INTRODUCTION
The profitability of dairy farms is an increasing concern in many economies around the world (Ellis et al., 2020; Ojo et al., 2020). This is the result of continuous economic, political, social, and environmental factors affecting different countries and regions (O’Leary et al., 2018; Ramsbottom et al., 2021). Therefore, dairy farmers are continuously searching and updating strategies and technologies that can improve the dairy farm technical and economic performance (Atzori et al., 2013). Dairy cows under heat stress (HS) have reduced DMI, milk yield, and reproductive performance and increased culling, diseases, and mortality rates, resulting in a negative effect on the economy of the farm (Collier et al., 2017; Armengol and Fraile, 2018; Becker et al., 2020). Recent reports indicate that the majority of dairy cows across the world experience some degree of HS, directly affecting the dairy farm profitability (Hempel et al., 2019; Ouellet et al., 2019; Ogundeji et al., 2021). Furthermore, the continuous increase in milk yield due to genetic and management improvements (Weigel et al., 2017) and the increasing global temperatures due to climate change (Segnalini et al., 2013; Hristov et al., 2018; Gauly and Ammer, 2020) will lead to more HS, mainly in European dairy breeds with a reduced ability to dissipate excess heat load gain during summers in the Mediterranean (Pereira et al., 2014). Cooling systems (CS) can partially revert these effects (Chen et al., 2016; Polsky and von Keyserlingk, 2017). To accurately evaluate the effects of HS and CS, it is necessary to develop models that estimate the complex biological response quantitatively, over time. The classical deterministic approaches to calculate profitability can be practical to use but ignore the complexity and dynamic interactions of a dairy herd (Dhuyvetter et al., 2000; VanBaale et al., 2004). For example, cows under HS experience reduced fertility in summer months, resulting in changes in the seasonal calving, changes in production, incidence of diseases, and effects on culling strategies. Several stochastic and dynamic dairy herd simulators have been developed and can be used to evaluate the effects of management changes in dairy herd technical and economical indices (Sørensen et al., 1992; De Vries, 2004; Calsamiglia et al., 2018). However, to our knowledge they have not been used to evaluate the effects of HS and CS. The aim of the present study was to determine the technical and economic impact of HS in selected locations of the Mediterranean with a wide range of intensities of HS to model the profitability of investing in CS using a stochastic dynamic dairy herd simulator.
MATERIALS AND METHODS
Weather Data and Locations Selection
The present study was conducted without using live animals, so approval by an Institutional Animal Care and Use Committee was not necessary. Historical weather data from 276 locations worldwide from 2016 to 2018 were collected. All data were obtained from the online Weather Underground platform (TWC Product and Technology IBM-LLC; https://www.weatherunderground.com). Of this database, 70 locations were selected from across Europe, North Africa, and the Middle East within the latitudes (64°14′38.81″N to 31°39′16.91″N) and longitudes (−25°23′35.67″E to 37°22′39.40″E), to map the HS intensity in the Mediterranean and surrounding areas. Of the 70 locations, only those under the class Csa subtype that is characterized by hot and dry summers by the Köppen-Geiger climate classification were considered for the present analysis, which accounts for 21 locations (Beck et al., 2018). The data include the daily minimum and maximum temperature (Tmin–max) and relative humidity (RHmin–max).
Heat Stress and Cooling Systems Model
To estimate the biological response to HS and the reduction effects of CS in the different locations, daily weather data were submitted to the sine temperature and humidity index (THI) model proposed by St-Pierre et al. (2003), which runs day by day over a 24-h period, calculating the THI load (THILoad) that represents the integral of the cumulative THI units above a threshold (THIthreshold) where HS occurs. The THIthreshold value of the sine model was set at 68 units (Zimbelman et al., 2009). The THI index equation used in the model was proposed by the NRC (1971), where T is the air temperature (°C) and RH is the relative humidity (%):
THI = (1.8 × T + 32)
– [(0.55 – 0.0055 × RH) × (1.8 × T – 26)].
