oral vaccines. BMC Biotechnology 6:1 DOI 10.1186/1472-6750-6-1.
Quinlan AR, Stewart DA, Strömberg MP, Marth GT. 2008. Pyrobayes: an improved base caller for SNP discovery in pyrosequences. Nature Methods 5:179_181 DOI 10.1038/nmeth.1172.
Rehman HU, Vahjen W, Awad WA, Zentek J. 2007. Indigenous bacteria and bacterial metabolic products in the gastrointestinal tract of broiler chickens. Archives of Animal Nutrition 61:319_335 DOI 10.1080/17450390701556817.
Rinttilä T, Apajalahti J. 2013. Intestinal microbiota and metabolites_implications for broiler chicken health and performance1. The Journal of Applied Poultry Research 22:647_658 DOI 10.3382/japr.2013-00742.
Round JL, Mazmanian SK. 2009. The gut microbiota shapes intestinal immune responses during health and disease. Nature Reviews Immunology 9:313_323 DOI 10.1038/nri2515.
Selle P, Cadogan D, Ru Y, Partridge G. 2010. Impact of exogenous enzymes in sorghumor wheat-based broiler diets on nutrient utilization and growth performance. International Journal of Poultry Science 9:53_58 DOI 10.3923/ijps.2010.53.58.
Selle P, Liu S, Cowieson A. 2013. Sorghum: An enigmatic grain for chicken-meat production. In: Parra PC, ed. Sorghum: production, growth habits and health benefits. Hauppauge: Nova Publishers Inc, 1_44.
Selle PH, Truong HH, McQuade LR, Moss AF, Liu SY. 2016. Reducing agent and exogenous protease additions, individually and in combination, to wheat- and sorghum-based diets interactively influence parameters of nutrient utilisation and digestive dynamics in broiler chickens. Animal Nutrition 2(4):303_311 DOI 10.1016/j.aninu.2016.08.001.
Shakouri M, Iji P, Mikkelsen L, Cowieson A. 2009. Intestinal function and gut microflora of broiler chickens as influenced by cereal grains and microbial enzyme supplementation. Journal of Animal Physiology and Animal Nutrition 93:647_658 DOI 10.1111/j.1439-0396.2008.00852.x.
Singh K, Shah T, Deshpande S, Jakhesara S, Koringa P, Rank D, Joshi C. 2012. High through put 16S rRNA gene-based pyrosequencing analysis of the fecal microbiota of high FCR and low FCR broiler growers. Molecular Biology Reports 39:10595_10602 DOI 10.1007/s11033-012-1947-7.
Snell-Castro R, Godon J-J, Delgenès J-P, Dabert P. 2005. Characterisation of the microbial diversity in a pig manure storage pit using small subunit rDNA sequence analysis. FEMS Microbiology Ecology 52:229_242 DOI 10.1016/j.femsec.2004.11.016.
Sonnenburg JL, Backhed F. 2016. Diet-microbiota interactions as moderators of human metabolism. Nature 535:56_64 DOI 10.1038/nature18846.
Stanley D, Denman SE, Hughes RJ, Geier MS, Crowley TM, Chen H, Haring VR, Moore RJ. 2012. Intestinal microbiota associated with differential feed conversion efficiency in chickens. Applied Microbiology and Biotechnology 96:1361_1369 DOI 10.1007/s00253-011-3847-5.
Stanley D, Geier MS, Denman SE, Haring VR, Crowley TM, Hughes RJ, Moore RJ. 2013. Identification of chicken intestinal microbiota correlated with the efficiency of energy extraction from feed. Veterinary Microbiology 164:85_92 DOI 10.1016/j.vetmic.2013.01.030.
Stanley D, Hughes RJ, Geier MS, Moore RJ. 2016. Bacteria within the gastrointestinal tract microbiota correlated with improved growth and feed conversion: challenges presented for the identification of performance enhancing probiotic bacteria. Frontiers in Microbiology 7:187 DOI 10.3389/fmicb.2016.00187.
Steelman SM, Chowdhary BP, Dowd S, Suchodolski J, Jane£ka JE. 2012. Pyrosequencing of 16S rRNA genes in fecal samples reveals high diversity of hindgut microflora in horses and potential links to chronic laminitis. BMC Veterinary Research 8:1 DOI 10.1186/1746-6148-8-1.
Torok VA, Hughes RJ, Mikkelsen LL, Perez-Maldonado R, Balding K, Macalpine R, Percy NJ, Ophel-Keller K. 2011. Identification and characterization of potential performance-related gut microbiotas in broiler chickens across various feeding trials. Applied and Environmental Microbiology 77:5868_5878 DOI 10.1128/AEM.00165-11.
Tremaroli V, Bäckhed F. 2012. Functional interactions between the gut microbiota and host metabolism. Nature 489:242_249 DOI 10.1038/nature11552.
Van der Wielen PW, Lipman LJ, Van Knapen F, Biesterveld S. 2002. Competitive exclusion of Salmonella enterica serovar Enteritidis by Lactobacillus crispatus and Clostridium lactatifermentans in a sequencing fed-batch culture. Applied and Environmental Microbiology 68:555_559 DOI 10.1128/AEM.68.2.555-559.2002.
Yu Z, Morrison M. 2004. Improved extraction of PCR-quality community DNA from digesta and fecal samples. Biotechniques 36:808_813.
Yutin N, Galperin MY. 2013. A genomic update on clostridial phylogeny: gramnegative spore formers and other misplaced clostridia. Environmental Microbiology 15:2631_2641 DOI 10.1111/1462-2920.12173.
Zakrzewski M, Proietti C, Ellis JJ, Hasan S, Brion MJ, Berger B, Krause L. 2016. Calypso: a user-friendly web-server for mining and visualizing microbiomeenvironment interactions. Bioinformatics.