Introduction
Dairy farm profitability depends on a herd’s reproductive performance, but this relationship is complex. Farmers and consultants can easily assess reproductive performance by benchmarking pregnancy rate (i.e., 21-d pregnancy rate) or other reproductive metrics, but they find it difficult to measure the economic impact (e.g., profitability) of changes in reproductive outcomes, alternative reproductive management programs, or reproductive management decisions that affect individual cows. This paper presents a decision support tool that may assist dairy farm managers in making better decisions regarding the economics of reproductive management.
Rationale
The overall economic value of improving reproductive performance could be assessed by simulating the expected herd structure according to reproductive performance (e.g., herd dynamics in response to 21-d pregnancy rate) and calculating the expected herd net return (De Vries et al., 2010; Fricke et al., 2010; Cabrera, 2011). This is an important first step for realizing the farm-specific potential gains due to improved reproductive efficiency. Nonetheless, oftentimes, decision-makers are confronted with specific reproductive program adjustments (e.g., the possibility to decrease the interval between AI services), reproductive program changes (e.g., a different synchronization protocol), or introduction of new technologies (e.g., heat detection devices) for which they would like to know the full economic impact. The marginal net return of introducing reproductive innovations and managerial changes could be assessed by simulating reproductive performance along with its costs and benefits on a farm-by-farm specific basis (Giordano et al., 2011; 2012; 2013; Kalantari and Cabrera, 2012). An online decision support tool (http://DairyMGT.uwex.edu/ : Tools: Wisconsin-Cornell Dairy Repro: A Reproductive Programs Economics Analysis Tool) has been created for this purpose and is the focus of this paper.
Different herds can achieve similar reproductive performance with different reproductive management programs, whereas different reproductive performance could be observed with the same reproductive management program in different herds. For example, two herds using the same synchronization of ovulation protocols in combination with AI after detection of estrus may observe very different 21-d pregnancy rates due to different levels of fertility (a trait affected by many factors) and efficiency to detect cows in estrus. Moreover, fine-tuning reproductive programs (e.g., replacing Cosynch-72 by Ovsynch-56 to increase fertility to TAI services) and adopting new technologies would generate differences in reproductive performance and expected net returns for the farm. The ability to compare the future performance of a farm’s current versus an alternative reproductive program, and therefore the impact on profitability, is paramount for reproductive management decision-making. Examples of these changes could encompass:
- Including, excluding, or changing the level of use of either or both synchronization programs and estrus detection programs (Giordano et al., 2011);
- Changing the interbreeding interval (IBI) of timed AI (TAI) services after synchronization of ovulation (Giordano et al., 2011);
- Exploring different plausible service and conception rates (Giordano et al., 2012);
- Starting a resynchronization protocol before the first pregnancy test;
- Introducing the use of chemical pregnancy tests to detect nonpregnant cows (Giordano et al., 2013); or
- Assessing the cost benefit of introducing heat detection devices.
These crucial analyses need to be performed within a defined farm, in which the difference between two scenarios, current and alternative, could provide a good measure of the impact of the proposed changes.
The Decision Support Tool
The Wisconsin-Cornell Dairy Repro tool provides the opportunity to define reproductive programs in great detail. For instance, the user can describe any estrus detection (ED), any synchronization program for TAI, or, what is more common, any combination of both for the current reproductive program and for an alternative one.
The first step for defining reproductive programs is to select the type of program that best describes the farm’s current management for first, second, and subsequent services, and an alternative program of interest (Figure 1). In an attempt to facilitate the simulation of alternative programs, a table has been included with the tool documentation that contains plausible results for AI services after detection of estrus or synchronization of ovulation programs (Table 1). After defining the IBI for TAI services for the current and alternative synchronization programs, the performance of the current and alternative programs is defined by outlining the percentage of cows bred in estrus before first TAI and in between TAI services as well as the expected conception rates (CR) for each breeding type (estrus detection versus TAI). Other reproductive variables such as the last day to inseminate cows before culling them from the herd, the estimated estrous cycle duration, average calving interval to estimate a priori the labor cost for estrus detection for both the current and alternative programs, and the duration of the dry period are also defined. Finally, a series of reproductive management program cost-related input variables are defined, such as the type and required labor for pregnancy diagnosis, labor for heat detection, and labor and costs associated with hormones, if any. An example of a particular type of analysis that can be accomplished by using the Wisconsin-Cornell Dairy Repro tool is presented in Figure 1. In this example, a net profit of $13,200/yr was estimated for a 1,000-cow herd when the percentage of cows bred in estrus was increased from 40% to 70% by using heat detection devices on 50% of the lactating cows.
