ABSTRACT
The high cost of feed ingredients, the use of non-renewable sources of phosphate and the dramatic increase in the environmental load resulting from the excessive land application of manure are major challenges for the livestock industry. Precision feeding is proposed as an essential approach to improve the utilization of dietary nitrogen, phosphorus and other nutrients and thus reduce feeding costs and nutrient excretion. Precision feeding requires accurate knowledge of the nutritional value of feedstuffs and animal nutrient requirements, the formulation of diets in accordance with environmental constraints, and the gradual adjustment of the dietary nutrient supply to match the requirements of the animals. After the nutritional potential of feed ingredients has been precisely determined and has been improved by the addition of enzymes (e.g. phytases) or feed treatments, the addition of environmental objectives to the traditional feed formulation algorithms can promote the sustainability of the swine industry by reducing nutrient excretion in swine operations with small increases in feeding costs. Increasing the number of feeding phases can also contribute to significant reductions in nutrient excretion and feeding costs. However, the use of precision feeding techniques in which pigs are fed individually with daily tailored diets can further improve the efficiency with which pigs utilize dietary nutrients. Precision feeding involves the use of feeding techniques that allow the provision of the right amount of feed with the right composition at the right time to each pig in the herd. Using this approach, it has been estimated that feeding costs can be reduced by more than 10%, and nitrogen and phosphorus excretion can both be reduced by more than 38%. Moreover, the integration of precision feeding techniques into large-group production systems can provide real-time off-farm monitoring of feed and animals for optimal slaughter and production strategies, thus improving the environmental sustainability of pork production, animal well-being and meat-product quality. Current and future research will lead to the development of innovative mathematical models that accurately estimate daily individual nutrient requirements, the proper calibration of these models in commercial conditions, and the evaluation of the practical feeding and nutritional improvements provided by this feeding approach as well as its economic and environmental feasibility.
INTRODUCTION
In industrial and semi-industrial swine production areas, feed costs represent more than 60% of the costs of production. In growing-finishing pig production systems, feeding programs are proposed to maximize population responses at minimal feed costs. However, nutrient requirements vary greatly between the pigs of a given population (Brossard et al., 2009; Pomar, 2007) and for each pig over time following individual patterns. In order to maximize the desired population response, which is usually body weight gain, population requirements are associated with those of the most demanding pigs, with the result that most of the pigs receive more nutrients than they need and the efficiency of dietary nutrient utilization is reduced (Jean dit Bailleul et al., 2000; Hauschild et al., 2010). This is because, for most nutrients, underfed pigs will exhibit reduced growth performance and overfed ones will exhibit near optimal performance. Given that unutilized nutrients are excreted via the urine or feces, feeding pigs to maximize population responses is associated with high feeding costs and high levels of nutrient excretion.
Reducing feed costs, the excretion of excess nutrients such as nitrogen (N) and phosphorus (P), and the use of non-renewable resources is essential to the development of sustainable pig production systems (Honeyman, 1996; Rotz, 2004; Jondreville and Dourmad, 2005). The excretion of N and P is affected mainly by the amount of N and P ingested, the metabolic availability of those nutrients, and the balance between dietary nutrient supply and the animals' requirements (Jongbloed and Lenis, 1992). If feeding costs and nutrient excretion are to be minimized, it is essential that the composition of the available feed ingredients, their nutritional potential and the animals' requirements be properly characterized and that the supply of dietary nutrients be accurately adjusted to match the requirements of the animals. For the feeding of a population, however, optimal feed composition is difficult to estimate, as the response of the population to rising concentrations of nutrients is affected by many factors, including genetics, gender and the environment as well as the variability between the individuals of the population to be fed. Optimal nutrient concentrations should then be estimated, given that feeds are provided to heterogeneous populations over long periods (Leclercq and Beaumont, 2000; Pomar et al., 2003; Brossard et al., 2007). Feeding pigs individually according to their daily requirements is therefore proposed for reducing feeding cost and improving nutrient efficiency by reducing excesses of the most economically and environmentally detrimental nutrients without jeopardizing animal performance. The objective of this paper is to describe the implementation of precision feeding techniques in growing-finishing pig facilities by briefly describing each of these elements and the potential improvement in the utilization efficiency of dietary protein and P. We will especially focus on the use of new tools to automatically feed daily tailored diets to group-raised animals and the potential of this approach in growing-finishing pig operations.
THE PRECISION FEEDING APPROACH
Precision farming or precision agriculture is an agricultural concept that relies on the existence of in-field variability. This concept is about doing the right thing, in the right place, in the right way, at the right time. Precision feeding is based on the fact that animals within a herd differ from each other in terms of age, weight and production potential and therefore each have different nutrient requirements. Precision feeding involves the use of feeding techniques that allow the right amount of feed with the right composition to be provided at the right time to each pig in the herd. Essential elements for precision feeding in livestock production systems include,
1) the precise evaluation of the nutritional potential of feed ingredients,
2) the precise determination of nutrient requirements,
3) the formulation of balanced diets that limit the amount of excess nutrients, and
4) the concomitant adjustment of the dietary supply and concentration of nutrients to match the evaluated requirements of each pig in the herd.
Evaluation of the nutritional potential of feed ingredients. Energy, amino acids, minerals, vitamins and water are essential nutrient constituents of feeds that need to be supplied to producing animals for body maintenance, growth, reproduction and lactation. Body growth results from the synthesis of muscle, adipose tissue, bone, hair, skin and other body components and depends on an adequate supply of dietary nutrients. Growing pigs must be provided with these essential nutrients in adequate amounts and in forms that are palatable and efficiently utilized for optimal growth.