From the outputs of the sine model, several equations were used as proposed by St-Pierre et al. (2003) to estimate the reduction in DMI (kg/cow per day), milk yield (kg/cow per day), and pregnancy rate (PR, %/mo) and increases in mortality (%/mo) as a function of the THILoad. In the present research, the implementation of CS considers the use of fans and sprinklers in the feeding line as described by St-Pierre et al. (2003), with the assumption that one unit of CS is needed per 3,800 kg of BW, and each unit consumes 0.65 kW/h. The model assumes that the reduction of the THI dipends on current temperature and relative humidity. Therefore, the reduction in THI under CS conditions was described with the followingequation, which represents the reduction in the annual THILoad (St-Pierre et al., 2003):
ΔTHI = –17.6 + (0.36 × T) + (0.04 × RH),
where ΔTHI is the change in the apparent THI, T is the current temperature (°C), and RH is the current relative humidity (%) before applying the CS. All the calculations were made with the MS Excel 365 spreadsheets (Microsoft Corporation). A contour plot map representing the total THILoad (units/yr) with the data of the 70 locations was created with OriginLab Pro 7.5 (OriginLab Corp.). The fluctuations in milk fat and protein content within a year were incorporated into the simulation, and details of the model are shown in Supplemental Table S1 and Figures S1 and S2 (https:/ /doi.org/10.6084/m9.figshare.21678932; Espinoza Sandoval, 2022).
The Dairy Herd Simulator Scenarios Model
General Scenario Creation. The effects of HS and CS were simulated over 6 years with 60 replicates per year in a stochastic dynamic dairy herd simulator (Calsamiglia et al.,2018). The initial configuration for all the scenarios was a herd with the same technical and economic characteristics: 300 dairy cows with average milk yield of 40 L/cow per day (12,200 L/cow per 305 d), a PR of 20%, and a milk price of €0.28, €0.32, and €0.36/L (Table 1). The milk prices were selected based on Spain’s national average and variations between milk buyers. The effects of HS and CS on DMI, milk yield, reproduction, and culling were adopted from St-Pierre et al. (2003). The milk fat and protein content were submitted to the model developed to adjust fluctuations to typical conditions of the Mediterranean, where each month of the year was calibrated to fit the model presented in Supplemental Material (https://doi.org/10.6084/m9.figshare.21678932; Espinoza Sandoval, 2022).
Table 1. General inputs (productive, reproductive, and economic) configurated in the dairy herd simulator for each of the scenarios of heat stress and cooling systems
A control scenario (THILoad = 0) was also created as a reference without any effects of HS or CS but with the same technical and economic characteristics of the other scenarios.
Heat Stress and Cooling System Scenarios. In the herd previously described, 7 intensities of HS scenarios were simulated individually in intervals of 5,000 THILoad: 1,000, 6,000, 11,000, 16,000, 21,000, 26,000, and 31,000. The effects of HS were evaluated for 330, 1,080, 1,560, 2,100, 2,700, 3,360, and 4,080 h/ yr of HS, respectively. The effects of HS calculated with the model described previously included DMI loss, milk yield loss, PR reduction, and culling rate increases (St-Pierre et al., 2003). Although changes in PR are the combined effects on fertility and heat detection rate, in the present model, these changes apply only as changes in fertility. In the 7 previously described scenarios, the effects of CS were estimated at an initial investment of €100 and €200/cow, with a payoff time of 6 years and an interest rate of 5%. The operation cost considered electricity, water, and maintenance and was set at €0.097/h per unit of CS, representing €5.74/h of CS for 280 cows.