Table 1. Reproductive programs that can be selected for first, second, and subsequent services available in the Wisconsin-Cornell Dairy Repro tool (http://DairyMGT.uwex.edu : Tools: Reproduction) and plausible results that could be used as reference. Note: The user will ultimately define the reproductive program and quantify the outcomes obtained by the current program or speculate the outcomes to be obtained by an alternative program. The values provided are estimates based on the author’s experience or data from the literature.
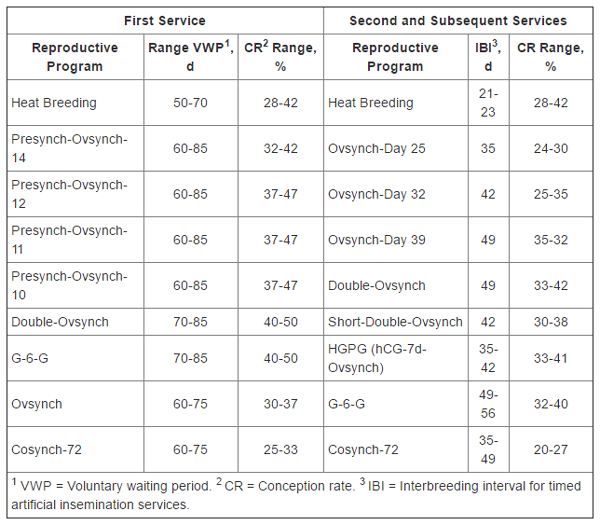
Figure 1. Screenshot of Wisconsin-Cornell Dairy Repro tool available athttp://DairyMGT.uwex.edu : Tools: Reproduction applied to test an alternative reproductive program on a 1,000-cow herd using an alternative heat detection system with a cost of $10,000 for computer/hardware, $100 per collar for 500 cows, and $1,000/yr for maintenance. It is assumed that the percentage of cows receiving AI at a detected estrus before or after TAI will increase from 40% to 70% with a CR of 35% for estrus breedings.
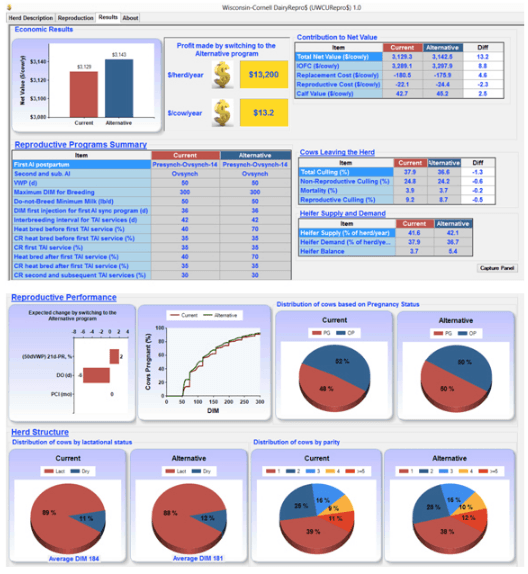
Based on the previously described user-defined reproductive data and additional herd information including number of lactating cows, culling, mortality, stillbirth, lactation curves, and economic parameters (e.g., milk price, cost of a replacement), the tool calculates, simultaneously, the cumulative pregnancies and the expected net return of cows becoming pregnant at different times throughout lactation (Figure 2). The estimated net return normally decreases with a longer nonpregnant period. For example, a cow that becomes pregnant at 60 d after a previous calving would have an average net return of $6.54/d during her current lactation. In contrast, the net return would be only $5.85/d if the cow became pregnant at 240 d, a difference of $0.69/d or $252/cow/yr. Following such concept, the economic value of a reproductive program can be assessed by multiplying the percentage of cows expected to become pregnant during a period of time (circles) times the estimated net return expected from those cows (squares).
Decision Support Tool Applications
Practical applications for the tool are outlined below. For example, a farmer could apply the tool to investigate whether the addition of estrus detection to a farm’s current pure TAI program would improve reproductive performance and profitability (following Giordano et al., 2011). In this example, the user describes the current program for first service as Double-Ovsynch (82 d voluntary waiting period; 45% CR) followed by Ovsynch for resynchronization (IBI for TAI of 42 d; 30% CR). After adding estrus detection, it is expected that 50% of the cows receive AI at detected estrus with a 30% CR across all AI services. Assessment with the tool indicates that both programs (current and alternative) would have similar reproductive performance (Figure 3, upper panel) and similar net return ($/cow/yr).