Determination of the nutritive value of dietary ingredients for livestock is a difficult and tedious task. Not long ago, total chemical nutrient content was used to characterize the nutritional potential of dietary ingredients in livestock feeds. Animal digestion and metabolism studies revealed the principles of nutrient sources and the relationship between chemical composition and absorbed nutrient value (Johnson, 2007). Over time, new technologies for nutrition research have been developed to more precisely quantify the nutrient fractions of feed ingredients that are available to the animals' metabolism for maintenance and production purposes. The progression of the characterization of the nutritional potential of feed ingredients and animal requirements from a total to a digestible basis, and then to an available or net basis, allows for the formulation of diets with nutrient levels that are closer to the animals' requirements without the use of excessive safety margins. The advantages and limitations of different evaluation methods were presented in previous studies for energy (Noblet et al., 2003), amino acids (Stein et al., 2007) and P (Jondreville and Dourmad, 2005). However, although the careful assessment of the bioavailability of each dietary nutrient is critical for evaluating the nutritional value of feed ingredients for pigs and for estimating the animals' requirements, challenges remain to be overcome in the future, namely the use of bioavailability estimation methods that are accurate and easy to use in practice, that yield values that are additive in mixtures of feed ingredients, and that take into account the need for consistency in the bases used for expressing bioavailability in feed ingredients and requirements in animals (Stein et al., 2007). Additionally, the nutritional value of feed ingredients is not based only on the nutrient composition of the ingredients but also on the metabolic fate of these nutrients in the animal (Noblet and van Milgen, 2004). Grinding, heat treatment and the addition of enzymes are also important factors that should be taken into account when assessing the potential of nutritious foods. The precise evaluation of the nutritional potential of feed ingredients is required for livestock precision feeding.
Precise estimation of nutrient requirements. For specific nutrients (e.g. essential amino acids), and when all other nutrients are provided at adequate levels, nutrient requirements are defined as the amounts of nutrients needed for specified production purposes, which in farm animals are production outputs such as growth rate, protein deposition, milk yield, etc. (Fuller, 2004). Depending on the production purpose and the nutrient, the required nutrient amount can be considered the minimum amount that, when all other nutrients are given at adequate levels, will prevent signs of deficiency and allow the animal to perform its needed functions in a normal manner (Lassister and Edwards, 1982). In farm animals, nutrient requirements are affected by factors related to the animal (e.g. genetic potential, age, weight and sex), the feed (e.g. nutrient composition, digestibility and anti-nutritional factors) and the environment (e.g. temperature and space allowance) (Noblet and Quiniou 1999). When applied to pig populations, nutrient requirements are defined as the amount of nutrients needed for specified production purposes such as optimal growth rate and protein deposition (Hauschild et al., 2010). Furthermore, this definition should be considered in the context of feeds provided to heterogeneous populations over long periods (Ferguson et al., 1997; Knap, 2000; Leclercq and Beaumont, 2000; Pomar et al., 2003).
In practice, two methods are used to estimate the nutrient requirements of domestic growing animals: empirical and factorial. In the empirical method, nutrient requirements are those that either maximize or minimize one or several sets of performance parameters (e.g. growth rate) during a determined period. In the factorial method, however, daily requirements are estimated as the sum of the requirements for maintenance and production (Fuller and Chamberlain, 1982). These requirements are estimated for each nutrient or its precursor and take into account the efficiency with which each nutrient is used for each metabolic function (van Milgen and Noblet, 2003). Ultimately, both methods of estimating nutrient requirements are based on experimental results from the study of the relationship between nutrient intake and animal response. In the empirical method, this relationship is used to estimate the optimal response to varying nutrient levels of a population of animals showing some degree of heterogeneity. In contrast, the factorial method estimates, for a unique animal at one specific growing state, the requirements at zero production and those allowing maximal growth. Thus, when the factorial method is used to estimate the requirements of a given population, the chosen individual should be the best representative of the population (Pomar et al., 2003; Hauschild et al., 2010). The empirical method estimates optimal nutrient allowances from a population perspective, whereas the factorial method addresses the needs of one reference animal during a very short period, normally one day (Pomar, 2007). The relationship between the empirical and factorial methods is difficult to establish (Figure 1; Haulschild et al., 2010) and is affected by many factors related to the animal, the growth state and population heterogeneity.
Figure 1. Cumulative distribution of requirements estimated by the factorial method (○) and effect of different lysine-to-net energy (Lys:NE) ratios on weight gain estimated by the empirical method (▬) for a live-weight interval from 24 to 54 kg (According to Hauschild et al., 2010)
Mechanistic mathematical models that implement the factorial approach are proposed because of the complexity of animal responses and the numerous factors modulating them and thus the optimal level of nutrients that will optimize production systems (NRC, 1998; van Milgen et al., 2008; van Milgen et al., 2009). These models must, however, be properly calibrated to allow accurate estimates of the nutrient amounts that will maximize animal growth while minimizing nutrient excesses and excretion. An important feature of the modeling approach is the enhancement of the concept of nutrient requirements by a description of the animal responses to nutrient intake. These models can take into account the interactions between nutrients and the animal and thus contrast with conventional systems with fixed, tabulated "values-needs". However, such models are challenged with complex problems, as follows:
• Animals may follow different consumption and growth paths from the ones observed in the reference population even if they have similar genetics and are raised in equivalent farms and under apparently equivalent environmental and health conditions;
• Animals are raised in heterogeneous groups in terms of genetics, sex, healthiness or husbandry practices; and
• Animals are fed in heterogeneous groups according to phase-feeding programs of varying length.
Different mathematical models have been developed to simulate the growth of a pig that, in standard production conditions, can be considered the best representative of the population (Whittemore and Fawcett, 1976 ; Black et al, 1986 ; Moughan et al, 1987 ; Pomar et al, 1991 ; NRC, 1998 ; Ferguson et al, 1994 ; Knap, 1999 ; Birkett and de Lange 2001; Wellock et al, 2004; van Milgen et al, 2008). These models simulate growth or estimate the requirements of a given nutrient based on the information collected from similar populations. This information is used to set, a priori, the model parameters that will characterize the best representative of that population and will remain unchanged during the simulation. Therefore, model users have to be very careful to identify any differences that may exist between the reference and the target populations as well as any changes in the evolution of this target population during growth.