Heat Stress and Cooling Systems Profitability Model
Productive and Technical Model. A total of 72 HS and CS scenarios were simulated. Of the 90 outputs generated by the simulator, 6 variables were selected for their relevance, including milk yield, feed costs, PR, culling rate, income over feed costs (IOFC), and number of lactations. Therefore, 12 equations were obtained, 6 for HS and 6 for CS, as a function of the THILoad for each of the scenarios previously described. Depending on the type of response, linear and segmented regressions were performed. Then, the 12 equations were applied to each of the 21 selected Mediterranean locations but could be used to estimate the effects on other locations within the range of THILoad
Model of Change for the Net Margin. The change in net margin (€/cow per year; ΔNM), which represents the total economic response of the simulations, was calculated as the differential between the HS and CS scenarios and then modeled as 2-sections segmented regression (Ryan and Porth, 2007) by the general model as follows:
y = a1 +b1 r for r < c,
y = a2 + b2 r for r > c.
where y is the change in ΔNM (€/cow per year); for the first regression line, a1 is the intercept and b1 the slope, c is the breakpoint, and x is the THILoad; and for the second regression line, when x is greater than c, a2 is the intercept and b2 the slope. This results in 6 different models that account for the 2 costs of CS and the 3 milk prices as a function of the THILoad that were applied to the 21 selected Mediterranean locations.
Statistical Analysis
The selected outputs of HS and CS simulated scenarios, such as milk yield loss, feed costs, IOFC, PR, breeding costs, and culling were submitted to linear regression analysis by the PROC REG in SAS v9.4 (SAS Institute Inc.). The outputs for the average lactations and the ΔNM between the HS and CS scenarios were submitted to 2-section segmented regression analysis using the package “Segmented” v1.6 (Muggeo, 2008) as a function of the THILoad in R v4.2 statistical software (https://www.R-project.org/).
RESULTS
Weather Data and Mapping
The analysis of 276 worldwide weather station locations is presented in Supplemental Table S2 (https://doi.org/10.6084/m9.figshare.21668009; Espinoza Sandoval, 2022), containing the location name and its closest meteorological station, the duration of HS in hours per year, and the yearly THILoad. Figure 1 shows the contour plot map of 70 locations in Europe, North Africa, and the Middle East, corresponding to the Mediterranean area and surroundings. The map shows that over the latitude of 50°, the THILoad was always less than 4,725 units, and over 52° was equal to 0. The map also shows a diagonal and linear increase of the THILoad intensity from north to south and west to east.
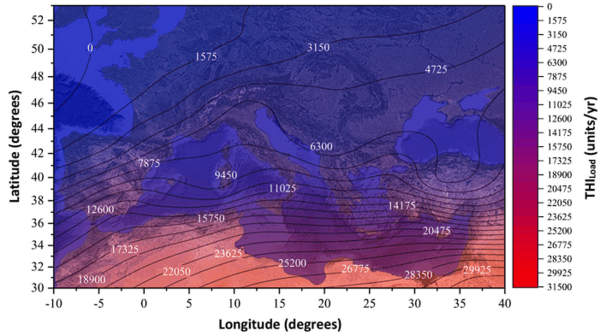
Figure 1. Contour plot map representing the yearly THILoad from 70 locations across Europe, North Africa, and the Middle East. Each contour line represents an increased interval of 1,575 THILoad (units/yr), with data ranging from 0 to 31,500. THILoad is the integral of accumulated THI units above the THIthreshold of 68.
The highest values were found in the Middle East, with a maximum of 31,424 in Tel Aviv. From the selected 21 locations under the Csa classification, the THILoad (average of 12,530 ± 5,730) ranged from 6,908 to 31,4.
Productive, Technical, and Economic Simulation Outputs
The general, productive, and reproductive outputs of the 14 simulated scenarios are presented in Table 2.
