Milk quality is considered a welfare issue. Most dairy cooperatives have established quality-based payment programs to provide incentives to improve milk quality (Botaro et al. 2013). Total bacterial count, somatic cell count (SCC), and milk lipid and protein contents are some measurements that either result in the application of a penalty or in the receipt of a premium (Renau 2001). These parameters are also utilised not only in the milk industry, but also in dairy research, and are targets of managerial, nutritional, and health protocols.
SCC is a diagnostic parameter for udder health. The quarters with SCC > 200 000/ml are considered to be infected (Schukken et al. 2003). However, SCC is a variable parameter and is influenced by a number of factors (i.e., udder infection and inflammation, milking interval, milk yield, lactation stage, parity, drug administration, and season) (Kehrli and Schuster 1994; Nielsen et al. 2005). Additionally, SCC can also vary from day to day and from milking to milking in the same quarters (Quist et al. 2008).
Fatty acids, which are essential for milk fat synthesis, originate from de novo synthesis in alveolar epithelial cells, and can also be delivered through the diet, and mobilised from body reserves. Milk lipids consist of triacylglycerol (TAG-CE) (95%), diacylglycerol (DAG) (2%), phospholipid (PL), cholesterol (CHOL) (1%), and free fatty acid (FFA) (0.01%) (Jensen 1995; Ma 2012).
Numerous proteins make up protein content of milk. Casein is the primary protein and exists in α, β, γ, and κ fractions. Non-casein milk proteins are classified as whey proteins. The major whey proteins are α-lactalbumin (α-LA) and β-lactoglobulin (β-LG), which are synthesised only in alveolar epithelial cells. Whey proteins play roles in several biological processes such as lactose synthesis, prevention of cancer, treatment of chronic stress-induced disease, transfer of passive immunity, and regulation of mammary gland phosphorus metabolism. Additionally, immunoglobulin (Ig) and serum albumin, which originate from theplasma, contribute to anti-tumour and antioxidant activities, and are involved in the immune response via colostrum (Maas et al. 1997; Madureira et al. 2007).
Milk proteins and fatty acids are influenced by many factors, such as age (Ng-Kwai-Hang et al. 1987), seasonal changes (Heck et al. 2009), diet (Jahreis et al. 1996), genetic variations (Gaunt 1973), intramammary infections (Auldist et al. 1995), lactation stage (Ng-Kwai-Hang et al. 1987), milking frequency (Shields et al. 2011), and milk yield (Mantere-Alhonen 1995). Significant interactions are also described between milk SCC, lipids and proteins (Ng-Kwai-Hang et al. 1987), suggesting that global assessment of milk composition may be needed for evaluation at animal and herd level. In order to carry out such an analysis, however, the factors that cause variability should first be identified. The aim of this study was to evaluate variability in milk SCC and lipid and protein profiles with respect to breed, parity, days in milk, and sampling time.
Material and methods
Herd and cows. This study was carried out on Holstein (n = 5) and Brown Swiss cows (n = 6), which were housed on the Ataturk University Research Farm and fed the same ration [80% forage (80% corn silage, 10% alfalfa hay, and 10% wheat straw) and 20% compound feeds (18–19% crude protein and 2700 ME kcal/kg)]. All cows were free from mastitis and in the early lactation period (34 ± 6 DIM, 14 ± 2 kg milk); the animals varied by parity (parity 1, n = 3; parity 2, n = 2; parity 3, n = 3; parity 4, n = 3).
Bacteriological procedure. The health status of quarters was evaluated using bacteriological analysis (Harmon et al. 1990). Briefly, 10 ml of individual milk samples were collected aseptically into sterile plastic tubes and transported to the laboratory in iceboxes within 15 min. Ten μl of milk samples were cultured on blood and MacConkey agar and incubated at 37 °C for 24–48 h. This analysis revealed the absence of any intramammary infections.
Sampling procedure. Milk samples of equal volumen (10 ml) were collected from four quarters into 40 ml plastic tubes (LP Italiana SPA, Milan, Italy). Sampling was initiated just before A.M. milking (06:00 h) and was carried out until P.M. milking (18:00 h) every 2 h. Sampling was repeated on three consecutive days.