One often-forgotten aspect of animal production is that, in a given environment, the response of a population to nutrient intake depends on the response of each individual animal within the population. It is difficult to translate individual animal responses into average population responses, because individual and population responses differ in form and magnitude (Pomar, 1995; Pomar et al., 2003; Wellock et al., 2004), and because these differences increase with the heterogeneity of the populations (Pomar et al., 2003; Brossard et al., 2006). This suggests that population variability needs to be addressed in the interpretation of population responses, along with the underlying biological mechanisms, in order to identify general laws governing animal responses and determine the optimal nutrient levels required for optimal growth (Leclercq and Beaumont, 2000). In this context, we need to review and identify the type of mathematical models that should be developed and the level of aggregation of these models, so that we can simulate the response of groups of animals in order to optimize nutrient levels for livestock management. New approaches are being proposed today to characterize the individual pigs within a population, with consideration given to the relationships between model parameters (Brossard et al., 2006). Once those relationships (i.e. the variance-covariance matrix) have been established, this information can be used to generate virtual populations of animals based on the average pig profile of the population. Then, the random variation between these model parameters may be added to other model parameters to simulate the population. This approach helps with finding the best time to change the diet from economic and environmental perspectives, with identifying the optimal slaughter strategy, and with determining the input of nutrients that optimizes a factor of production for the population (van Milgen et al., 2009). These methods optimize population responses when pigs are fed a unique feed during given periods and assume that actual population performance is the same as that of the reference population. In this context, therefore, low-performing pigs would be fed diets with higher nutrient concentrations than they need for optimal growth and, at the same time, some highly demanding pigs would be underfed.
Formulation of balanced feeds that reduce nutrient excretion. Formulating a complete feed in an animal production system involves determining a mix of ingredients that meets specific nutrient requirements in accordance with the production objectives (Patience et al., 1995). An important step in diet formulation consists of determining the amount of nutrients that each ingredient will supply to the animal's metabolism as well as the amount needed by the animal to reach the desired production level. Linear programming is without a doubt the most widely used approach for diet formulation (Patience et al., 1995) and involves determining the level of incorporation of the available ingredients that, by respecting a series of linear constraints, will minimize (or maximize) an objective function, typically the cost of the mix. From a nutritional standpoint, all formulation methods assume that there is no ideal diet relative to the ingredients used. The ingredients are therefore selected on the basis of their availability, composition and cost (Patience et al., 1995; NRC, 1998; Sauvant et al., 2004). Two complete feeds are considered equivalent if they satisfy all the imposed constraints.
The least-cost feed formulation methods generally used by the industry try to minimize the cost of the feed mix, without taking into account the environmental consequences of excess nutrients (Patience et al., 1995) and animal responses (Sauvant, 1992). Thus, a feed formula may be nutritionally adequate and economically optimal but may still provide significant amounts of excess and unavailable nutrients. Unfortunately, reducing excess nutrients in feed is often considered a complex and costly task that adversely affects competitiveness. Feed formulation designed to minimize excess nutrients and unavailable fractions is, however, an essential step in reducing nutrient excretion (Le Bellego and Noblet, 2002). To solve this problem, a multi-objective optimization method based on the traditional least-cost formulation program was developed to reduce feed cost and total N (Jean dit Bailleul et al., 2001) and P (Pomar et al., 2007a) content in pig feeds. In the traditional feed formulation method, the objective function is defined in order to minimize the cost of the final formula, as follows:

where
C is the ingredient mix cost per unit weight (e.g. $/t), c
i represents the price per unit weight of the i
th ingredient, and x
i represents the amount of the i
th ingredient in the diet. The main characteristics of this model are the consequences of the linear nature of the objective function and constraints, requiring verification of the following assumptions (Wilton et al., 1974):
• Additivity - the value of the objective function is the sum of the contributions of each ingredient and, similarly, the nutritional contribution of a blend of ingredients is the sum of the nutrient contribution of each ingredient;
• Proportionality - the change in the contribution of an ingredient in a blend changes the nutritional value and cost of the blend in proportion to the change; and
• Divisibility - the incorporation of an ingredient in a mixture is divisible indefinitely.
In addition to these assumptions, linear programming requires certainty that implies that the coefficients are known and constant. However, it is important to verify these assumptions when selecting a method for estimating the availability of nutrients. For example, the apparent ileal digestibility of amino acids does not satisfy the additivity constraint, because the animal response to increasing levels of an amino acid is not necessarily linear (Stein et al., 2007). Ileal digestibility values were standardized to circumvent these limitations (Jondreville and Dourmad, 2005; Stein et al., 2007).
We saw that, in the traditional least-cost formulation method, ci represents the price per unit weight of the i
th ingredient. This value can be modified to incorporate an additional cost, namely that associated with the amounts of N or P that are estimated to be in excess or unavailable. The objective function will be then written for N and P, respectively, as follows:

where
pi is the cost of ingredient i per unit weight, r
1,i and r
2,
i are, respectively, the amounts of N and P resulting from the i
th ingredient, and
α and
β are the unit costs associated with the excess or unavailable N and P, respectively. This additional feed cost represents the environmental feed costs and should be viewed in the feed formqulation context as an approximation of N and P excretion. Thus, the values of α and β can be viewed as a tax on excretion, an excretion treatment cost or an additional transport cost. With this formulation, we optimize the formula by taking into account two or three objectives.