Nonetheless, further analyses of different scenarios demonstrate that the impact of adding ED could be substantially different, depending on whether it is performed before or after first TAI. Before first TAI only, ED (assuming 30% CR to ED breedings) decreases the overall value of the reproductive program because the CR to AI at detected estrus (30%) before first TAI is much lower than the CR for first TAI (45%; lower percentage of total pregnant after the first TAI for the program adding ED, Figure 3, upper panel). Further, assuming that 50% of cows receive AI at detected estrus, at least 42% CR would be needed to make this change worthwhile before first TAI (Figure 3, bottom panel). On the other hand, introducing detection of estrus (50% of the cows) after first TAI only with a CR of 30% will provide a profit of $12.25/cow/yr (Figure 3, bottom panel) to the overall farm reproductive program. Indeed, the breakeven CR of introducing estrus detection after first TAI is 27%, and there would be approximately $3.65/cow/yr additional profit for every 1% increase in the percentage of cows receiving AI at detected estrus. It could be concluded then, for this farm, that a good economic alternative would be to introduce detection of estrus, but only after first TAI.
Figure 2. Cumulative percentage of pregnant cows according to reproductive program (circles) and expected net return (squares) calculated as the aggregation of milk income over feed cost plus income from calves minus feed costs, minus culling and mortality costs, and minus reproductive program costs.
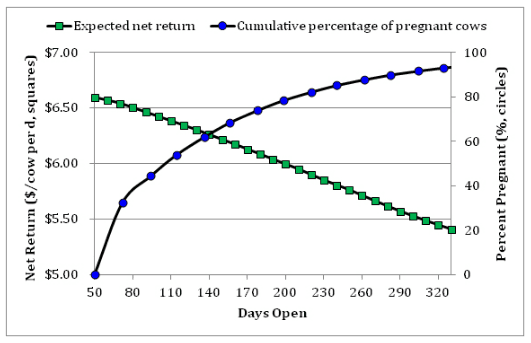
Figure 3.The top panel shows the reproductive performance of a pure timed synchronization program consisting of Double-Ovsynch (82 d voluntary waiting period; 45% conception rate) for first service followed by Ovsynch initiated 32 d after TAI for resynchronization (interbreeding interval of 42 d) and a 30% conception rate (circles) and the reproductive performance of a combined program that consists of the same synchronization program with the addition of 50% of cows receiving AI at detected estrus with a 30% conception rate before and after first timed artificial insemination (squares). The estimated net present value ($/cow per yr) for both programs was the same (no gain or loss after introducing estrus detection). The bottom panel shows the difference in net present value between an alternative program in which 50% of cows receive AI at detected estrus with different levels of conception rate. Cows receive AI at detected estrus before (squares) or after (circles) first TAI.
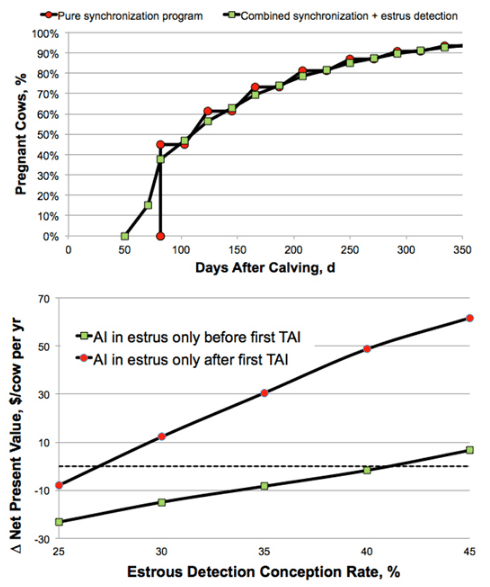
Another question that could be addressed with the tool is, for example, the value of reducing the IBI when using technologies that allow earlier identification of nonpregnant cows such as chemical tests (blood test) or ultrasound (Giordano et al., 2013) coupled with efficient resynchronization of ovulation programs. It is reasonable to expect a higher profit with a shorter IBI. Using the tool, we observed consistent net present value ($/cow/yr) increases with a range between $22 ($2,836 for 49 d IBI versus $2,814 for 56 d IBI) when 80% of cows were inseminated after estrus and $59 ($2,957 for 28 d IBI versus $2,898 for 35 d IBI) when 30% of cows were inseminated after estrus (Figure 4). These observations demonstrate the significant benefit that could be attained by shortening the IBI. However, it is important to study these profitability changes considering the potential drawbacks of using earlier nonpregnancy diagnosis. Earlier nonpregnancy diagnosis may lead to more test inaccuracies and higher levels of observed embryonic death after testing, which could have negative economic impacts. In this regard, sensitivity analysis to evaluate the use of a blood chemical test to reduce the IBI by 1 week demonstrated that such strategy was still profitable provided that the sensitivity and specificity for the chemical test was higher than 96.4% and 95.1%, respectively, and pregnancy losses during the week period from the early to the late nonpregnancy diagnosis test were less than 8.9% (Giordano et al., 2013). Because such levels of sensitivity and specificity (Silva et al., 2007; Romano and Larson, 2010), and early pregnancy losses (Santos et al., 2004) are observed in commercial farms, it could be concluded that blood chemical tests (or any other test that allows reducing the IBI) may be an economically feasible alternative under certain farm conditions.