Analysis of milk components:
Somatic cell count. The SCC was determined by an automated fluorescent microscopic somatic cell counter (Somacount150®, Bentley Instrument, USA). Ethidium bromide dye was used to detect cellular DNA. The SCC results were transformed to log10 base to obtain homogeneity.
Lipids. Milk lipid profiles were analysed by high performance thin layer chromatography (HPTLC). Five hundred μl of n-hexane : iso-propanol 3 : 2 (v/v) mixture were added to 1000 μl of milk. After vigorous vortexing, the tubes were centrifuged at 5000 g for 5 min at 40 °C, and the upper phase were used for HPTLC analysis (Hara and Radin 1978).
The electrophoresis was carried out in tris-glycine running buffer pH 8.3 (Trizma base 1.515 g, glycine 7.2 g, SDS 0.25 g/500 ml) at 20 mA/gel constant current for 90 min and proteins were visualised by coomassie blue R250 staining.
Evaluation of chromatograms and electrophoretograms. HPTL chromatograms and SDS-PAGE electrophoretograms were scanned with an Epson Perfection® V500 Photo scanner at 600 DPI resolution as 16-bit grayscale TIFF images and analysed with TL 120 software (Kaynar et al. 2013). Results were expressed as the percentages of fractions.
Data analysis. Data were subjected to 4-way ANOVA using the GLM Procedure (SAS 2002). The model included the main effects of breed, parity, sampling day, and sampling time as well as their 2-, 3- and 4-way interactions. Non-significant terms were subjected to backward elimination from the linear model. Differences among subcategories were compared using the LSD option. Differences were considered to be statistically significant at P < 0.05.
Table 2. Effects of variables on milk lipid profiles.
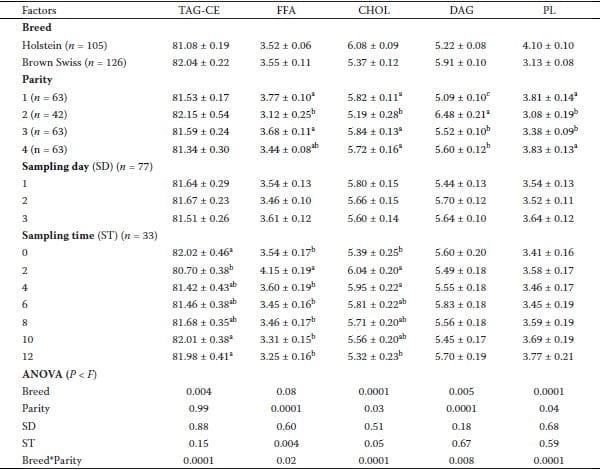
The experiment started in 34 ± 6 DIM and A.M. milking was at 06:00 h. TAG-CE = triacylglycerol, FFA = free fatty acid, CHOL = cholesterol, DAG = diacylglycerol, PL = phospholipid.
a,b,c,dsuperscripts represent statistical differences among the factor categories.
Table 3. Effects of variables on milk protein profiles.
The experiment started in 34 ± 6 DIM and A.M. milking was at 06:00 h. NS = not significant .
a,b,c,d Superscripts represent statistical differences among the factor categories.
Results
Unless mentioned below there was no main effect of fixed factors and their interactions. The effects of proposed factors causing variation in SCC (Table 1), lipid (Table 2), and protein (Table 3) profiles are presented.
Somatic cell count
SCC increased as parity advanced (P < 0.0005) and SCC decreased at later sampling times relative to A.M. milking (P < 0.0001).
Lipid profile
Brown Swiss had higher TAG-CE (P < 0.004) and DAG (P < 0.005) and lower CHOL (P < 0.0001) and PL (P < 0.0001) fractions than Holstein. As parity advanced, the FFA (P < 0.0001), CHOL (P < 0.03), DAG (P < 0.0001), and PL (P < 0.04) fractions fluctuated.