With the goal of evaluating this method, feeds for growing (20-65 kg live weight) and finishing (65-105 kg live weight) pigs formulated with two balanced protein levels (90 and 130 g/kg) and two available P levels (3.2 and 2.6 g/kg) were evaluated in two economic contexts (France and Quebec) over a 12-month period (June 2002-May 2003). Microbial phytase was added to the feeds at rates of 0, 250, 500 and 750 phytase units. All feed formulas contained the required available P and other nutrients. The increase in β reduced total P by reducing the excess and unavailable P fractions in feed formulas. During the period studied, excess and unavailable P decreased by 5% or more for an increase in feed cost of about 1.5% in France and 1% in Quebec. For some months, however, the economically optimal solution was close to a solution that produces substantially less excess and unavailable P at a slightly higher cost. For example, in nearly half of the months, a reduction of 10% or more was achieved in Quebec for the same 1% increase in ingredient costs (Figure 2). The reduction in excess and unavailable P with increasing β occurred independently of the addition of microbial phytase. The combined effect of microbial phytase incorporation and the proposed feed formulation method on the reduction of excess and unavailable P is almost additive. In contrast, because these criteria are generally in opposition, increasing one criterion will frequently decrease the other. The cost of the feed therefore increases when the economical solution is forced to further reduce the consumption of excess or unavailable dietary N or P. Thus, the combined use of these two techniques can promote the sustainability of the swine industry by contributing to the reduction of P excretion in swine operations with small increases in feeding cost. The proposed method can be applied to minerals other than P or to other livestock production systems in which mineral excretion can be estimated from feed composition. Similar results were obtained with N (Jean dit Bailleul et al., 2001), and this finding is in agreement with other studies (Chapoutot and Pressenda, 2005). Formulating balanced diets that minimize excess and unavailable nutrients is part of the precision feeding approach. More details about integrating α and β into the calculation of the objective function are given elsewhere (Jean dit Bailleul et al., 2001; Beaudoin et al., 2002; Pomar et al., 2007a; Dubeau et al., 2008).
Figure 2. Relationship between the variation in excess and unavailable P and feeding costs from June 2002 to May 2003 in the French economic context (According to Pomar et al., 2007a)
Progressive adjustment of the nutrient supply to match the requirements of a group of animals. In most countries, growing pigs are allowed to consume feed ad libitum from weaning until they reach market weight. The factors governing voluntary feed intake in growing pigs are numerous and complex and correspond to long-, medium- and short-term monitoring systems (Revell and Williams, 1993). However, it is generally accepted that pigs consume feed to meet their energy requirements for maintenance and growth (Emmans, 1981; Tess et al., 1983; Black et al., 1986). In this case, pigs consume a greater amount of low-concentrate feeds (Pekas, 1983) while limiting their consumption of energy-rich feeds (Roy et al., 2000). However, this ability to adjust feed intake can be subject to the limitation of intake capacity (Black et al., 1986; Kyriazakis and Emmans, 1995; Tsaras 1998; Whittemore et al., 2003), although this limitation decreases as the pigs get older and heavier (Pomar and Matte, 1995). In all cases, however, energy requirements increase faster than the requirements for most other nutrients, with the result that the optimal concentration of nutrients in diets for growing animals progressively decreases over the growing period (NRC, 1998). After the proper characterization of the nutritional potential of feed ingredients, the proper estimation of population nutrient requirements and the formulation of balanced diets, the accurate adjustment of the nutritional content of the feed to match these requirements is required and phase feeding is the most common technique.
Phase feeding involves feeding a number of successive diets, each differing in its protein, energy or amino acid balance in order to match the evolving nutritional requirements of the growing pigs (Figure 3). It should be expected that increasing the number of feeding phases will reduce feeding costs, decrease nutrient excretion and improve feed efficiency. The economic and environmental benefits of this concomitant nutrient adjustment increase with the increase in the number of feeding phases, as simulated by Letourneau Montminy et al. (2005) and as demonstrated by Beers et al. (1991, cited by van der Peet-Schwering et al., 1999), Bourdon et al. (1995), van der Peet-Schwering et al. (1996) and others. However, increasing the number of feeding phases increases the costs of feed storage and management. Pig production using two- and three-phase feeding systems are still the most popular (van der Peet-Schwering et al., 1999; Pigeon, 2001). The development of feeding systems that allow blend feeding and the automatic distribution of two premixes that, combined in variable ratios, could meet the requirements of pigs throughout their growing period (Feddes et al., 2000; Figure 4) makes this technique promising again, because it allows for significant reductions in nutrient excretion without increased feeding costs (Letourneau Montminy et al., 2005). Such premixes can be complete diets formulated to satisfy the requirements of pigs at the beginning and end of their growing period (Bourdon et al., 1995). Feeding with two premixes may also be a promising option for feed companies, because it means that only two feeds need to be prepared, with only the proportions changing between feeding phases and farms. However, because the needs for the various nutrients do not evolve in the same manner during growth, the preparation of these premixes becomes a non-linear problem that requires more complex resolution algorithms. Letourneau Montminy et al. (2005) partly solved this problem by modifying the formulation algorithm to prepare the two premixes simultaneously and by identifying the optimal proportion of these two premixes over the entire growing and finishing period. Mathematical models are also excellent tools and can virtually evaluate the impact of different phase-feeding strategies.
Figure 3. Nutrient concentration in feeds served to pigs according to a daily, three-phase or 10-phase feeding system
Figure 4. Example of level of incorporation of the initial (A) and final (B) premixes in blend feeding systems
The effect of feeding a population of pigs using a three-phase (3P) or daily multiphase (DP) system on growth performance, body composition, and N and P excretion was studied in a recent study with eight pens containing 10 pigs each (Pomar et al., 2007b). Two premixes were formulated with a modified formulation algorithm (Letourneau Montminy et al., 2005) and blended for the two feeding groups using an automatic feeding system developed especially for this project (Performixx Robotic Inc., Coaticook, QC, Canada). All pigs in the same pen received the same feed. The 3P pigs received the same blend in the same feeding phase, each of which lasted 28 d, whereas the DP pigs received a daily adjusted blend during the 84 d of the experiment. Individual feed intake was measured daily, the pigs were weighed at least every 2 wk, and backfat and muscle depth measurements were taken on Days 0, 28, 56 and 84 of the experiment. Body fat (BF), body lean (BL), bone mineral content (BMC) and bone mineral density (BMD) were measured in the overall pig body using a dual-energy X-ray absorptiometry (DXA) densitometer (DPX-L, Lunar Corp., Madison, WI, USA) at the beginning and end of the experiment. The DP pigs tended (P = 0.0756) to consume more feed (+3.7%) than the 3P pigs but only during the first feeding phase. The DP pigs consumed 7.3% less protein (P = 0.0052; Figure 5) but a similar amount of total P. For the entire growing period, the DP pigs tended (P = 0.0780) to gain more weight (+2.4%) than the 3P pigs, mainly because of faster growth (P = 0.0190) during the first feeding period. At the end of the study, the total body protein mass of the two groups of pigs was similar, but the DP pigs had 8% more body lipids (P = 0.0369) than the 3P pigs (Figure 6). Daily multiphase feeding reduced N excretion by 12% (P = 0.0047), whereas P excretion was reduced by less than 2% (P > 0.05). Because the DP pigs retained 8% less P (P = 0.036) and the total P contents of the premixes did not differ much, the reduction of P excretion by the daily multiphase feeding system was limited.