The economic consequences of HS and the implementation of CS are presented in Tables 3 and 4, respectively. Several model equations of these consequences for technical and economic outputs were developed as a function of the THILoad (Supplemental Tables S3, S4, and S5; https://doi.org/10.6084/m9.figshare.21668009; Espinoza Sandoval, 2022). Their graphical representations are shown in Supplemental Figure S3 (https://doi.org/10.6084/m9.figshare.21668009; Espinoza Sandoval, 2022). Changes in milk yield were the most relevant effect of HS and CS. The fluctuations of milk yield under HS and CS scenarios at different THILoad are shown in Figure 2. In the HS scenarios, the average daily milk yield was reduced 17% (from 41.7 to 35.7 L/d), but daily losses during the months most prone to HS reached up to 15 L/d in the 31,000 THILoad scenario. The overall result was a farm yearly total milk yield loss due to HS from 3 to 434 (×1,000 L). After the implementation of CS, milk losses were reduced by 60% compared with the HS scenario losses, and yearly averages increased. For example, in the 11,000 THILoad scenario, the yearly average milk yield increased from 40.0 to 41.0 L/d, and for the 31,000 THILoad scenario, it increased from 35.7 to 39.3, compared with the 41.7 L/d in the control scenario. Moreover, the total herd milk loss was lower, ranging from 2 to 215 (×1,000 L). Although the incorporation of milk fat and protein content fluctuations throughout the year is relevant in the model, these fluctuations are apparently due to natural circadian cycles and independent of HS (Salfer et al., 2019). Furthermore, no model describes the effects of HS and CS on milk fat and protein content and, therefore, we could not incorporate that into the present simulation. The result is that milk composition was not affected by THILoad, nor by the implementation of CS (Table 2). The HS reduced DMI, resulting in a reduction of €56,597 in feed costs in the 31,000 THILoad scenario. In the CS scenarios, the feed costs were lower than in control but higher compared with the corresponding HS scenario, representing an increase of up to €30,353 in the 31,000 THILoad scenario due to the CS. The IOFC (€/cow per day) was reduced by 19%, from 5.24 in the non-HS scenario to 4.23 under HS, and recovered around 40% of the losses with CS compared with the losses from HS. The effects on reproductive efficiency were measured as PR. The fluctuations of PR under HS and CS scenarios at different THILoad are shown in Figure 3.
Table 2. Selected outputs of general, productive, and reproductive performance of the theoretical simulated scenarios of heat stress (HS) and cooling systems (CS)
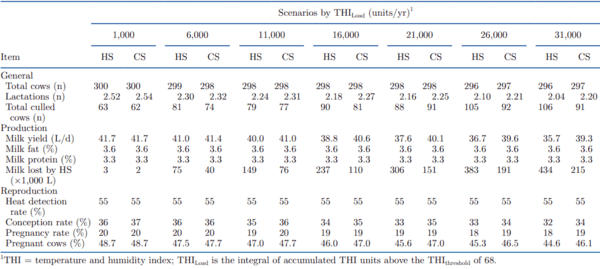
During months of HS, PR was reduced from 20 to 15%. However, the effects of HS lasted for some months, and the average yearly PR in the HS scenarios ranged from 18 to 20%. When CS were implemented, PR recovered back to 19 to 20%. This reproductive response increased the average number of pregnant cows in the CS scenarios. For example, in the 31,000 THILoad scenario, the percentage of pregnant cows in the herd was reduced from 48.7 in non-HS to 44.6 in HS and then partially recovered to 46.1 after the implementation of the CS. The cost of breeding increased as the THILoad increased due to lower fertility, ranging from €11,275 to €11,773. The implementation of CS reduced breeding costs from €11,508 to €11,262 but, overall, differences were quantitatively small. The total number of culled cows increased in the HS scenarios from 63 to 106 cows, and the CS reduced the culling from 91 to 62 cows depending on THILoad. The number of average lactations of cows in the HS simulated scenarios decreased from 2.52 to 2.04 and was partially recovered after the implementation of CS, ranging from 2.20 to 2.54. This was attributed to the reduction of culled cows and the increase in PR. The effects of HS and CS in the function of the THILoad on milk yield, PR, and net margin had a strong linear relationship, and equations are presented in Supplemental Tables S3, S4, and S5 and Figure S3 (https://doi.org/10.6084/m9.figshare.21668009; Espinoza Sandoval, 2022).