Sampling day did not cause variation in lipid fractions. The FFA (P < 0.004) and CHOL (P < 0.05) fractions varied by the sampling time, whereas the TAG-CE, DAG, and PL fractions did not exhibit diurnal variation.
There was a breed . parity interaction effect on lipid profile (P < 0.02 to P < 0.0001). There were parabolic increases in TAG-CE, FFA, and DAG percentages in Holstein, whereas there were parabolic decreases in CHOL and PL percentages in Brown Swiss, as the parity advanced (Figure 1).
Figure 1. Effect of breed by parity interaction on milk lipid profiles.
Protein profile
About 24% of milk proteins were identified in SDSPAG electrophoretograms. Except for the lactoferrin fraction, there was a breed effect on protein profile. Brown Swiss cows had greater fractions of IgG and IgA, albumin, casein (α, β, and κ), α-LA, and β-LG in milk than Holstein cows (P < 0.0005 to P < 0.0001).
As parity advanced, protein fractions changed in different fashions (P < 0.02 to P < 0.0001). There was linear decrease in the IgG fraction, parabolic decreases in the albumin and IgA fractions after the 2nd lactation, parabolic increases in the lactoferrin, α-casein, α-LA, and β-LG fractions after the 2nd lactation, a cubic change in the κ-casein fraction and an exponential decrease in the β-casein fraction after the 1st lactation.
Milk protein profile varied on consecutive sampling days (P < 0.04 to P < 0.0001). The lactoferrin, α-casein, β-casein, and β-LG fractions increased quadratically, the albumin and α-LA fractions increased linearly, the IgA fraction decreased quadratically, and the κ-casein fraction decreased linearly over the course of sampling over three consecutive days.
Among milk protein fractions, only the IgG fraction exhibited diurnal variation (P < 0.0001). There was a cubic pattern in the IgG fraction after A.M. milking.
A significant breed × parity interaction effect was observed for protein profile (P < 0.001 to 0.0001; Figure 2). Changes in milk protein fractions with advancing parity for Holstein were more notable than for Brown Swiss.
Figure 2. Effect of breed by parity interaction on milk protein profiles.
Except for the α and β-casein fractions, other milk proteins changed over consecutive sampling days differently in each breed (P < 0.09 to P < 0.0001; Figure 3). The IgG and albumin fractions increased at a higher rate in Holstein than Brown Swiss towards the last day of sampling. The lactoferrin and α-LA fractions decreased quadratically in Holstein and increased quadratically in Brown Swiss over sampling days. The κ-casein fraction decreased quadratically in Holstein and decreased linearly in Brown Swiss. The IgA fraction decreased quadratically and the β-LG fraction increased quadratically in both breeds, at a lesser extent in Holstein tan in Brown Swiss.
Figure 3. Effect of breed by sampling day interaction on milk protein profiles. The experiment started in 34 ± 6 DIM.
Diurnal variations in milk protein fractions differed between Holstein and Brown Swiss, except for the albumin fraction (P < 0.02 to P < 0.0001; Figure 4).
Figure 4. Effect of parity by sampling day interaction on milk protein profiles. The experiment started in 34 ± 6 DIM.
Discussion
Somatic cell count
Breed, parity, sampling time, stage, and lactation number do influence test day milk yield, SCC, lipid and protein content in cows (Schutz et al. 1990), sheep (Gonzalo et al. 1994), and goats (Boscos et al. 1996). Milk components are important variables for research and applied herd management.
SCC is an important marker in evaluation of udder health (Renau 2001; Schukken et al. 2003). Many factors such as infection status, age, stage of lactation, season, stress, technical aspects, diurnal and day-to-day variations affect SCC (Dohoo and Meek 1982; Sheldrake et al. 1983; Leavens et al. 1997). In agreement with the literature (Dohoo and Meek 1982; Schutz et al. 1990; Harmon 1994), SCC increased with parity in the present study, which could be related to increased milk production (Table 1). SCC increases with advanced parity in non-infected quarters, which may be associated with a higher prevalence of permanent glandular damage from resolved infections in older cows. Some researchers reported little change in cell concentrations in non-infected quarters with advancing age (Sheldrake et al. 1983; Laevens et al. 1997), whereas others have observed a decrease in SCC in the first part of lactation (Schepers et al. 1997). Diurnal fluctuation and sampling time variation were reported as SCC-influencing factors (Harmon 1994).