daily
Figure 5: Average weekly protein intake of pigs fed according to the three-phase or daily multiphase feeding system
Figure 6. Body protein and lipid retention in pigs fed according to a three-phase or daily multiphase feeding system
INDIVIDUAL FEEDING STRATEGIES FOR PRECISION FEEDING
One of the limitations of group feeding is that all pigs are fed with a unique feed during relatively long periods. Determining the optimal composition of this complete feed is complex, and when population responses are optimized, most of the pigs in the population will receive more nutrients than they need, and a small portion of the population will be fed above requirements (Figure 1). Nutrient requirements within a pig population vary greatly, and this variation is affected by many factors such as genetic variation (e.g. sows are inseminated with mixed semen), management (e.g. groups are formed with pigs born on different days and/or raised in different locations) and health. Feeding pigs individually according to genetics, gender and actual feed intake and growth patterns can help simplify the estimation of nutrient requirements and reduce excess nutrients.
Agriculture and Agri-Food Canada, the University of Lleida, Spain and the Universidade Federal de Santa Maria in RS, Brazil in collaboration with other national and international institutions is developing a new automatic and intelligent precision feeder (AIPF; patent pending) based on precision feeding techniques to optimize swine production systems from animal, economic and environmental perspectives. The potential impact of using precision feeding techniques on feeding cost and nitrogen (N) and phosphorus (P) excretion was evaluated (Pomar et al., 2011). For that purpose, data from a population of growing-finishing female pigs from 25 to 105 kg of body weight were used in this study to characterize feed intake, body weight gain and nutrient requirement patterns of individual pigs of a population (Pomar et al., 2007b). Group-feeding results have been described in the previous section. Each pig of this population was simulated using a slightly modified version of the growing pig module of InraPorc® (van Milgen et al., 2008). A detailed description of model modifications has been given previously (Hauschild et al., 2010). Because this model estimate animal responses by simulating the utilization of nutrients based on concepts used in net energy and ideal protein systems, comparisons between simulation alternatives cannot be attributed to the model itself. Individual pigs were characterized by their voluntary feed intake and growth potential, which is defined respectively by the pig's appetite expressed as net energy (NE) intake and its potential for protein deposition. The model is based on the transformation of dietary nutrients into body protein and lipids, which are then used to estimate body weight. Because the animals' appetite is expressed as net energy intake, standardised ileal lysine (Lys) requirements estimated by either method are expressed in relation to this element (Lys:NE). All results presented in this paper were obtained with the modified InraPorc model as described Hauschild et al. 2010 and Pomar et al. (2011).
In this simulation study the generated population of pigs were fed ad libitum either according to a typical three-phase feeding program or a daily tailored feed to each pig as could be provided using precision feeding techniques (Pomar et al., 2009). In the three-phase feeding program all pigs of the population received a common feed which composition was previously estimated to maximise population growth. In the daily tailored feeding program, each pig received every day the feed that satisfied its nutrients requirements.
The Lys:NE requirements for three-phase feeding program were estimated by the modified growth model for each of the three 28-day feeding phases for which the initial BW averaged 24.5 ± 4.1, 54.4 ± 4.9 and 81.6 ± 5.4 kg, respectively. The optimal population dietary Lys:NE concentration for each feeding period was estimated by simulating individual pig growth based on feeds containing 10 MJ NE/kg but graded levels of lysine. In this study, optimal population levels of lysine for each feeding phase were those maximising ADG. Maximal population ADG was reached at 1.09, 0.80 and 0.65 g/MJ of Lys:NE ratio in periods one to three, respectively (Hauschild et al., 2010). To estimate lysine requirements for daily tailored feeding program, the modified pig growth model was inverted to estimate the individual daily lysine requirement according to the animal's current state and its potential for protein deposition (van Milgen et al., 2008). The optimum daily Lys:NE ratio was obtained by dividing the daily lysine requirement by the estimated NE appetite for this day.
In this study, simulated and observed values were similar for both average daily feed intake (ADFI; 2.44 vs. 2.43 kg, P = 0.995 and average daily gain (973 vs. 972 kg, P = 0.987). Population protein and P requirements were established in this study to maximise population average daily gain and did not include any safety margins as normally used in commercial conditions. Because pigs were fed in the traditional 3P feeding program to maximise population growth, most pigs received more nutrients that required (Hauschild et al., 2010). Determining the optimal composition of this complete feed is complex, and when population responses are optimized, most of the pigs in the population will receive more nutrients than they need, and a small portion of the population will be fed above requirements (Figure 1). In the present study, maximal population ADG was 12% higher than the requirement of the average pig and corresponded to the requirement of pigs in the 82% percentile of the population (Hauschild et al., 2010). Feeding pigs under these requirements may contribute to reduce feeding cost but will also reduce population growth. In fact, the most performing pigs of the population are those that will be the most penalised with this strategy.