Change in the Net Margin
The segmented regression models for the ΔNM are presented in Table 5, and the yearly evolution of the net margin in the different THILoad and CS scenarios is shown in Figure 4. The ΔNM (€/cow per year) is the differential between the total expenses and income, so this variable accounts for all the dairy herd interaction effects. In Figure 5, where the models are presented with the 3 milk prices and 2 CS initial investment scenarios, a plateau between scenarios 1,000 and 6,000 THILoad was observed. When the THILoad increased above 6,000, a linear increase was observed in all the economic scenarios (R2 = 0.99; P < 0.01). The estimated THILoad values when the line crossed 0 in ΔNM (€/cow per year) for the initial CS investment of €100/cow and the 3 milk prices were 8,380 at €0.28/L, 7,269 at €0.32/L, and 6,352 at €0.36/L. When the initial CS investment was €200/cow, the values were 11,005 at €0.28/L, 9,141 at €0.32/L, and 8,547 at €0.36/L. In all cases, as milk prices increased or the investment cost of CS decreased, the THILoad at which the implementation of CS was profitable was lower.
Table 3. Economic outputs of the simulations, with the absolute value of the control and the difference between the control and the heat stress (HS) scenarios, at a milk price of €0.32/L
1 Absolute value per farm and year in the control (CON) scenario. THI = temperature and humidity index. HS scenarios (THILoad, units/yr) difference compared with control. THILoad is the integral of accumulated THI units above the THIthreshold of 68.
2 IOFC = income over feed costs.
Productive, Technical, and Economic Estimations in the Mediterranean
The models previously described were applied to the 21 locations across the Mediterranean to evaluate the effects of HS compared with the control scenario (Table 6). Milk yield decreased on average 655 ± 316 L/cow per year, ranging from 346 to 1,696 L. Feeding costs decreased on average €109 ± €48/cow per year, ranging from €62 to €267, and the IOFC also decreased to an average of €4.5 ± €0.2/cow per day, ranging from €3.8 to €4.7. Annual PR decreased on average 1.0 ± 0.5%/ yr, ranging from 0.6 to 2.5%. Culling rates increased on average 8.3 ± 2.5%/yr, ranging from 5.8 to 16.6%, and the number of lactations decreased on average 0.32 ± 0.06, ranging from 0.26 to 0.50. After implementing CS in the 21 locations across the Mediterranean (Table 7), compared with the HS scenarios, milk yield increased on average 330 ± 160 L/cow per day, ranging from 173 to 859 L. Although feeding costs increased on average €52 ± €26/cow per year, ranging from €29 to €139, the IOFC increased on average €0.3 ± €0.1/cow per day, ranging from €0.1 to €0.7. Annual PR increased on average 0.3 ± 0.2%/yr, ranging from 0.1 to 1.0%. Culling rates decreased on average 1.7 ± 0.7%/yr, ranging from 0.1 to 3.9%, and the number of lactations increased in average 0.06 ± 0.03, ranging from 0.04 to 0.15. The 6 ΔNM model equations were applied to the 21 selected locations in the Mediterranean as a function of the THILoad. The segmented regression had 2 linear sections, but the THILoad values were above the breakpoints of the models for all the locations (Table 8). For the initial investment in CS of €100/cow, the ΔNM (€/ cow per year) with a milk price of €0.28/L averaged €28 ± €34, ranging from −€9 to €135, being negative in 4 locations and positive for the rest. For a milk price of €0.32/L, the average was €41 ± €44, ranging from −€2 to €187, being negative in 2 locations and positive for the others. For a milk price of €0.36/L, the average was €59 ± €55, ranging from €5 to €239, being positive in all locations. When the initial investment in CS increased to €200/cow, the number of locations with a negative ΔNM (€/cow per year) increased. For a milk price of €0.28/L the average was €9 ± €34, ranging from −€24 to €120, being negative in 9 locations and positive in 14. With a milk price of €0.32/L, the average was €26 ± €45, ranging from −€17 to €174, being negative in 8 locations a positive in 15. For a milk price of €0.36/L, the average was €39 ± €56, ranging from −€16 to €225, being negative in 5 locations and positive in 16. Lisbon and Marseille, with a THILoad of 6,908 and 7,007, respectively, were only profitable in the scenario of €0.36 of milk price and €100 of CS investment. Beja and Girona were positive only in the scenarios of €0.32 and €0.36 of milk price and an investment in CS of €100. In Barcelona, Alghero, and Rome, with a THILoad ranging from 8,472 to 8,591, the ΔNM was positive in the €100 and negative in all the scenarios of CS at €200. Larissa was positive in all the CS scenarios of €100 and negative only in the scenarios of milk price of €0.28 and €0.32 and CS investment of €200. The ΔNM in Bari was only negative in the scenario of a milk price of €0.28 and a CS investment of €200. In contrast, in Valencia, Istanbul, Seville, Córdoba, Athens, Algiers, Trapani, Murcia, Tunis, Antalya, and Tel Aviv, where the THILoad ranged from 11,072 to 31,424, the ΔNM was positive for all scenarios, ranging from €3 to €239/ cow per year.