Diurnal variation (Table 1) suggests that sample collection time relative to A.M. milking should be consistent for accuracy of comparison in evaluation of animals and data. In agreement with other studies (Dohoo and Meek 1982; Harmon 1994), which suggested a decrease before milking, SCC was lowest immediately before A.M. and P.M. milking. Clearly, this was a dilution effect. Day to day variation in SCC was also reported in healthy quarters, which was attributed to possible elimination of microorganisms before sampling or stress and trauma (Dohoo and Meek 1982). However, in the current study a day-today variation was not observed (Table 1).
Milk lipids
In addition to dietary factors (Palmquist et al. 1993), which were eliminated in this study by administering the same feeding program, breed and parity were the most influential factors on milk lipid profile (Table 2, Figure 1) as has also been found in previous studies (De Peters et al. 1995; Kelsey et al. 2003; Soyeurt et al. 2006). In this study, Holstein had higher CHOL, and PL percentages, whereas Brown Swiss had higher TAG-CE and DAG percentages.
In contrast to previous studies (Svennersten-Sjaunja et al. 2005; Forsback et al. 2010), day-to-day variations were an insignificant factor for milk lipid content. Changes in the FFA and CHOL fractions within the same day (Table 2) could be related to blood flow and the rate of milk synthesis (Kaartinen 1995). A parabolic increase and then a gradual decrease in the FFA fraction together with a stable CHOL fraction could indicate immediate utilisation for body reserves.
The TAG-CE, FFA, and DAG fractions decreased quadratically in Holstein, whereas the CHOL and PL fractions decreased quadratically in Brown Swiss, as the parity advanced (Figure 1). This phenomenon could indicate differences in the respective needs of these breeds to conserve these nutrients to ensure adequate milk composition for calves.
Milk proteins
Milk proteins were affected by all proposed factors (Table 3, Figures 2, 3, and 4). It is clear that Brown Swiss had considerably higher protein fractions than Holstein cows; this finding reflects their genetics (Cerbulis and Farrell 1975). Overall, the breed by parity, and breed by sampling day interactions appear to be driven by aging, suggesting competition between udder and maternal reserves (Ng-Kwai-Hang et al. 1984, 1987).
The day-to-day variation in protein profiles is minimal (Svennersten-Sjaunja et al. 2005; Forsback et al. 2010). Although the overall amount did not vary greatly, the composition of milk protein fractions exhibited considerable variation between sampling days (Table 3). Although diurnal variation in milk protein fractions was reported in previous studies (Gilbert et al. 1973; Quist et al. 2008), only IgG varied within the day (Table 3).
Mammary gland absorbs most of the components from the blood stream and then combines them with structural enzymes to synthesise milk. The effects of breed, parity, sampling day, and sampling hour as well as their interactions on milk components were here evaluated in cows. In conclusion, (1) SCC was considerably influenced by parity and sampling hour. (2) Parity, breed, and sampling day exerted an influence on the composition of milk lipid and protein fractions. (3) There were twoway interaction effects of factors on SCC, but not on milk lipid and protein fractions. These factors and their interactions should be considered in the planning of research and in the evaluation of data.
This article was originally published in Veterinarni Medicina, 60, 2015 (1): 6–15 doi: 10.17221/7920-VETMED.
References
1. Auldist MJ, Coats S, Rogers GL, McDowell GH (1995): Changes in the composition of milk from healthy and mastitic dairy cows during the lactation cycle. Animal Production Science 35, 427–436.
2. Boscos C, Stefanakis A, Alexopoulos C, Samartzi F (1996): Prevalence of subclinical mastitis and influence of breed, parity, stage of lactation and mammary bacteriological status on Coulter Counter Counts and California Mastitis Test in the milk of Saanen and autochthonous Greek goats. Small Ruminant Research 21, 139–147.