Most of the pigs fed in the 3P and all of the pigs in the PF program received every day the amount of nutrients or more required to satisfy their maintenance and growth needs. For most nutrients such as protein and P, overfed pigs will exhibit near optimal performance and therefore, average responses from populations fed according to both feeding programs obtained similar growth performances. Furthermore, simulated protein and P retention was not affected by the feeding method (Table 1). However, feeding pigs with daily tailored diets reduced N and P intake respectively by 25% and 29%, and the corresponding excretions were reduced both by more than 38%. Feeding costs was estimated to be reduced by more than 8.7 $/pig which represents a feeding cost reduction of 10%. It should be noted, however, that the most performing pigs of the herd received more protein and P when fed the daily tailored diets than when fed in the three-phase feeding program. Protein and P requirements estimated in this study did not include any safety margin and therefore, the estimated N and P reductions are probably underestimated. Phosphorus estimations have however be interpreted with caution because actual models simulating P retention seldom takes into account the effect of P intake in bone mineralisation.
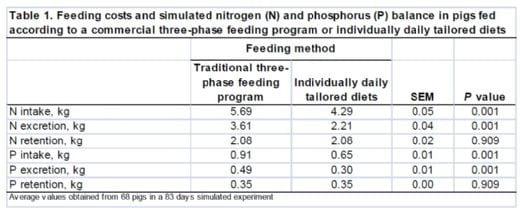
The expected reduction in feeding costs and N and P excretion which can be obtained when feeding pigs with daily tailored diets will be affected by the cost of the available ingredients, the composition of the premixes and the animal variation. Feeds formulated with large safety margins and large amounts of excess nutrients for optimal response in heterogeneous populations (e.g. genetic potential, sex and weight) increase the excretion of nutrients. In these situations, it should be expected that the impact of feeding pigs individually with daily tailored diets will reduce feeding costs and improve dietary nutrient efficiency more than observed in the present study.
This study has demonstrated that in growing-finishing pig facilities precision feeding may be an effective approach for reducing feeding costs and improving nutrient efficiency by reducing excesses of the most economically and environmentally detrimental nutrients without jeopardizing animal performance. However, the proper implementation of precision feeding in livestock production systems ought to include 1) the proper evaluation of the nutritional potential of feed ingredients, 2) the precise determination of nutrient requirements, 3) the formulation of premixes that limit the amount of excess nutrients, and 4) the concomitant adjustment of the dietary supply and concentration of nutrients to match the evaluated requirements of each pig in the herd.
More research is needed to properly calibrate the AIPF and evaluate the practical feeding and nutrient improvements and economic feasibility of this feeding approach. Nonetheless, the precision feeding approach described in this paper can be incorporated into large-group (hog-sorting) growing-finishing production systems and can thus enhance the competitiveness of the swine industry by:
1. Feeding pigs within a herd according to their individual nutrient requirements, which are modulated by their gender, genotype, growth rate, etc., and thus:
• reducing feeding costs by reducing the expensive excess supply of nutrients (protein, P, etc.),
• reducing feed fabrication, storage, management and shipping costs by using the same two or more premixes on all farms, and
• reducing the excretion of N, P and other polluting constituents of manure and the amount of soil required for manure application;
2. Managing feeds and animals using advanced computerized technologies, and thus:
• allowing real-time off-farm monitoring of feeds and animals, which is essential for optimal slaughter and production strategies that improve overall industry economic efficiency,
• reducing labour requirements and costs through the automatic monitoring and management of feeds and animals, and
• allowing the early identification of diseases and the precise application of individual treatments, resulting in improved herd performance and lower veterinary costs; and
3. Allowing easy application of treatments within the herd, and thus:
• producing value-added carcasses and meat,
• facilitating the evaluation of new feeds and feed co-products, and
• facilitating the determination of nutrient requirements.
The proposed precision farming system can help the swine industry target specific markets by:
• automatically managing individual feed supplies (voluntary or restricted feeding) and feed composition (e.g. providing higher levels of P to future reproduction gilts, limiting fatness or enhancing it in market pigs, etc.) in order to manipulate the growth rate and composition of each pig in response to specific production goals or target markets;
• allowing the early identification of diseases and the application of personalized treatments, thus helping reduce antibiotic use;
• reducing the risk of water contamination from swine operations by improving N and P use efficiency;
• reducing ammonia and methane emissions from swine facilities, contributing to the reduction of odours, and improving labour conditions and relationships with neighbours; and
• increasing nutrient use efficiency and improving animal welfare and meat quality, thus complying with societal standards in terms of environmental footprint, environmental sustainability, animal well-being and high-quality meat products, as well as improving the acceptability of pork.
Pig production in Brazil has been in continuous expansion from more than ten years with large diversity in farm size, management systems and feeding types. However, even due to that diversity, Brazilian nutritional feeding methods follow international patterns close to those observed in North America and Europe. These Brazilian production characteristics associated with new concepts in the production of grains, as the precision agriculture, can be facilitate the implantation of precision feeding techniques in Brazil.
References
Beaudoin, I., F. Dubeau, and C. Pomar. 2002. Multi-objective optimization models for swine production system (a special volume published by the east-west journal of mathematics) Computational Mathematics and Modeling. p 197-206. CMM, Bangkok, Thailand.
Birkett, S., and C. F. M. de Lange. 2001. A computational framework for a nutrient flow representation of energy utilization by growing monogastric animals. The British Journal of Nutrition 86: 661-674.
Black, J. L., R. G. Campbell, I. H. Williams, K. J. James, and G. T. Davies. 1986. Simulation of energy and amino acid utilisation in the pig. Research and Development in Agriculture 3: 121-145.
Bourdon, D., J. Y. Dourmad, and Y. Henry. 1995. Réduction des rejets azotés chez les porcs en croissance par la mise en oeuvre de l'alimentation multiphase, associée a l'abaissement du taux azoté. Journées de la Recherche Porcine 27: 269-278.
Brossard L, Dourmad J-Y, Rivest J and van Milgen J 2009. Modelling the variation in performance of a population of growing pig as affected by lysine supply and feeding strategy. Animal 3, 1110-1114.
Brossard, L., J. van Milgen, P. Y. Lannuzel, R. Bertinotti , and J. Rivest. 2006. Analyse des relations entre croissance et ingestion à partir de cinétiques individuelles : Implications dans la définition de profils animaux pour la modélisation. Journées de la Recherche Porcine 38217-224.