Table 4. Economic outputs of the simulations, with the absolute value of the control and the difference between the control and the cooling system (CS) scenarios and with an initial investment in CS of €100/cow and a milk price of €0.32/L
1 Absolute value per farm and year in the control (CON) scenario. THI = temperature and humidity index. CS scenarios (THILoad, units/yr) diference compared with control. THILoad is the integral accumulated THI units above THIthreshold of 68.
2 IOFC = income over feed cost.
Figure 2. Milk yield (L/cow per day) pattern evolution over the 6-yr simulation in the different theoretical scenarios, control (CON), heat stress (HS), and cooling systems (CS). THILoad is the integral of accumulated THI units above the THIthreshold of 68.
Figure 3. Pregnancy rate (%) pattern evolution in the 6-yr simulation in the different scenarios, control (CON), heat stress (HS), and cooling systems (CS). THILoad is the integral of accumulated THI units above the THIthreshold of 68.
Table 5. Summary of the segmented regressions model of change in the net margin (€/cow per year), which represents the difference between the heat stress and cooling system (CS) scenarios through 6 yr of simulation, with the different combinations of CS investment (€100 and €200/ cow) and milk prices (€0.28, €0.32, and €0.36/L)1
1 Intercepts(a1,2), slopes(b1,2), and breakpoints(c) of the 2-section segmented regression model. THI = temperature and humidity index; THILoad is the integral of accumulated THI units above the THIthreshold of 68. RMSE = root mean square error.
Figure 4. Net margin (€) pattern evolution in the 6-yr simulation in the scenario of €0.32 and €100, control (CON), heat stress (HS), and cooling systems (CS). THILoad is the integral of accumulated THI units above the THIthreshold of 68.
Figure 5. Segmented regression models of change in the net margin, in the theoretical scenarios (0.28–100, 0.28–200, 0.32–100, 0.32–200, 0.36–100, and 0.36–200) under different milk prices (€0.28, €0.32, and €0.36/L) and the initial investment in cooling systems (€100 and €200/ cow) as a function of the annual THILoad (units/yr). THILoad is the integral of accumulated THI units above the THIthreshold of 68.