3. Botaro BG, Gameiro AH, dos Santos MV (2013): Quality based payment program and milk quality in dairy cooperatives of Southern Brazil: an econometric analysis. Scientia Agricola 70, 21–26.
4. Cerbulis J, Farrell HM (1975): Composition of milks of dairy cattle. I. Protein, lactose, and fat contents and distribution of protein fraction. Journal of Dairy Science 58, 817– 827.
5. De Peters EJ, Medrano JF, Reed BA (1995): Fatty acid composition of milk fat from three breeds of dairy cattle. Canadian Journal of Animal Science 75, 267–269.
6. Dohoo IR, Meek AH (1982): Somatic cell counts in bovine milk. Canadian Veterinary Journal 23, 119–125.
7. Forsback L, Lindmark-Mansson H, Andren A, Akerstedt M, Andree L, Svennersten- Sjaunja K (2010): Day-to-day variation in milk yield and milk composition at the udderquarter level. Journal of Dairy Science 93, 3569–3577.
8. Gaunt NS (1973): Genetic and environmental changes posible in milk composition. Journal of Dairy Science 56, 270–278.
9. Gilbert GR, Hargrove GL, Kroger M (1973): Diurnal variations in milk yield, fat yield, milk fat percentage, and milk protein percentage of Holstein-Friesian cows. Journal of Dairy Science 56, 409–410.
10. Gonzalo C, Carriedo JA, Baro JA, San Primitivo F (1994): Factors influencing variation of test day milk yield, somatic cell count, fat, and protein in dairy sheep. Journal of Dairy Science 77, 1537–1542.
11. Hara A, Radin NS (1978): Lipid extraction of tissues with a low-toxicity solvent. Analytical Biochemistry 90, 420–426.
12. Harmon RJ (1994): Physiology of mastitis and factors affecting somatic cell counts. Journal of Dairy Science 77, 2103–2112.
13. Harmon RJ, Eberhart RJ, Jasper DE, Langlois BE, Wilson RA (1990): Microbiological procedures for the diagnosis of bovine udder infection. In: Proceedings of National Mastitis Council, Arlington (VA), USA, 4–34.
14. Heck JML, van Valenberg HJF, Dijkstra J, van Hooijdonk ACM (2009): Seasonal variation in the Dutch bovine raw milk composition. Journal of Dairy Science 92, 4745– 4755.
15. Jahreis G, Fritsch J, Steinhart H (1996): Monthly variations of milk composition with special regard to fatty acids depending on season and farm management systemsconventional versus ecological. European Journal of Lipid Science and Technology 98, 356–359.
16. Jensen RG (1995): Handbook of Milk composition. Access Online via Elsevier.
17. Kaartinen L (1995): Physiology of the bovine udder. In: Sandholm M, Honkanen-Buzalski T, Kaartinen L, Pyorala S (eds.): The Bovine Udder and Mastitis. 1st ed. University of Helsinki, Faculty of Veterinary Medicine. 14–23.
18. Kaynar O, Ileriturk M, Hayirli A (2013) Evaluation of Computational modifications in HTLC with gel analysis software and flatbed scanner for lipid separation. Journal of Planar Chromatography-Modern TLC 26, 202–208.
19. Kehrli ME, Shuster DE (1994): Factors affecting milk somatic cells and their role in health of the bovine mammary gland. Journal of Dairy Science 77, 619–627.
20. Kelsey JA, Corl BA, Collier RJ, Bauman DE (2003) The effect of breed, parity, and stage of lactation on conjugated linoleic acid (CLA) in milk fat from dairy cows. Journal of Dairy Science 86, 2588–2597.
21. Laemmli UK (1970): Cleavage of structural proteins during the assembly of the head of bacteriophage T4. Nature 227, 680–885.
22. Laevens H, Deluyker H, Schukken YH, De Meulemeester L, Vandermeersch R, De Muelenaere E, De Kruif A (1997): Influence of parity and stage of lactation on the somatic cell count in bacteriologically negative dairy cows. Journal of Dairy Science 80, 3219–3226.