Chapoutot, P., and F. Pressenda. 2005. Conséquences des nouveaux «systèmes d'unités phosphore» sur la formulation des régimes. Productions Animales 18: 209-228.
Dubeau, F., P. O. Julien, and C. Pomar. 2008. Étude de cas : Un porcin dans un environnement sain. INFOR 46.
Emmans, G. C. 1981. A model of the growth and feed intake of ad libitum fed animals, particularely poultry. In: G. M. Hillyer, C. T. Whittemore and R. G. Gunn (eds.) Computers in animal production. The british society of animal production-occasional publication no 5. p 103-110. Thames Ditton, Surrey, England.
Feddes, J. J. R., C. A. Ouellette, and J. J. Leonard. 2000. A system for providing protein for pigs in intermediately sized grower/finisher barns. Canadian Agricultural Engineering 42: 209-213.
Ferguson, N. S., R. M. Gous, and G. C. Emmans. 1994. Preferred components for the construction of a new simulation model of growth, feed intake and nutrient requirements of growing pigs. South African Journal Of Animal Science 24: 10-17.
Ferguson, N. S., R. M. Gous, and G. C. Emmans. 1997. Predicting the effects of animal variation on growth and food intake in growing pigs using simulation modelling. Animal Science 64: 513-522.
Fuller, M. F. 2004. The encyclopedia of farm animal production. CABI Pub., Wallingford, Oxon, UK.
Fuller, M. F., and A. G. Chamberlain. 1982. Protein requirements of pigs. In: W. Haresign (ed.) Recent advances in animal nutrition. p 175-186. Butterwoths, London, U.K.
Hauschild, L., C. Pomar, and P. A. Lovatto. 2010. Systematic comparison of the empirical and factorial methods used to estimate the nutrient requirements of growing pigs. Animal 4: 714-723.
Honeyman, M. S. 1996. Sustainability issues of US. Swine production. Journal of Animal Science 74: 1410-1417.
Jean dit Bailleul, P., J. F. Bernier, J. van Milgen, D. Sauvant, and C. Pomar. 2000. The utilization of prediction models to optimize farm animal production systems: The case of a growing pig model. In: J. P. McNamara, J. France and D. Beever (eds.) Modelling nutrient utilization in farm animals No. Wallingford, Oxon, UK. p 379-392. CAB International 2000, Wallingford, Oxon, UK.
Jean dit Bailleul, P., J. Rivest, F. Dubeau, and C. Pomar. 2001. Reducing nitrogen excretion in pigs by modifying the traditional least-cost formulation algorithm. Livestock Production Science 72: 199-211.
Johnson, D. E. 2007. Contributions of animal nutrition research to nutritional principles: Energetics. Journal of Nutrition 137: 698-701.
Jondreville, C., and J. Y. Dourmad. 2005. Le phosphore dans la nutrition des porcs. Productions Animales 18: 183-192.
Jongbloed, A. W., and N. P. Lenis. 1992. Alteration of nutrition as a means to reduce environmental pollution by pigs. Livestock Production Science 31: 75-94.
Knap, P. W. 1999. Simulation of growth in pigs: Evaluation of a model to relate thermoregulation to body protein and lipid content and deposition. Animal Science 68: 655-679.
Knap, P. W. 2000. Stochastic simulation of growth in pigs: Relations between body composition and maintenance requirements as mediated through protein turn-over and thermoregulation. Animal Science 71: 11-30.
Kyriazakis, I., and G. C. Emmans. 1995. The voluntary feed intake of pigs given feeds based on wheat bran, dried citrus pulp and grass meal, in relation to measurements of feed bulk. British Journal of Nutrition 73: 191-207.
Lassiter JW and Edwards HM 1982. Animal nutrition. Reston Publishing Company, Reston, VA, USA.
Le Bellego, L., and J. Noblet. 2002. Performance and utilization of dietary energy and amino acids in piglets fed low protein diets. Livestock Production Science 76: 45-58.
Leclercq, B., and C. Beaumont. 2000. Etude par simulation de la réponse des troupeaux de volailles aux apports d'acides aminés et de protéines. Productions Animales 13: 47-59.
Letourneau Montminy, M.-P., C. Boucher, C. Pomar, F. Dubeau, and J.-P. Dussault. 2005. Impact de la méthode de formulation et du nombre de phases d'alimentation sur le coût d'alimentation et les rejets d'azote et de phosphore chez le porc charcutier. Journées de la Recherche Porcine 37: 25-32.
Moughan, P. J., W. C. Smith, and G. Pearson. 1987. Description and validation of a model simulating growth in the pig (20-90 kg liveweight). New Zealand Journal of Agricultural Research 30: 481-490.
Noblet, J., V. Bontems, and G. Tran. 2003. Estimation de la valeur énergétique des aliments pour le porc. Productions Animales 16: 197-210.
Noblet, J., and N. Quiniou. 1999. Principaux facteurs de variation du besoin en acides aminés du porc en croissance. Techni-Porc 22: 9-16.
Noblet, J., and J. van Milgen. 2004. Energy value of pig feeds: Effect of pig body weight and energy evaluation system. Journal of Animal Science 82: E229-238.
NRC. 1998. Nutrient requirements of swine. 10th ed. National Academy Press, Washington, DC.
Patience, J. F., P. A. Thacker, and C. F. M. de Lange. 1995. Swine nutrition guide. 2nd ed. University of Saskatchewan. Prairie Swine Center, Saskatoon, Saskatchewan Canada.
Pekas, J. C. 1983. Effect of gastric-feeding on feed consumption, growth, organ size, and body composition of swine. Appetite 4: 23-30.
Pigeon, S. 2001. Suivi des plans des interventions agroenvironnementales des fermes porcines du québec. Rapport final présenté à fédération des producteurs de porcs du québec, février 2003 (année de référence 2001).
Pomar, C. 1995. A systematic approach to interpret the relationship between protein intake and deposition and to evaluate the role of variation on production efficiency in swine. In: Proc. of the symposium on Determinants of Production Efficiency in Swine. p 361-375.