DISCUSSION
The biological response to HS has been recognized since the mid-1930s (Rhoad, 1936). Since then, our understanding of the effects of HS on dairy cow performance has made notable progress (Tao et al., 2020). Reports from all over the world indicate that the great majority of dairy cows across the globe experience some degree of HS, directly affecting dairy farm profitability (Hempel et al., 2019; Ouellet et al., 2019; Ogundeji et al., 2021). Results of 276 locations worldwide (Supplemental Table S2; https://doi.org/10.6084/m9.figshare .21668009; Espinoza Sandoval, 2022) show only 4 places with a THILoad value of zero: La Paz, Bolivia; Punta Arenas, Chile; Nuuk, Greenland; and Reykjavík, Iceland. In contrast, the highest THILoad values were found in Balikpapan, Indonesia, and Nabire, Indonesia (91,168); Bangkok, Thailand (91,845); Nabire, India (92,289); and Singapore, Singapore (98,327). The rest of the locations presented a positive THILoad, averaging 24,150, with the lowest values being in Aberdeen, UK (26); Bogotá, Colombia (22); Culdrose, UK (31); and Dublin, Ireland (22). This study is the first that shows a worldwide perspective of THILoad in dairy cows based on the model of St-Pierre et al. (2003). Therefore, dairies must rely on HS abatement systems to reduce the effects of HS on animal performance, welfare, and dairy farm profitability, from simple shades and barn air circulation systems to more complex CS. This represents an investment that, in times of economic uncertainty, dairy farmers need science-based tools to make decisions. The main objective of the present research was to develop a simulation modeling methodology based on 3 principles to help in the decision-making process of cooling cows: (1) straightforward to use, (2) replicable in different worldwide scenarios, and (3) adaptable to particular economic and technical conditions. The evaluation of the effects of HS on dairy farm technical and economic indicators is complex due to the multiple interactions among factors. For example, reduced fertility in summer results in seasonality of calving and milk yield (Washington et al., 2002; De Rensis and Scaramuzzi, 2003). In these conditions, the use of dynamic stochastic dairy herd simulations are excellent tools for modeling these effects (Calsamiglia et al., 2018). The first step to develop a profitability model is obtaining a quantifiable response to HS and CS. The St-Pierre et al. (2003) model, based on the THI index, has the advantage that it only requires temperature and relative humidity data that are frequently available from meteorological stations, compared with other indices such as the heat load index, which requires black globe temperature and wind speed that need to be measured on-site (Gaughan et al., 2008; Tresoldi et al., 2019). Schüller et al. (2013) compared data between the closest meteorological station and on-site farm measurements and found that farm measures were 11 THI units higher, and the THI index obtained using data from close meteorological stations may underestimate HS compared with the heat load index when measured on-farm. However, the opposite may also occur in other stations. In any case, the higher the precision of measurements, the better the value of results obtained with the model. In the model of St-Pierre et al. (2003), the THIthreshold was reduced from 72 to 70, and we further reduced it to 68 (Zimbelman et al., 2009). The trend for a lower THIthershold will continue over time as cows continue increasing their milk yield and metabolic heat production. The model of St-Pierre et al. (2003) contemplates the main HS effects that affect the economic performance in a dairy herd: changes in DMI, milk yield, fertility, and culling rates, with no consideration to changes in milk components. Although the milk fat and protein reduction during the summer has been traditionally associated directly with HS (Staples and Thatcher, 2011), the response of milk components to CS has been inconsistent (Tao et al., 2018). Salfer et al. (2019) suggested that the changes in milk fat and protein content throughout the year was the result of a physiological circadian cycle more than the direct effects of HS. In the present study, changes in fat and protein content throughout the year were accounted for (Supplemental Figures S1 and S2; https://doi.org/10 .6084/m9.figshare.21678932; Espinoza Sandoval, 2022) but not associated with HS or CS. In fact, the correlation between the model and the simulation output was very strong. Overall, the application of the St-Pierre et al. (2003) model was appropriate to be incorporated into our simulation. For example, Flamenbaum (2012) reported annual milk losses of up to 1,500 L/cow in Israel, consistent with the 1,695 L/cow per year in Tel Aviv in the present report. In contrast, some models of HS only focus on milk yield and fat and protein content, ignoring the reproductive and culling effects, and do not integrate the CS response (Bernabucci et al., 2014; Yano et al., 2014; Carabaño et al., 2016). Other models have been developed for a