23. Ma L (2012): Regulatory factors of milk fat synthesis in dairy cows. [PhD Dissertation.] Virginia Polytechnic Institute and State University, Blacksburg, VA, USA.
24. Maas JA, France J, McBride BW (1997): Model of milk protein synthesis a mechanistic model of milk protein synthesis in the lactating bovine mammary gland. Journal of Theoretical Biology 187, 363–378.
25. Madureira AR, Pereira CI, Gomes AMP, Pintado ME, Malcata FX (2007): Bovine whey proteins – Overview on their main biological properties. Food Research International 40, 1197–1211.
26. Mantere-Alhonen S (1995): Composition of milk. In: Sandholm M, Honkanen-Buzalski T, Kaartinen L, Pyorala S (eds.): The Bovine Udder and Mastitis. 1st ed. University of Helsinki, Faculty of Veterinary Medicine. 24–30.
27. Ng-Kwai-Hang KF, Hayes JF, Moxley JE, Monardes HG (1984): Variability in test-day milk production and composition and relation of somatic cell counts with yield and compositional changes of bovine milk. Journal of Dairy Science 67, 361–366.
28. Ng-Kwai-Hang K, Hayes JF, Moxley JE, Monardes HG (1987): Variation in milk protein concentrations associated with genetic polymorphism and environmental factors. Journal of Dairy Science 70, 563–570.
29. Nielsen NI, Larsen T, Bjerring M, Ingvartsen KL (2005): Quarter health, milking interval, and sampling time during milking affect the concentration of milk constituents. Journal of Dairy Science 88, 3186–3200.
30. Palmquist DL, Denise Beaulieu A, Barbano DM (1993): Feed and animal factors influencing milk fat composition. Journal of Dairy Science 76, 1753–1771.
31. Quist MA, LeBlanc SJ, Hand KJ, Lazenby D, Miglior F, Kelton DF (2008): Milking-to-milking variability for milk yield, fat and protein percentage, and somatic cell count. Journal of Dairy Science 91, 3412–3423.
32. Renau JK (2001): Somatic cell counts: Measures of farm management and quality. In: Proceeding in Annual Meeting of National Mastitis Council, Nevada, USA, 29–37.
33. SAS (2002): User’s Guide. Statistics, Version 9. Statistical Analysis System. SAS Inst., Inc., Cary, NC, USA.
34. Schepers AJ, Lam TJGM, Schukken YH, Wilmink JBM, Hanekamp WJA (1997): Estimation of variance components for somatic cell counts to determine thresholds for uninfected quarters. Journal of Dairy Science 80, 1833–1840.
35. Schukken YH, Wilson DJ, Welcome F, Garrison-Tikofsky L, Gonzalez RN (2003): Monitoring udder health and milk quality using somatic cell counts. Veterinary Research 34, 579–596.
36. Schutz MM, Hansen LB, Steuernagel GR, Kuck AL (1990): Variation of milk, fat, protein, and somatic cells for dairy cattle. Journal of Dairy Science 73, 484–493.
37. Sheldrake RF, Hoare RJT, McGregor GD (1983): Lactation stage, parity, and infection affecting somatic cells, electrical conductivity, and serum albumin in milk. Journal of Dairy Science 66, 542–547.
38. Shields SL, Rezamand P, Sevier DL, Seo KS, Price W, McGuire MA (2011): Effects of increased milking frequency for the first 21 days post partum on selected measures of mammary gland health, milk yield and milk composition. Journal of Dairy Research 78, 301–307.
39. Soyeurt H, Dardenne P, Gillon A, Croquet C, Vanderick S, Mayeres P, Bertozzi C, Gengler N (2006), Variation in fatty acid contents of milk and milk fat within and across breeds. Journal of Dairy Science 89, 4858–4865.
40. Svennersten-Sjaunja K, Larsson U, Bertilsson J, Sjaunja LO (2005): The relative day-to-day variation in milk yield and composition for cows milked two or three times daily. In: Annual Meeting of the European Association for Animal Production, Uppsala, Sweden, 5–8 June, 382.