Figure 4. Effect of interbreeding interval and estrus detection service on the net present value (NPV; $/cow/yr) following Giordano et al. (2013).
Conclusion
Evaluating the economic impact of adopting new strategies or technologies for dairy cattle reproductive management is possible with the "Wisconsin-Cornell Dairy Repro" decision support tool (http://DairyMGT.uwex.edu/ : Tools: Reproduction). Dairy farm owners, managers, and consultants have the opportunity to define current reproductive management programs and compare them to alternatives that may lead to improved reproductive performance and herd profitability.
This article was originally published in DAIReXNET eXtension. 23 October 2013.
Literature Cited
Cabrera, V.E. 2011. The economic value of changes in 21-day pregnancy rate and what controls this value. 21st American Dairy Science Association Discover Conference: Improving Reproductive Efficiency of Lactating Dairy Cows. Itasca, IL. 10 May 2011. Available at:http://dairymgt.uwex.edu/publications/abstracts/2011_Discover_Cabrera.pdf.
De Vries, A., J. Van Leeuwen, and W.W. Thatcher, 2010. Economics of improved reproductive performance in dairy cattle. University of Florida IFAS Extension. Available at:http://edis.ifas.ufl.edu/an156.
Fricke, P.M., S. Stewart, P. Rapnicki, S. Eicker, and M. Overton. 2010. Pregnant vs. open: Getting cows pregnant and the money it makes. eXtension, DAIReXNET Reproduction Resources. Available at: http://www.extension.org/pages/15604/dairy-cattle-reproduction.
Giordano, J.O., P.M. Fricke, M.C. Wiltbank, and V.E. Cabrera. 2011. An economic decision-making support system for selection of reproductive management programs on dairy farms. Journal of Dairy Science 94:6216-6232.
Giordano, J.O., A. Kalantari, P.M. Fricke, M.C. Wiltbank, and V.E. Cabrera. 2012. A daily herd Markov-chain model to study the reproductive and economic impact of reproductive programs combining timed artificial insemination and estrus detection. Journal of Dairy Science 95:5442-5460.
Giordano, J.O., P.M. Fricke, and V.E. Cabrera. 2013. Economics of resynchronization strategies including chemical tests to identify nonpregnant cows. Journal of Dairy Science 96:949-961.
Kalantari, A.S., and V.E. Cabrera. 2012. The effect of reproductive performance on the dairy cattle herd value assessed by integrating a daily dynamic programming with a daily Markov chain model. Journal of Dairy Science 95:6160-6170.
Romano, J.E., and J.E. Larson. 2010. Accuracy of pregnancy specific protein-B test for early pregnancy diagnosis in dairy cattle. Theriogenology 74:932-939.
Santos, J.E., W.W. Thatcher, R.C. Chebel, R.L. Cerri, and K.N. Galvao. 2004. The effect of embryonic death rates in cattle on the efficacy of estrus synchronization programs. Anim. Reprod. Sci. 82-83:513-535.
Silva, E., R.A. Sterry, D. Kolb, N. Mathialagan, M.F. McGrath, J.M. Ballam, and P.M. Fricke. 2007. Accuracy of a pregnancy-associated glycoprotein ELISA to determine pregnancy status of lactating dairy cows twenty-seven days after timed artificial insemination. J. Dairy Sci. 90:4612-622.