particular region and are difficult to replicate in other scenarios (Mukherjee et al., 2013). The present work evaluates the combination of fans and sprinklers as direct cooling in the feeding line, where more research has been conducted. This system is currently the most frequently used on farms worldwide due to its effectiveness and adaptation to multiple climate conditions. The system consumes from 4.5 to 25.7 L/h per cow (Van Os, 2019) and may become an issue in the Mediterranean, where the water exploitation index ranges from 20 to 80%. In fact, several areas located in the Middle East and North Africa are classified as severe desertification areas (Milano et al., 2013; Xevgenos et al., 2016). Therefore, consideration of water supply and the development of more water-efficient systems is necessary (Ortiz et al., 2015; Drwencke et al., 2020). Several approaches have been used to evaluate the profitability of CS, the majority under US conditions (St-Pierre et al., 2003; Ferreira et al., 2016). However, technical and economic conditions change among regions and countries, and even at the farm level. In the present study, the base technical and economic configuration of the simulated herds was based on the Mediterranean. For the 21 locations, 15 were inside the European Union, with small technical and economic differences among countries, and the remaining 6 were outside the European Union, where the interpretation should be more carefully considered. Overall, the profitability of investing in CS increased with the THILoad but was affected by the milk price and investment cost. For example, in Valencia, Spain, with a THILoad of 11,475, the effect of milk price was €17, and in Tel Aviv, Israel, with a THILoad of 31,424 was €54. This is due to the different b2 slopes of the regression lines (Table 5 and Figure 5). The model estimations in the 21 Mediterranean locations found that with a CS investment of €100, the CS was profitable in 70% of the locations, 21% with risk (average was positive, but values were not different from 0), and 10% not profitable. With an investment of €200, 49% of CS were profitable, 14% with risk (average was positive, but values were not different from 0), and 37% not profitable. For example, in Girona, Spain, the models suggest a negative-to-low profitability (−€7 to €14 / cow per year), so the investment in this location was risky.
Table6. Estimated effects of heat stress compared with the control scenario in selected locations in the Mediterranean1
1 Country codes according to the international standard norm (ISO-3166–1 α-2). THI = temperature and humidity index; THILoad is the integral of accumulated THI units above the THIthreshold of 68. IOFC = income over feed costs.
Table 7. Estimated effects of cooling systems compared with the heat stress in selected locations in the Mediterranean1
1 Country codes according to the international standard norm (ISO-3166–1 α-2). THI = temperature and humidity index; THILoad is the integral of accumulated THI units above the THIthreshold of 68. IOFC = income over feed costs.
Table 8. Change in the net margin (∆NM, €/cow per year) with the different model scenarios in selected locations of the Mediterranean at 2 cooling system investment costs (€100 vs. €200/cow) and 3 milk prices (€0.28, €0.32, and €0.36/L)1
1 Country codes according to the international standard norm (ISO-3166–1 α-2). THI = temperature and humidity index; THILoad is the integral of accumulated THI units above the THIthreshold of 68.
The presented methodology and models can help in the decision-making process of cooling, even though the optimal equilibrium between profitability and animal welfare needs to be considered. The incorporation of individual sensor-based data-acquisition systems such as body temperature, respiration rate, and rumination, among others, may be incorporated in future models to increase the accuracy of HS predictions and the efficiency of CS (Becker et al., 2021; Hut et al., 2022). The use of models that project how climate change will increase the intensity of HS in the coming decades will also contribute to evaluating the suitability of investing in CS (Dunn et al., 2014; Qi et al., 2015; Thornton et al., 2021).
CONCLUSIONS
This study determined and evaluated the effects of various intensities of HS in different locations in the Mediterranean. In most locations, HS resulted in reduced cattle performance and dairy farm profitability. Cooling systems partially revert the negative effects of HS, but their profitability will depend on the intensity of HS and its effects on performance, the milk price, the initial costs of investment in CS, and how they interact.
Simulation techniques that model the whole-herd system can achieve better evaluations than traditional techniques due to their ability to contemplate more interactions at the herd level.
This article was originally published in Journal of Dairy Science. 106:5485–5500 https://doi.org/10.3168/jds.2022-22816. This is an Open Access article under the CC BY license (http://creativecommons.org/licenses/by/4.0/).