Pomar, C. 2007. Predicting responses and nutrient requirements in growing animal populations: The case of the growing-finishing pig. In: M. D. Hanigan, J. A. Novotny and C. L. Marstaller (eds.) Mathematical modeling in nutrition and agriculture. p 309-330. Virginia Polytechnic and State University, Blacksburg, VA.
Pomar, C., and J. J. Matte. 1995. Effet de l'incorporation d'écailles d'avoine dans l'aliment servi à volonté sur le rationnement en nutriments, la prise alimentaire et les performances de croissance du porc en finition. Journées de la Recherche Porcine 27: 231-236.
Pomar, C., D. L. Harris, and F. Minvielle. 1991. Computer-simulation model of swine production systems. 1. Modeling the growth of young-pigs. Journal of Animal Science 69: 1468-1488.
Pomar, C., I. Kyriazakis, G. C. Emmans, and P. W. Knap. 2003. Modeling stochasticity: Dealing with populations rather than individual pigs. Journal of Animal Science 81 (E. Suppl. 2):: E178-E186.
Pomar, C., F. Dubeau, M.-P. Letourneau Montminy, C. Boucher, and P.-O. Julien. 2007a. Reducing phosphorus concentration in pig diets by adding an environmental objective to the traditional feed formulation algorithm. Livestock Science 111: 16-27.
Pomar, C., J. Pomar, D. Babot, and F. Dubeau. 2007b. Effet d'une alimentation multiphase quotidienne sur les performances zootechniques, la composition corporelle et les rejets d'azote et de phosphore du porc charcutier. Journées de la Recherche Porcine 39: 23-30.
Pomar, C., L. Hauschild, G. H. Zhang, J. Pomar, and P. A. Lovatto. 2009. Applying precision feeding techniques in growing-finishing pig operations. Revista Brasileira de Zootecnia 38 226-237 (Supl. special).
Pomar C, Hauschild L, Zhang GH, Pomar J, Lovatto PA (2011) Precision feeding can significantly reduce feeding cost and nutrient excretion in growing animals. In Modelling nutrient digestion and utilisation in farm animals (In press).
Revell, D. K., and I. H. Williams. 1993. A review physiological control and manipulation of voluntary food intake. In: E. S. Batterham (ed.) Manipulating pig production iv. p 55-80. Australasian Pig Science Association.
Rotz, C. A. 2004. Management to reduce nitrogen losses in animal production. Journal of Animal Science 82: E119-137.
Évaluation de la capacité d'ingestion et du potentiel de dépôt protéique maximal des porcs de 20 à 65 kg de poids vif. Journées de la Recherche Porcine 32: 227-233.
Sauvant, D., J.-M. Perez, and G. Tran. 2004. Tables de composition et de valeur nutritive des matières premières destinées aux animaux d'élevage: Porcs, volailles, bovins, ovins, caprins, lapins, chevaux, poissons No. . INRA Editions, Paris, France.
Sauvant, D. 1992. Systematic modelling in nutrition. Reprod Nutr Dev 32 : 217-30.
Stein, H. H., B. Seve, M. F. Fuller, P. J. Moughan, and C. F. M. de Lange. 2007. Invited review: Amino acid bioavailability and digestibility in pig feed ingredients: Terminology and application. Journal of Animal Science 85: 172-180.
Tsaras, L. N. 1998. The prediction of the voluntary food intake of pigs on poor quality foods. Animal Science 66: 713-723.
Tess, M. W., G. L. Benett, and G. E. Dickerson. 1983. Simulation of genetic changes in life cycle efficiency of pork production. Journal of Animal Science 56: 336-353.
van der Peet-Schwering, C. M. C., A. W. Jongbloed, and A. J. A. Aarnink. 1999. Nitrogen and phosphorus consumption, utilisation and losses in pig production: The netherlands. Livestock Production Science 58: 213-224.
van der Peet-Schwering, C. M. C., N. Verdoes, and G. M. Beelen. 1996. Effect of feeding and housing on the ammonia emission of growing and finishing pig facilities. Research Institute for Pig Husbandry 5.3: 27-28.
van Milgen, J., L. Brossard, and J. Y. Dourmad. 2009. Using inraporc to reduce nitrogen and phosphorus excretion. In: P. C. Garnsworthy and J. Wiseman (eds.) Recent advances in animal nutrition - 2008. p 179-194. Nottingham University Press, Nottingham.
van Milgen, J., and J. Noblet. 2003. Partitioning of energy intake to heat, protein, and fat in growing pigs. Journal of Animal Science 81 (E. Suppl. 2): E86-E93. Online. Available: http://www.asas.org/symposia/03esupp82/jas2426.pdf. Accessed May. 2421, 2003.
van Milgen, J. Valancogne, A., Dubois, S., Dourmad, J.-Y., Seve, B. and Noblet, J. 2008. Inraporc: A model and decision support tool for the nutrition of growing pigs. Animal Feed Science and Technology 143: 387-405.
Wellock, I. J., G. C. Emmans, and I. Kyriazakis. 2004. Modeling the effects of stressors on the performance of populations of pigs. Journal of Animal Science 82: 2442-2450.
Wilton, J. W., C. A. Morris, E. A. Jenson, A. O. Leigh, and W. C. Pfeiffer. 1974. A linear programming model for beef cattle production. Canadian Journal of Animal Science 54: 693-707.
Whittemore, C. T., and R. H. Fawcett. 1976. Theoretical aspects of a flexible model to simulate protein and lipid growth in pigs. Animal Production 22: 87-96.
Whittemore, E. C., G. C. Emmans, and I. Kyriazakis. 2003. The relationship between live weight and the intake of bulky foods. Animal Science 76: 89-100.
Roy, A., J. F. Bernier, and C. Pomar. 2000.
This presentation was given at the IV CLANA CBNA AMENA, Sao Pedro, Brazil in November 2010. Engormix.com thanks the author and the organizing committee for this huge contribution to our community.